Jump to a key chapter
Media Content Analysis Definition
Media Content Analysis is a method used to systematically examine communications in media. By understanding this process, you can dissect content to uncover patterns, themes, and meanings behind the information presented in various media forms.
Media Content Analysis refers to the systematic examination and interpretation of media messages to identify specific themes, symbols, or meanings. This analysis helps in understanding underlying patterns or trends in various types of media communications.
Importance of Media Content Analysis
Media Content Analysis is important for several reasons:
- Understanding Influence: Determines how media content influences audiences.
- Content Trends: Identifies trends and patterns in media messaging over time.
- Audience Insights: Helps you understand how different audiences perceive media messages.
- Message Impact: Evaluates the effectiveness of media messages in reaching target audiences.
For instance, you could use Media Content Analysis to study how different news outlets cover a political event, noting the differences in language, tone, and imagery.
Steps in Conducting Media Content Analysis
To conduct Media Content Analysis, you typically follow these steps:
- Define Your Research Question: Clearly state what you intend to find out.
- Select Your Sample: Choose the media content you will analyze, such as articles, videos, or social media posts.
- Determine Your Categories: Decide on the themes or elements you are looking to identify within the content.
- Analyze the Content: Examine the content according to your categories and research question.
- Interpret the Results: Draw conclusions based on your analysis and insights.
Always ensure that your categories are clear and specific to improve the reliability of your media content analysis results.
Media Content Analysis Explained
Understanding Media Content Analysis is crucial as it helps dissect and interpret the information disseminated across various media types. By analyzing content systematically, you can discover patterns, trends, and meanings that might not be immediately apparent. This process is essential for media studies and communication fields.
Media Content Analysis is a research technique for making replicable and valid inferences by categorizing textual, visual, or verbal media data into predetermined categories.
Importance of Media Content Analysis
Media Content Analysis plays a significant role in understanding how media influences society and individual perspectives. It aids in:
- Revealing Biases: Helps uncover biases and assumptions in media content.
- Understanding Representation: Analyzes how different groups are represented or misrepresented.
- Evaluating Media Effects: Assesses how media content can affect audience behavior and beliefs.
Consider analyzing the portrayal of climate change across different media outlets. This analysis might reveal which narratives are most prevalent and how they shape public perception.
Steps in Conducting Media Content Analysis
The process of conducting Media Content Analysis includes several systematic steps:
- Identify the Objective: Start with a clear goal or research question to guide your analysis.
- Select the Media: Choose samples such as articles, broadcasts, or social media posts that relate to your objective.
- Develop a Coding System: Create categories to classify the content. These should be clear and relevant to your research question.
- Process the Data: Examine and categorize the media content according to your coding system.
- Interpretation: Analyze the findings to draw conclusions that answer your research question.
While analyzing media content, it's important to consider the context and subtext of the messages being communicated. Media often reflects the socio-political environment of the time, and its influence can reach far beyond immediate impressions. Researchers should be mindful of the latent content, which includes the implications and hidden meanings that are not explicitly stated.
In Media Content Analysis, be aware of the evolving nature of media platforms and adjust your methods to include digital media's nuances.
Techniques for Media Content Analysis
To effectively study media communications, a variety of techniques are employed. These techniques can be broadly categorized as qualitative and quantitative, each offering unique insights into media content.
Qualitative Techniques in Media Content Analysis
Qualitative Techniques focus on exploring the deeper meanings and interpretations of media content. These techniques are subjective and often involve detailed descriptions and analyses. Common methods include:
- Thematic Analysis: Identifies recurring themes or messages in media content. This involves reading through the text or media form multiple times to find patterns.
- Discourse Analysis: Examines how language is used to construct meaning and convey power in media content. It explores the influence of context and culture on communication.
- Narrative Analysis: Analyzes the stories told in media content and how these narratives shape audience understanding and perception.
In qualitative analysis, always consider the cultural and social context in which the media content was created.
Qualitative media content analysis often uses computer-assisted qualitative data analysis software (CAQDAS) to organize and interpret large amounts of media data. This software can help identify patterns and themes that may not be obvious during manual analysis. It is invaluable for handling vast digital archives and streaming content.An example can be seen in analyzing political advertisements where the focus might be on the language used to convey particular ideologies or the imagery that suggests affiliative cues.To study these, consider the overlapping patterns or shifts in narrative style across different election cycles or media platforms. This provides insights into the evolution of political discourse and its impact on public opinion.
Quantitative Techniques in Media Content Analysis
Quantitative Techniques provide a more objective approach to media content analysis by using numerical data and statistical methods. These techniques include:
- Content Coding: Transforms media content into numerical data by categorizing elements such as frequency of key terms, presence of certain images, or climate change mentions. Each item is assigned a value for further analysis.
- Statistical Analysis: Employs statistical tools to analyze the coded data. This might include calculating the mean, median, or correlations.
- Trend Analysis: Investigates how certain media content elements change over time, such as the shift in tone regarding environmental issues. A common formula used in trend analysis could be the linear regression model, represented as: \ y = mx + c \ where y is the theme measure, m is the trend slope, x is the time variable, and c is the intercept.
Imagine you're analyzing the frequency of social media posts mentioning renewable energy over a year. You might use numerical data to map out the number of mentions per month and then apply statistical methods to identify any significant trends or peaks in this content.
While analyzing quantitative data, visual aids such as charts and graphs can greatly enhance comprehension and presentation.
The use of machine learning algorithms is advancing in quantitative media content analysis. These algorithms can automatically categorize and code large datasets, handling tasks that are too challenging to perform manually. For example, through Natural Language Processing (NLP), computers can now identify sentiment, categorize subjects, and detect anomalies in text-based media. This capability accelerates the analysis process and broadens the scope of data that can be examined, allowing researchers to uncover trends and insights at an unprecedented scale.
Media Content Analysis Examples
Studying examples of Media Content Analysis can greatly enhance your understanding of how it works in practice. These examples illustrate the application of techniques and methods to real-world media content.
Analyzing News Coverage
When analyzing news coverage, Media Content Analysis helps identify patterns in how different media outlets report on events. This might focus on aspects like:
- Bias Detection: Spotting discrepancies in the language or framing used by different outlets.
- Recurring Themes: Recognizing frequent subject areas, such as public safety or political bias.
- Source Quotation: Evaluating which experts or officials are quoted and how often.
Consider the coverage of a major environmental disaster. By analyzing how different media sources report on the event, you might note which outlets emphasize the environmental impact versus the economic implications.
Social Media Campaigns
Media Content Analysis is also used to assess the effectiveness of social media campaigns. By analyzing these campaigns, you can examine:
- Engagement Levels: Quantifying likes, shares, and comments to gauge audience interest.
- Sentiment Analysis: Determining the overall positive, negative, or neutral sentiment expressed in comments.
- Message Consistency: Ensuring the core message remains consistent across different platforms.
Tracking campaign hashtags can provide insights into public engagement and theme prevalence over time.
For social media campaigns, advanced analytics software may track interactions and visual data over time, offering trends that manual analysis would miss. Software capable of handling big data can store and process massive datasets, unlocking patterns in audience interactions not possible through traditional methods. This leads to strategic insights, such as the timing of posts for optimal engagement and which visual content attracts more attention. Employing machine learning algorithms helps predict future trends based on historical data, giving marketers and researchers an edge in campaign planning and execution.
Content Analysis of Advertising
In advertising, Media Content Analysis helps businesses understand how their ads communicate messages and affect consumer behavior. This involves examining:
- Visual Symbolism: Decoding recurrent symbols or themes in advertising images.
- Language Use: Analyzing the choice of words and their connotations to appeal to the target audience.
- Audience Reaction: Measuring the response to ads through surveys or social media feedback.
For instance, a study of food advertisements might reveal a tendency to associate certain food products with happiness or health, influencing consumer perception and purchase behavior.
Ad campaigns often utilize emotional appeals; understanding these can predict consumer reaction.
Content Analysis in Media Research
Content Analysis is a foundational method in media research, offering valuable insights into how media communicates with its audience. By systematically examining media messages, you can explore underlying patterns, trends, and symbols. This technique provides a lens through which you can assess the impact and effectiveness of media communications.
Role in Media Studies Methodologies
Within media studies, Content Analysis serves several critical purposes. It allows researchers to:
- Investigate Representation: Examines how certain groups or issues are portrayed within media content, informing debates on representation and diversity.
- Evaluate Media Influence: Analyzes how media shapes public opinion by identifying trends in narrative or language.
- Track Changes Over Time: Documents shifts in media focus or tone by comparing historical data with current content.
In some instances, Content Analysis is utilized to explore the socio-political context reflected in media messages. For example, political speeches in media can be analyzed for thematic consistency or changes in rhetoric during election campaigns. Content Analysis often employs software to sift through large datasets, facilitating pattern recognition and enabling researchers to make data-driven arguments about media practice and strategy.
Media researchers often use cross-disciplinary approaches to enrich their analysis, incorporating perspectives from sociology, psychology, and cultural studies.
Applications in Media Studies Research
Content Analysis is applied across diverse research fields within media studies. Some key applications include:
- Advertising Analysis: Investigates the effectiveness and appeal of advertising messages and their impact on consumer behavior.
- News Analysis: Studies bias or selectivity in news reporting, shedding light on journalistic practices.
- Social Media Monitoring: Tracks the flow and reach of ideas in digital communication, offering insights into viral trends and public discourse.
An example of Content Analysis can be seen in the study of political news coverage. By analyzing various media outlets, you might discover discrepancies in the portrayal of political candidates, highlighting potential biases or ethical considerations in journalism.
Consider utilizing open-access media databases to expand your research sources and uncover broader trends.
Content Analysis in media studies frequently leverages technological advances like AI and machine learning. These technologies can automate the analysis of large datasets, detecting sentiment or thematic shifts faster and with more accuracy than traditional methods. This computational approach offers a more comprehensive view of media impact and audience reception, facilitating real-time adjustments to media strategies in industries such as marketing and political campaigning.
Media Content Analysis - Key takeaways
- Media Content Analysis Definition: A systematic method for examining media communications to identify themes, patterns, and meanings.
- Importance: It uncovers biases, trends, audience perceptions, and the impact of media messages.
- Techniques: Includes qualitative (e.g., thematic, discourse, and narrative analysis) and quantitative methods (e.g., content coding, statistical analysis).
- Application Steps: Define research question, select media sample, develop categories, analyze content, and interpret results.
- Examples: Analyzing news coverage, social media campaigns, and advertising for bias, engagement, and message effectiveness.
- Role in Media Studies: Essential for evaluating media influence, representation, and tracking changes over time within media research methodologies.
Learn faster with the 10 flashcards about Media Content Analysis
Sign up for free to gain access to all our flashcards.
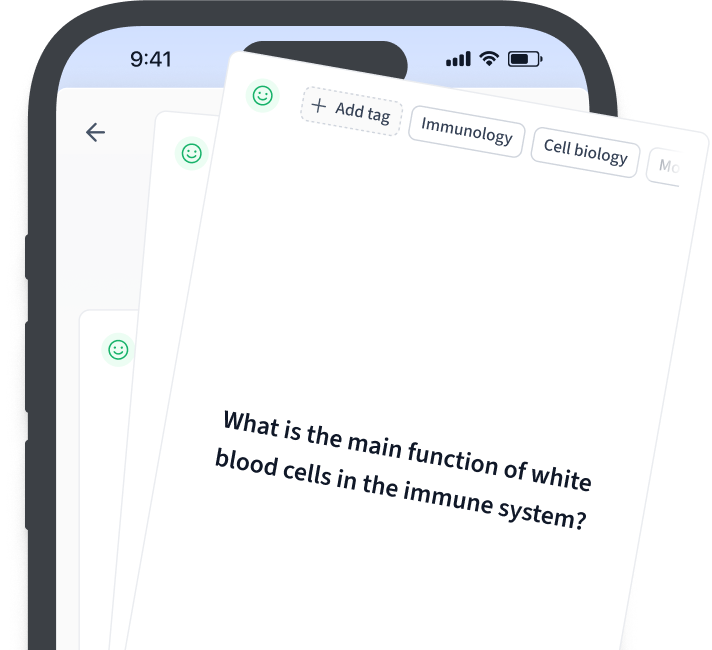
Frequently Asked Questions about Media Content Analysis
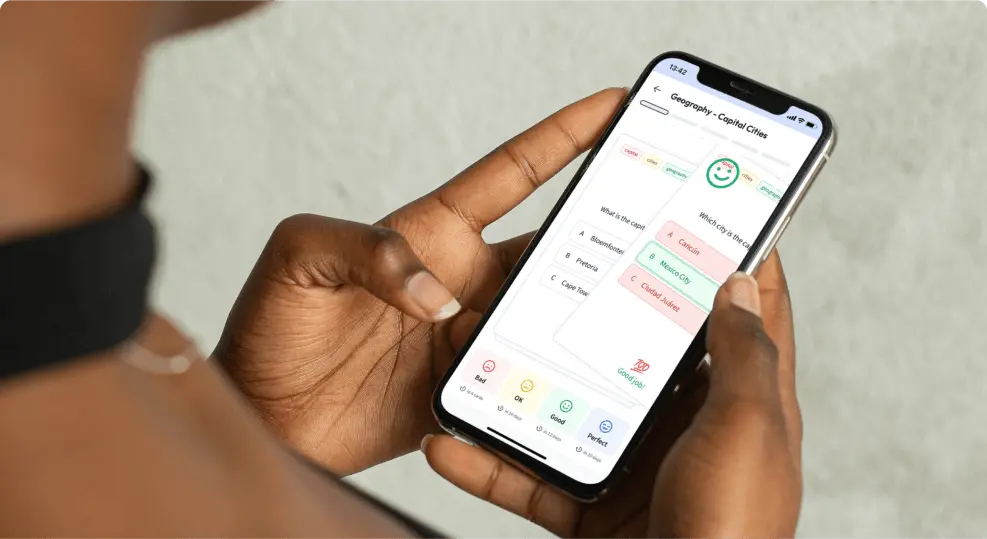
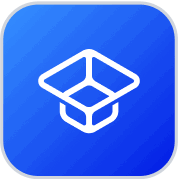
About StudySmarter
StudySmarter is a globally recognized educational technology company, offering a holistic learning platform designed for students of all ages and educational levels. Our platform provides learning support for a wide range of subjects, including STEM, Social Sciences, and Languages and also helps students to successfully master various tests and exams worldwide, such as GCSE, A Level, SAT, ACT, Abitur, and more. We offer an extensive library of learning materials, including interactive flashcards, comprehensive textbook solutions, and detailed explanations. The cutting-edge technology and tools we provide help students create their own learning materials. StudySmarter’s content is not only expert-verified but also regularly updated to ensure accuracy and relevance.
Learn more