Jump to a key chapter
What is Synaptic Integration
Synaptic integration is a crucial concept in neuroscience that explains how neurons in the brain and nervous system process and integrate incoming signals to generate an appropriate response. This integration of synaptic inputs influences the activity of neurons and ultimately contributes to the functioning of the nervous system. Understanding synaptic integration is vital for exploring how the brain processes information.
The Process of Synaptic Integration
Synaptic integration involves the combination of multiple synaptic inputs to generate a unified response in the postsynaptic neuron. This complex process requires several key steps:
- Resting Membrane Potential: Neurons have a resting potential due to the distribution of ions across their membranes. This potential sets the stage for synaptic signals.
- Synaptic Inputs: Neurons receive signals from other neurons via synapses, which can be excitatory or inhibitory. These signals lead to changes in the membrane potential.
- Spatial Summation: When postsynaptic potentials occur at different locations on the neuron, the neuron processes these inputs near simultaneously.
- Temporal Summation: When multiple synaptic potentials occur at the same location in quick succession, they can build upon each other to affect the neuron.
- Action Potential Generation: If the integrated synaptic inputs result in a sufficiently large change in the membrane potential, an action potential is triggered, allowing the neuron to pass the signal along.
Synaptic integration is the process by which multiple synaptic inputs combine within a neuron, determining whether or not the neuron will fire an action potential.
Consider a neuron receiving excitatory inputs from several sensory neurons and inhibitory inputs from an interneuron. The excitatory inputs depolarize the neuron, while the inhibitory input acts to hyperpolarize the neuron at the same time. If the excitatory effects are stronger when summed up (spatial and temporal summation) than the inhibitory ones, the postsynaptic neuron might reach the threshold needed to fire an action potential.
Not all signals received by a neuron lead to an action potential. Only when the combined effect of synaptic inputs crosses a certain threshold does the neuron respond.
What is Synaptic Integration
Synaptic integration is a fundamental concept in neuroscience that describes how neurons in the brain and nervous system process and integrate incoming signals to generate an appropriate response. The integration of synaptic inputs affects neuronal activity and contributes significantly to the overall functioning of the nervous system. Understanding synaptic integration is essential for exploring how the brain processes information.
The Process of Synaptic Integration
Synaptic integration involves the combination of several synaptic inputs to produce a unified response in the postsynaptic neuron. This complex process includes several key components:
- Resting Membrane Potential: Neurons maintain a resting potential due to the distribution of ions across their membranes, which prepares them for synaptic signaling.
- Synaptic Inputs: Neurons receive signals from other neurons via various synapses, which can be excitatory or inhibitory, leading to alterations in membrane potential.
- Spatial Summation: This occurs when postsynaptic potentials from different locations on the neuron are processed nearly simultaneously.
- Temporal Summation: Multiple synaptic potentials occurring at the same site in quick succession can accumulate to affect the neuron significantly.
- Action Potential Generation: When the integrated synaptic inputs produce a substantial change in the membrane potential, an action potential may be triggered, allowing the neuron to relay the signal.
Imagine a neuron that receives excitatory inputs from several sensory neurons and inhibitory input from an interneuron. The excitatory inputs promote depolarization, while the inhibitory input promotes hyperpolarization at the same moment. If the cumulative excitatory effects (via spatial and temporal summation) outpace the inhibitory ones, the postsynaptic neuron could reach the threshold necessary to initiate an action potential.
Not every signal that a neuron receives will result in an action potential. A neuron only fires when the combined synaptic inputs exceed a specific threshold.
Delving deeper into the molecular basis of synaptic integration, it's fascinating to understand how synaptic weights are calculated. The balance between excitation and inhibition is regulated by numerous factors, such as the availability of neurotransmitters, the density and type of receptors present, and the recent history of synaptic activity. Moreover, synaptic plasticity allows changes in synaptic strength over time, which is crucial for learning and memory. Inhibitory synapses, often localized on the soma and dendritic shaft, can shunt excitatory current, a mechanism termed 'shunting inhibition,' often playing a significant role in modulating neuronal output.
How Do Neurons Integrate Synaptic Inputs
Neurons are the basic units of the brain and nervous system, playing a vital role in integrating various synaptic inputs to produce complex responses. Understanding how neurons handle these inputs sheds light on a wide range of biological and cognitive processes. The integration of synaptic inputs depends on various factors contributing to the holistic response of a neuron.Neurons summate synaptic inputs through a process influenced by the nature and timing of synaptic signals. Here's a closer look into how this intricate process works.
Mechanisms of Synaptic Integration
Synaptic integration is regulated by several mechanisms, allowing neurons to process and respond to a diverse array of inputs:
- Electrotonic Properties: These refer to the passive electrical properties of a neuron, affecting how signals decay as they travel across dendrites to the soma.
- Synaptic Summation: This involves the summation of excitatory and inhibitory postsynaptic potentials at the axon hillock, which determines whether an action potential will be fired.
- Non-linear Integration: Dendritic spines can perform complex computations due to non-linear summation properties, potentially amplifying certain inputs.
The axon hillock is the part of the neuron where the accumulated potentials are evaluated to decide if an action potential should be generated.
Consider a neuron receiving three excitatory inputs and two inhibitory inputs:If one excitatory input contributes a +5 mV change, another a +3 mV and the third a +4 mV, while the inhibitory inputs provide -4 mV and -3 mV respectively:The total potential change would be: \[(5 + 3 + 4) + (-4 - 3) = +5\] mV above the resting potential. If this +5 mV exceeds the threshold for firing an action potential, the neuron will likely fire.
Synaptic integration can be understood more deeply through the lens of cable theory, which models neurons as cylindrical cables. This idea helps in approximating how passive potentials decay based on distance from the stimulus origin. Additionally, the Hodgkin-Huxley model describes how action potentials are generated and propagated, providing insight into how ionic conductances shape neuronal response. These models offer profound insights into how spatial factors and membrane properties impact synaptic input integration.
Excitatory inputs typically involve neurotransmitters like glutamate, whereas inhibitory inputs often involve GABA. These neurotransmitters determine the nature of postsynaptic potentials.
Importance of Synaptic Integration
Synaptic integration is crucial because it underpins how neurons communicate and how complex information is processed in the brain. Understanding this process provides insights into both normal brain function and neurological disorders. It involves the coordination of excitatory and inhibitory inputs, allowing neurons to integrate signals coming from various sources before deciding on an appropriate output. This ability is essential for maintaining brain plasticity, learning, and memory.
Synaptic Integration Mechanisms
Neurons use several mechanisms for synaptic integration. These mechanisms involve both passive and active properties of neurons that influence how signals are processed and the eventual output generated.
- Membrane Properties: The neuron's membrane properties, such as capacitance and resistance, play a role in determining how quickly the membrane potential changes in response to synaptic inputs.
- Location of Synapses: Inputs closer to the axon hillock have a greater impact on action potential initiation than those farther away.
- Synaptic Plasticity: Changes in the strength of synaptic connections over time can affect how neurons integrate inputs, contributing to learning and memory.
The axon hillock is a critical region within a neuron that acts as the site for deciding whether or not an action potential will be initiated based on integrated synaptic inputs.
A deeper understanding of synaptic integration reveals fascinating phenomena such as coincidence detection and input tuning. Coincidence detection refers to a neuron's sensitivity to inputs arriving simultaneously, which can often lead to action potential generation. In the auditory system, for instance, neurons are finely tuned to integrate inputs to detect small differences in sound timing, critical for sound localization. The role of dendritic branches adds another layer, where nonlinear properties allow for localized computations. This nonlinearity can enhance or dampen synaptic inputs, thus greatly influencing overall neuronal response.
Synaptic Integration Example
To illustrate synaptic integration, consider the following example. Imagine a postsynaptic neuron receiving simultaneous inputs from two excitatory and one inhibitory presynaptic neurons. Each of the excitatory inputs depolarizes the membrane by +3 mV, while the inhibitory input hyperpolarizes the membrane by -4 mV. The resultant change in membrane potential is determined by:\[ (3 + 3 - 4) \text{ mV} = +2 \text{ mV} \]This means that if the neuron had a resting potential of -65 mV, the combined inputs will alter the potential to -63 mV. If -63 mV lies above the threshold potential required to trigger an action potential, the neuron will fire.
Consider another neuron receiving both excitatory and inhibitory inputs with the following values:
- Excitatory Inputs: +7 mV and +5 mV
- Inhibitory Input: -6 mV
In real scenarios, neurons deal with hundreds or thousands of inputs at a time. They integrate these extensively to decide whether an overall excitatory or inhibitory effect prevails.
synaptic integration - Key takeaways
- Synaptic Integration: The process by which neurons combine multiple synaptic inputs to decide about firing an action potential.
- Importance: Synaptic integration affects neuronal activity and is essential for brain information processing, learning, and memory.
- Mechanisms: Synaptic integration involves temporal and spatial summation, electrotonic properties, and synaptic plasticity.
- Neuron Input Processing: Neurons evaluate excitatory and inhibitory inputs at axon hillock, determining action potential generation.
- Example: A neuron with more excitatory than inhibitory inputs may reach the threshold, triggering an action potential.
- Neuronal Functionality: Synaptic integration allows neurons to assess signal combinations, crucial for maintaining brain plasticity and cognitive functions.
Learn faster with the 12 flashcards about synaptic integration
Sign up for free to gain access to all our flashcards.
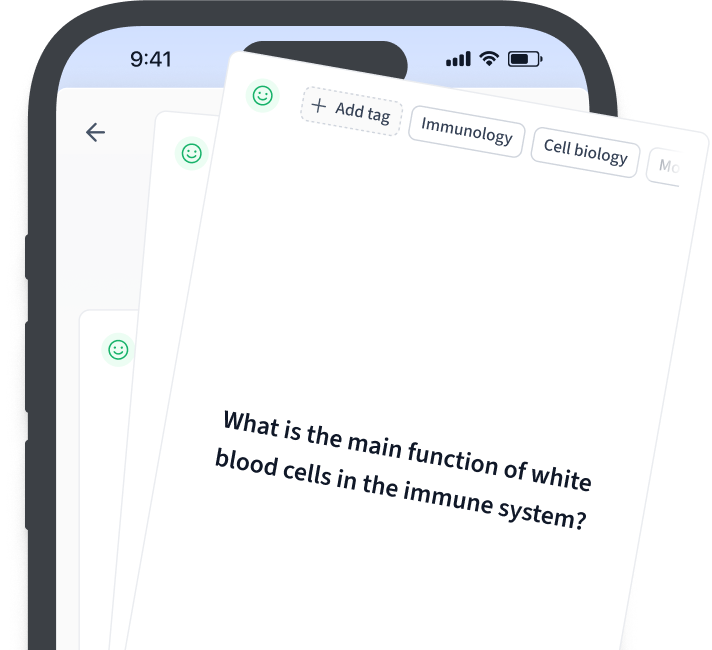
Frequently Asked Questions about synaptic integration
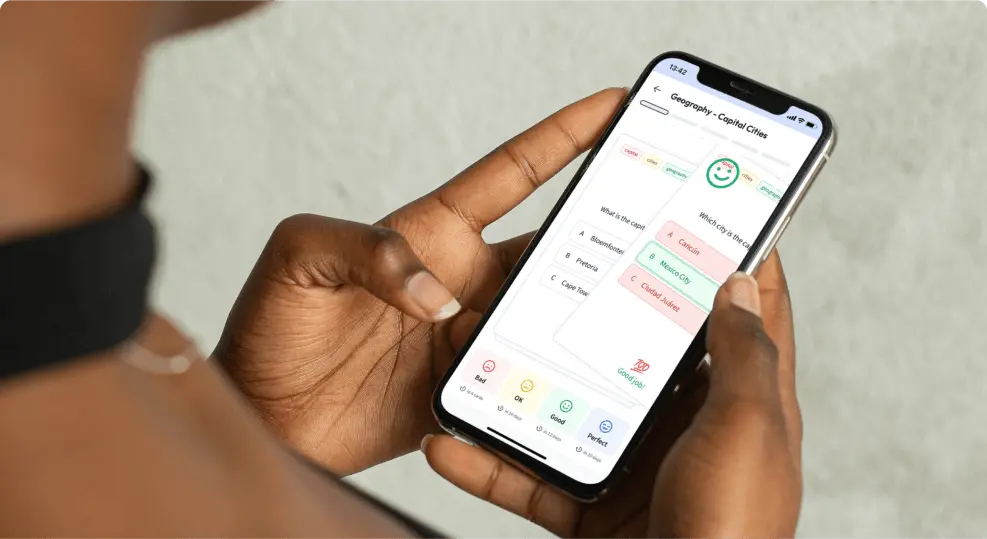
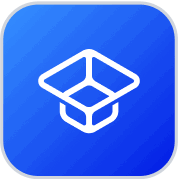
About StudySmarter
StudySmarter is a globally recognized educational technology company, offering a holistic learning platform designed for students of all ages and educational levels. Our platform provides learning support for a wide range of subjects, including STEM, Social Sciences, and Languages and also helps students to successfully master various tests and exams worldwide, such as GCSE, A Level, SAT, ACT, Abitur, and more. We offer an extensive library of learning materials, including interactive flashcards, comprehensive textbook solutions, and detailed explanations. The cutting-edge technology and tools we provide help students create their own learning materials. StudySmarter’s content is not only expert-verified but also regularly updated to ensure accuracy and relevance.
Learn more