Jump to a key chapter
Definition of Clinical Metabolomics
In modern medicine, clinical metabolomics is an emerging and exciting field that provides a comprehensive look into metabolic processes. It involves analyzing small molecules, known as metabolites, within biological samples to understand diseases, drug responses, and other clinical outcomes.
Clinical Metabolomics Explained
Clinical metabolomics is the application of metabolomics strategies to clinical settings. It seeks to give insights into various diseases and tailor healthcare approaches.
Clinical Metabolomics is the study of metabolites found within biological samples, used to provide insights into disease mechanisms, drug interactions, and personalized medical treatments.
When you delve into clinical metabolomics, several core concepts and methodologies are involved:
- Metabolites: These are small molecules found in blood, urine, and other tissue samples that reflect physiological states.
- Mass Spectrometry (MS): A key technique used to identify and quantify metabolites with high sensitivity.
- Nuclear Magnetic Resonance (NMR) Spectroscopy: Another technique that offers a detailed analysis of metabolite structure and concentration.
An example of clinical metabolomics in action is its use in oncology. By analyzing tumor tissue samples, doctors can identify metabolomic profiles that help in distinguishing between aggressive and non-aggressive cancers, thereby guiding treatment choices.
Metabolomic analysis does not only provide disease diagnosis but also monitors treatment efficacy and patient compliance.
The advancement of technology in clinical metabolomics has enabled the creation of databases that support extensive metabolite profiling. This allows researchers and clinicians to draw parallels between metabolomic data and disease states across large populations. By utilizing bioinformatics tools, clinicians can now relate variations in metabolomic profiles to genetic variances, enhancing the understanding of complex diseases at a systems biology level.
Techniques Used in Clinical Metabolomics
As clinical metabolomics evolves, various techniques have become essential in the analysis of metabolites within biological samples. These techniques provide valuable insights into health and disease conditions.
Mass Spectrometry in Clinical Metabolomics
One of the cornerstone techniques in clinical metabolomics is Mass Spectrometry (MS). It enables the precise measurement and identification of metabolites through the assessment of ions based on their mass-to-charge ratio. This technique is valued for its sensitivity and accuracy.
- High Sensitivity: Mass Spectrometry can detect metabolites at very low concentrations.
- High Throughput: Multiple samples can be processed quickly, making it ideal for large-scale studies.
In a clinical setup, mass spectrometry can be used to identify metabolic changes in patients undergoing treatment for liver disorders, enabling doctors to adjust therapies based on metabolic responses.
Always ensure sample preparation is carefully conducted to avoid contamination and ensure accurate results in mass spectrometry.
Nuclear Magnetic Resonance (NMR) Spectroscopy
Another vital technique is Nuclear Magnetic Resonance (NMR) Spectroscopy. This approach provides structural information about metabolites by exploiting the magnetic properties of atomic nuclei. It's advantageous due to its non-destructive nature.
- Structural Elucidation: NMR helps in determining the structures of newly identified metabolites.
- Reproducibility: With high consistency, NMR ensures that results are reliable and comparable across different studies.
Nuclear Magnetic Resonance (NMR) Spectroscopy is a technique used to study the structure and dynamics of molecules in solution with the aid of magnetic fields.
Liquid Chromatography-Mass Spectrometry (LC-MS)
Combining Liquid Chromatography (LC) and Mass Spectrometry, LC-MS provides enhanced separation and detection of metabolites. It is extensively used in complex sample analysis:
- Comprehensive Analysis: Allows separation of complex mixtures before analysis, minimizing interference.
- Efficiency: Suitable for both targeted and untargeted metabolomics studies.
Clinical metabolomics through LC-MS is making strides in personalized medicine. Its ability to quantitatively analyze metabolites opens pathways to predict patient responses to drugs, minimizing adverse effects and maximizing therapeutic efficacy. Researchers are continuously refining LC-MS methodologies to improve sensitivity further and expand its applications, from disease biomarker discovery to environmental metabolomics.
Translating Metabolomics into Clinical Practice
The integration of metabolomics into clinical practice holds promise for revolutionizing medical research and treatment approaches. It involves adapting metabolomics methodologies to understand better and treat diseases by tracking biochemical changes in the body.
Importance of Clinical Metabolomics in Medical Research
The field of clinical metabolomics significantly enhances medical research by offering a deeper insight into disease pathophysiology. It contributes by:
- Personalized Medicine: Tailoring treatments based on individual metabolic profiles.
- Disease Biomarkers: Discovering biomarkers for early disease detection and monitoring disease progression.
- Pharmacometabolomics: Predicting drug efficacy and toxicity, improving drug development.
Biomarker: A measurable indicator of a biological state or condition, often used in diagnosing diseases or assessing health.
For instance, in medical research, clinical metabolomics can identify specific biomarkers linked to Type 2 Diabetes by analyzing blood samples. This aids in early diagnosis and targeted therapy.
Collaborative research combining genetics and metabolomics could amplify the discovery of novel therapeutic targets.
Beyond traditional research approaches, clinical metabolomics is paving the way for new scientific inquiries. Researchers focus on metabolic pathways that influence not just common diseases but also rare genetic disorders. The development of integrative databases that marry metabolomic data with genomic and proteomic information allows for investigations into systems biology. This comprehensive outlook enhances understanding of intricate biological processes and leads to the development of multi-targeted therapies, benefiting conditions like complex neurodegenerative diseases.
Field | Application |
Cardiology | Identifying risk factors for heart diseases. |
Oncology | Understanding cancer metabolism and drug resistance. |
Neurology | Exploring metabolic changes in Alzheimer's disease. |
Metabolomics in Cancer Research
Cancer research significantly benefits from the application of metabolomics. By examining metabolites in cancerous tissues, researchers can uncover vital information about tumor biology, progression, and response to treatment.
Emerging Applications in Clinical Oncology
The field of clinical oncology has seen rapid integration of metabolomics to enhance cancer diagnosis, prognosis, and therapy. Researchers utilize metabolomics to:
- Identify Biomarkers: Discover unique metabolic markers that distinguish cancerous cells from normal cells, aiding in early detection and monitoring.
- Understand Cancer Metabolism: Explore how cancer cells alter their metabolism to support rapid growth and survival, leading to new therapeutic targets.
- Personalize Treatment: Use metabolic profiles to tailor treatments according to individual patient needs, potentially increasing treatment efficacy and reducing side effects.
Biomarker: In oncology, a biomarker can be a biological molecule found in blood, other body fluids, or tissues indicating a normal or abnormal process, or a condition or disease.
For example, in breast cancer, metabolomic studies have identified specific lipid metabolites that correlate with tumor aggressiveness. This information is crucial for oncologists when deciding between more aggressive treatment options and conservative management.
Using advanced technologies such as Mass Spectrometry (MS) and Nuclear Magnetic Resonance (NMR) Spectroscopy, cancer metabolomics provides a detailed metabolic landscape of cancer cells. This enables scientists to unveil the unique signatures of different cancer types.
Emerging metabolomic techniques could soon allow for non-invasive cancer diagnoses through simple blood tests.
In-depth research within cancer metabolomics focuses on the role of the tumor microenvironment in shaping metabolic pathways. Scientists are investigating how interactions between cancer cells, stromal cells, and immune cells influence metabolism and drive cancer progression. By understanding these complex interactions, novel treatments can be developed that disrupt these pathways specifically, offering targeted therapies with potentially fewer side effects than conventional chemotherapy. Additionally, this research lends itself to the exciting potential of using metabolomics not only to treat existing cancers but also to predict and prevent cancer development in at-risk populations.
clinical metabolomics - Key takeaways
- Definition of Clinical Metabolomics: Study of small molecules called metabolites within biological samples to understand diseases, drug responses, and personalize medical treatments.
- Techniques Used in Clinical Metabolomics: Key techniques include Mass Spectrometry (MS) for sensitivity and Nuclear Magnetic Resonance (NMR) Spectroscopy for structural analysis.
- Translating Metabolomics into Clinical Practice: Metabolomics allows tracking biochemical changes to improve disease understanding and treatment, emphasizing personalized medicine.
- Metabolomics in Cancer Research: Provides insights into tumor biology, cancer progression, and guides treatment choices based on metabolic profiles.
- Emerging Applications in Clinical Oncology: Identifying biomarkers, understanding cancer metabolism, and personalizing treatments are key metabolic applications in oncology.
- Importance of Clinical Metabolomics in Medical Research: Enhances understanding of disease mechanisms, drug interactions, and supports the development of targeted therapies.
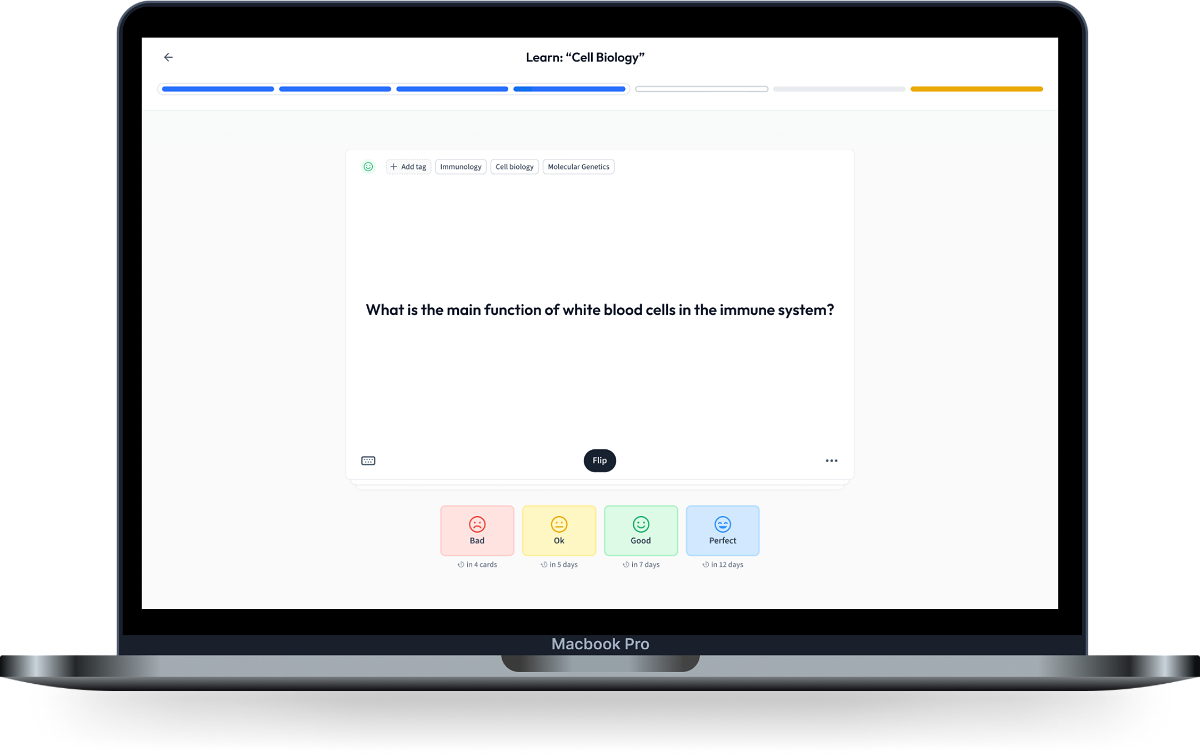
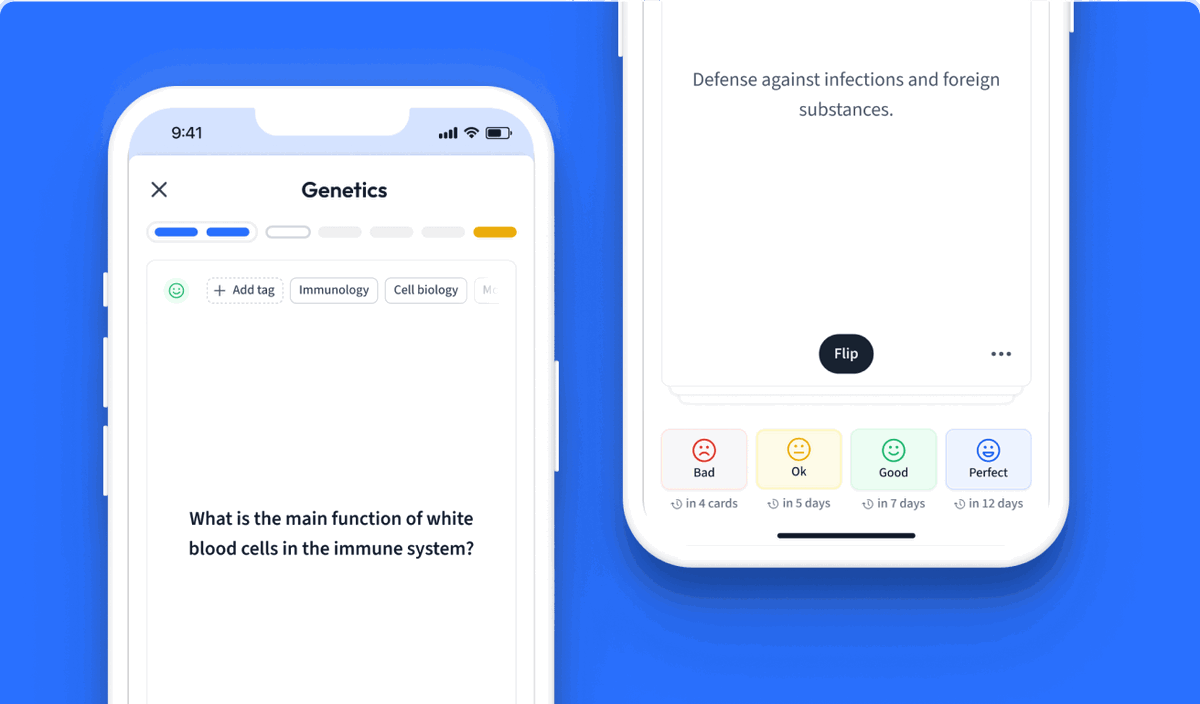
Learn with 12 clinical metabolomics flashcards in the free StudySmarter app
We have 14,000 flashcards about Dynamic Landscapes.
Already have an account? Log in
Frequently Asked Questions about clinical metabolomics
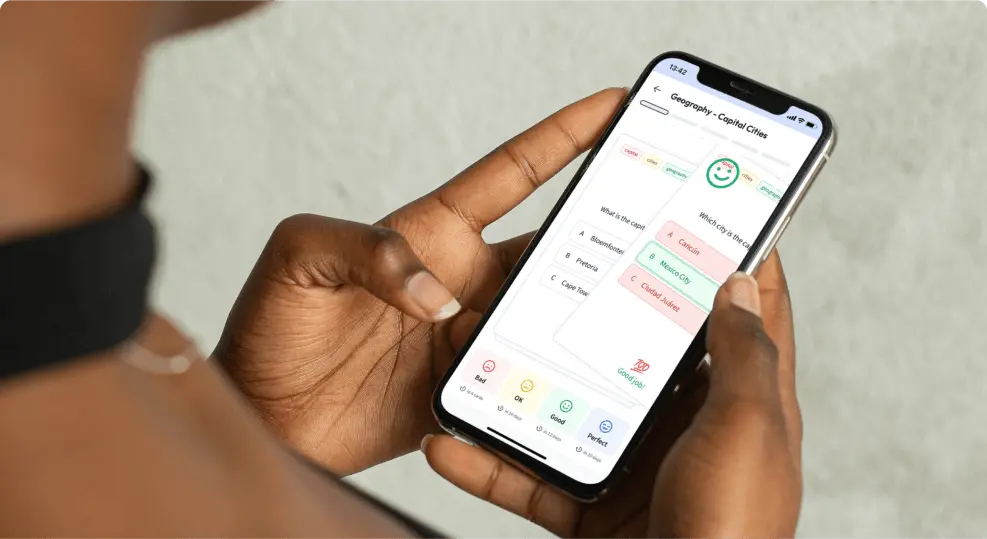
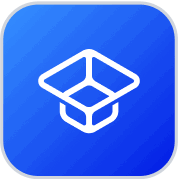
About StudySmarter
StudySmarter is a globally recognized educational technology company, offering a holistic learning platform designed for students of all ages and educational levels. Our platform provides learning support for a wide range of subjects, including STEM, Social Sciences, and Languages and also helps students to successfully master various tests and exams worldwide, such as GCSE, A Level, SAT, ACT, Abitur, and more. We offer an extensive library of learning materials, including interactive flashcards, comprehensive textbook solutions, and detailed explanations. The cutting-edge technology and tools we provide help students create their own learning materials. StudySmarter’s content is not only expert-verified but also regularly updated to ensure accuracy and relevance.
Learn more