Jump to a key chapter
Definition of Genomic Heterogeneity
Genomic heterogeneity refers to the diversity in the genetic makeup that can be found within the cells of a single individual or across populations. This phenomenon is crucial for understanding the complexity and variations that exist in biological systems and diseases.
Genome Heterogeneity Basics
The concept of genome heterogeneity is foundational in genetics and involves several critical aspects:
- DNA Variability: Not every cell in an organism will have an identical set of DNA due to mutations and other genetic variations that occur naturally.
- Somatic Mutations: These are alterations that occur in non-germ cells and can accumulate over the lifetime of an organism.
- Genetic Mosaicism: This occurs when different cells within the same individual have different genetic compositions, often due to mitotic errors or mutations.
- Polymorphism: The presence of multiple genetic variants or alleles within a population that leads to individual differences but does not necessarily cause disease.
Genomic Heterogeneity is the variation in genetic makeup across different cells and individuals, caused by mutations, genetic mosaicism, and polymorphisms.
Imagine a population of butterflies where some have black spots on their wings, while others do not. This variation is an example of genomic heterogeneity within a species.
Cancer Genome Heterogeneity Meaning
In the context of cancer, genomic heterogeneity becomes a critical factor in understanding tumor behavior and treatment challenges. Here’s why it’s important:
- Tumor Evolution: As cancer progresses, it accumulates genetic mutations, leading to diverse cancer cell populations within the same tumor.
- Treatment Resistance: Different genetic variants within a tumor may respond differently to treatments, which can result in treatment-resistant cancer cells surviving.
- Metastasis: Certain genetic changes can enable cancer cells to spread and colonize distant organs.
- Clonal Expansion: Refers to a tumor's growth driven by specific cell groups with advantageous mutations.
Intratumor Heterogeneity is a specific type of genomic heterogeneity observed within a single tumor mass. This complexity is due to the presence of different clones of cancer cells, each having unique mutations and phenotypic traits. Understanding intratumor heterogeneity is vital in predicting tumor behavior, treatment outcomes, and identifying therapeutic targets.
The ability of cancer cells to evolve and adapt is one reason why early detection and continuous monitoring are essential in cancer treatment.
Examples of Genomic Heterogeneity in Diseases
Genomic heterogeneity is prevalent among various diseases, significantly impacting their development and treatment. Understanding this diversity helps in crafting personalized medical approaches and recognizing disease progression patterns.
Genomic and Phenotypic Heterogeneity in Prostate Cancer
Prostate cancer is a prime example of genomic heterogeneity with multiple genomic alterations seen across patients. These alterations contribute to varying phenotypic expressions of the disease:
- Genetic Variations: DNA mutations such as deletions, amplifications, and point mutations occur across different gene loci.
- Epigenetic Changes: Alterations in DNA methylation and histone modification affect gene expression without changing the DNA sequence.
- Androgen Receptor Variability: Mutations and amplifications in androgen receptors may lead to different responses to hormonal therapies.
- Single Nucleotide Polymorphisms (SNPs): Diverse SNPs across populations contribute to differences in disease risk and progression.
Genomic Heterogeneity refers to the presence of diverse genetic variations within and between individual tumors, affecting disease characteristics and treatment outcomes.
Consider two prostate cancer patients: one shows sensitivity to androgen deprivation therapy, while the other does not. This difference can be attributed to heterogeneity in androgen receptor gene mutations.
Clonal Evolution in Prostate Cancer illustrates how genomic heterogeneity manifests within tumors. As cancer progresses, certain cell lines, or clones, expand due to beneficial mutations. This can be modeled mathematically by analyzing the growth rate of clones. For instance, if a clone growth is represented by \(N(t) = N_0 e^{rt}\), where \(N_0\) is the initial number of cells, \(r\) is the growth rate, and \(t\) is time, changes in \(r\) and initial conditions can help understand which mutations lead to aggressive phenotypes.
Prostate cancer genomic profiling is increasingly used to tailor treatment protocols and predict therapy outcomes more accurately.
Techniques for Identifying Genomic Heterogeneity
Identifying genomic heterogeneity involves various techniques that allow researchers to understand the genetic diversity within and between organisms. These tools enable accurate disease diagnosis, personalized treatment strategies, and insights into evolutionary processes.
Current Methods for Genome Analysis
Several established methods are used for genome analysis, each with its own strengths:
- Whole Genome Sequencing (WGS): Captures the entire genomic sequence, allowing for comprehensive mutation analysis and discovery of novel genetic variants.
- Exome Sequencing: Focuses on the protein-coding regions of the genome, known as exons, providing insights into specific gene functions and mutations related to diseases.
- Single-cell Sequencing: Enables the study of genomic variations at the single-cell level, revealing intratumor heterogeneity.
- Chromosomal Microarray Analysis (CMA): Detects chromosomal imbalances such as deletions and duplications that may not be evident through sequencing alone.
Whole Genome Sequencing (WGS) is a process that determines the complete DNA sequence of an organism's genome at a single time.
An example of genomic analysis is the use of whole genome sequencing to identify BRCA1 and BRCA2 mutations, which are associated with increased breast cancer risk. By analyzing the entire genome, researchers can detect these specific mutations and provide genetic counseling.
In mathematics, algorithms used for sequencing often utilize graph theory. Consider the challenge of sequence assembly, where DNA fragments need to be arranged to form the complete genome. A directed graph can be used where nodes represent sequence fragments, and edges connect fragments that overlap. This assembly problem can be approached using the Eulerian Path, where each edge is visited exactly once. The mathematical challenge is akin to solving the equation for path existence: \[\text{{Find }} P : V \times V \to E\]where \(E\) is the set of edges, and \(V\) is the set of vertices representing the sequence fragments.
Single-cell sequencing can unravel the intricacies of cellular heterogeneity, making it pivotal in cancer research and personalized therapy development.
Advances in Genomic Mapping
Recent advances in genomic mapping technology have significantly enhanced our ability to analyze diverse genomes efficiently:
- Long-Read Sequencing Technologies: These allow for the sequencing of large strands of DNA in a single read, reducing errors associated with repetitive sequences.
- Optical Mapping: Provides a high-resolution, physical map of the genome using fluorescent markers placed along DNA molecules.
- CRISPR-based Mapping: Utilizes targeted genome editing to map specific genes and regulatory elements, allowing precise annotation of genetic regions.
- Machine Learning Algorithms: Applied to process large genomic datasets, these algorithms predict functional elements and potential mutations within the genome.
Optical Mapping is a technique for constructing maps of the genome by examining DNA patterns using fluorescent labels.
Optical mapping has been crucial in resolving the complex structural variations in the maize genome, revealing substantial differences between various strains that impact traits like stress tolerance and productivity.
Implications of Genomic Heterogeneity
Understanding genomic heterogeneity offers profound insights into personalized medicine and the challenges in treatment resulting from genomic diversity. As you delve into this topic, you'll uncover how individual genetic variations can shape treatment outcomes and how these challenges are addressed.
Genomic Heterogeneity in Personalized Medicine
Personalized Medicine leverages genomic heterogeneity by tailoring medical treatment to the individual characteristics of each patient. This approach considers genetic differences, lifestyle, and environmental factors.
- Customized Therapies: Treatments can be adapted based on the patient's genetic profile, ensuring that they are more effective and cause fewer side effects.
- Risk Assessment: Genetic screening helps in assessing the risk of developing certain diseases, allowing for preventive strategies.
- Pharmacogenomics: Analyzes how genes affect a person's response to drugs, aiding in the selection of the most suitable medication.
- Genetic Counseling: Provides insights into hereditary conditions, helping patients understand and manage their health better.
Pharmacogenomics involves studying variations in drug metabolism genes, such as CYP450, which influence how drugs are metabolized and can significantly affect drug efficacy and safety. For example, variations in the CYP2C19 gene may alter the metabolism of drugs like clopidogrel, necessitating alternative treatments or dosages.
Personalized medicine can significantly reduce healthcare costs by minimizing ineffective treatments and focusing resources on targeted therapies.
Challenges in Treatment Due to Genomic Diversity
While genomic heterogeneity opens the door to personalized medicine, it also poses several challenges that impact treatment strategies:
- Complexity in Diagnosis: The presence of multiple genetic variations can complicate accurate diagnosis and hinder effective treatment planning.
- Treatment Resistance: Genomic diversity can lead to treatment-resilient cell populations, emerging as a significant hurdle in conditions like cancer.
- Diverse Response Rates: Patients with seemingly similar conditions might respond differently to the same treatment due to underlying genetic dissimilarities.
- Data Management: Handling and interpreting large volumes of genomic data require advanced computational tools and expertise.
Consider a clinical scenario where two patients with the same type of breast cancer receive the same chemotherapy regimen. One patient experiences significant tumor reduction, while the other shows minimal response. Detailed genomic testing may reveal differences in specific mutations, impacting drug sensitivity and resistance.
In tackling treatment resistance, precision oncology utilizes advanced models like patient-derived xenografts (PDXs) and organoids to simulate and analyze tumor growth patterns under various treatments. These models provide insights into potential resistance mechanisms and help in evaluating new therapeutic options before clinical application.
Multi-omics approaches, combining genomics with transcriptomics and proteomics, are increasingly used to improve understanding and prediction of genomic diversity impact on treatment outcomes.
genomic heterogeneity - Key takeaways
- Genomic heterogeneity: Refers to the diversity in genetic makeup within an individual or across populations, affecting biological systems and diseases.
- Genome heterogeneity concepts: Includes DNA variability, somatic mutations, genetic mosaicism, and polymorphism.
- Cancer genome heterogeneity: Involves diverse genetic cell populations within tumors, impacting tumor behavior and treatment responses.
- Examples of genomic heterogeneity in diseases: Prostate cancer shows genomic differences like genetic variations, epigenetic changes, and androgen receptor variability.
- Techniques for identifying genomic heterogeneity: Include whole genome sequencing, exome sequencing, single-cell sequencing, and chromosomal microarray analysis.
- Genomic and phenotypic heterogeneity in prostate cancer: Explains variations in DNA mutations and different phenotypes, affecting treatment responses.
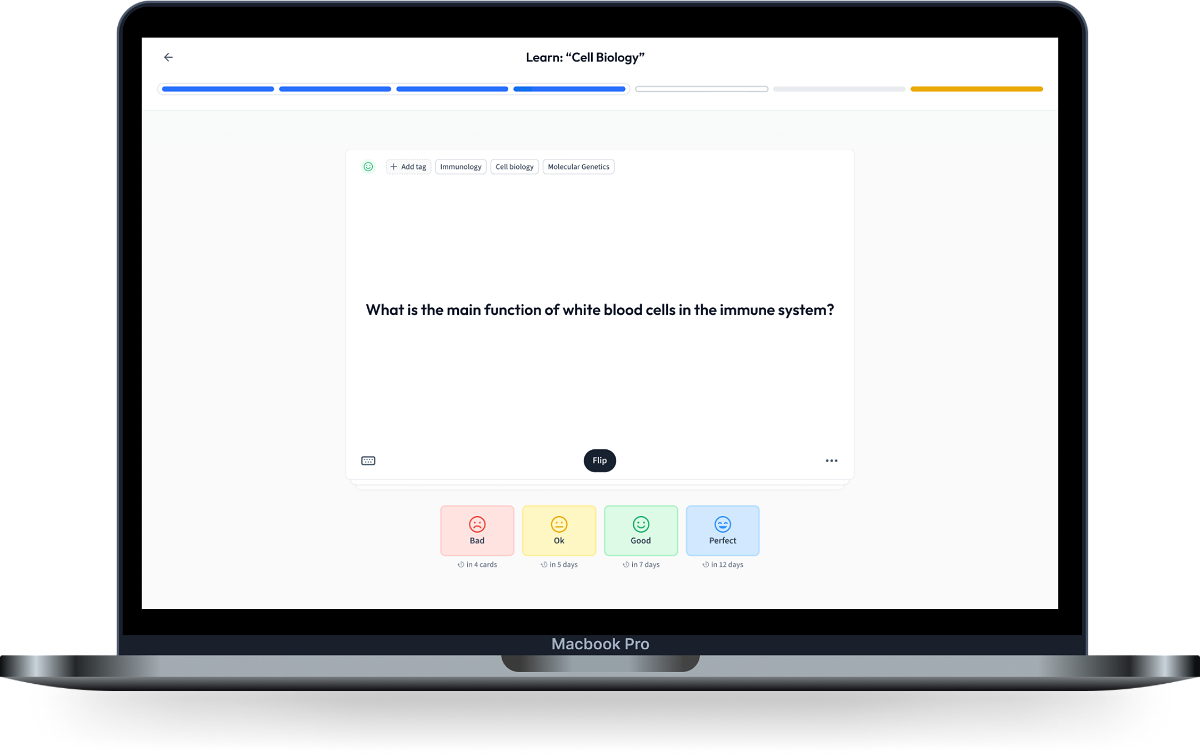
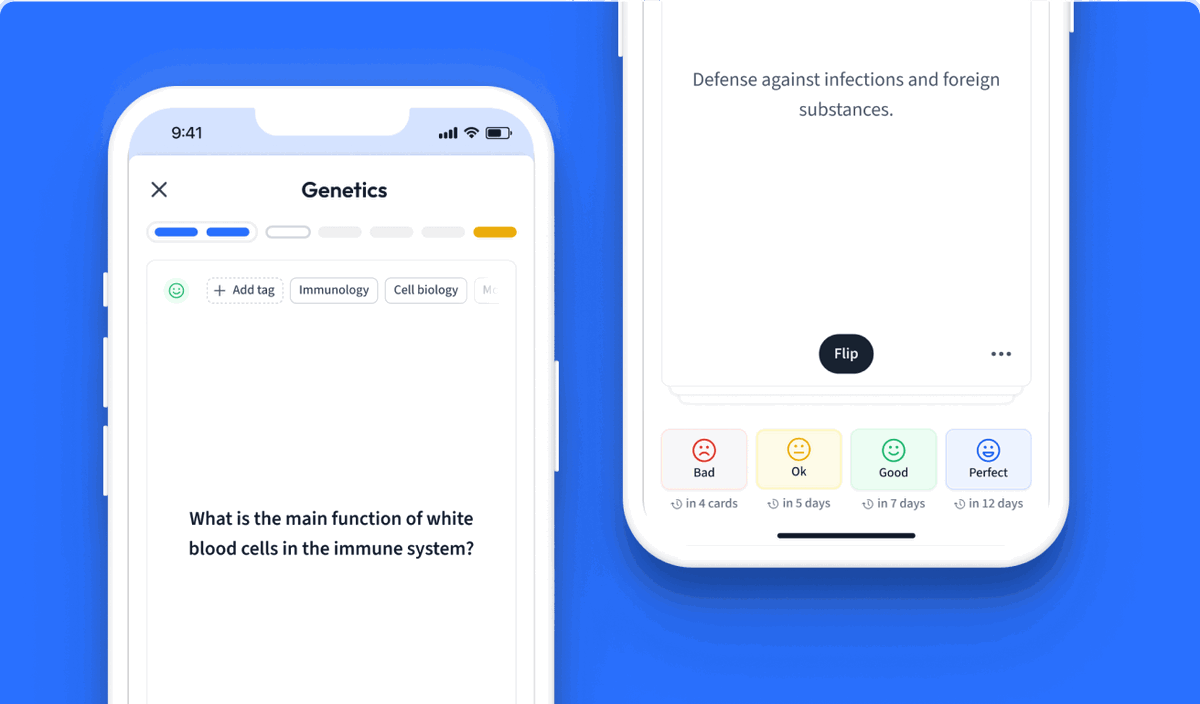
Learn with 12 genomic heterogeneity flashcards in the free StudySmarter app
Already have an account? Log in
Frequently Asked Questions about genomic heterogeneity
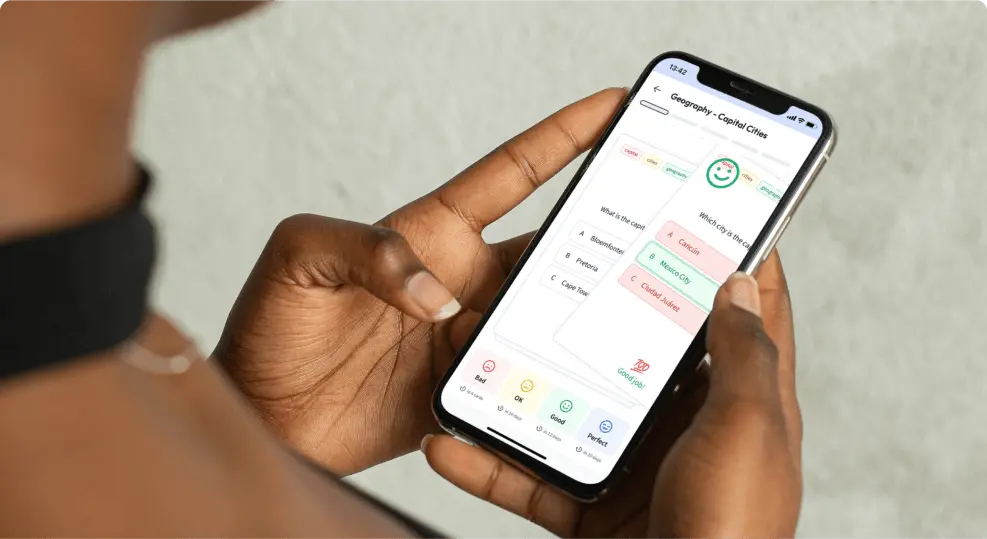
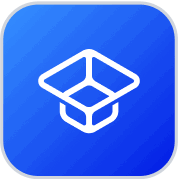
About StudySmarter
StudySmarter is a globally recognized educational technology company, offering a holistic learning platform designed for students of all ages and educational levels. Our platform provides learning support for a wide range of subjects, including STEM, Social Sciences, and Languages and also helps students to successfully master various tests and exams worldwide, such as GCSE, A Level, SAT, ACT, Abitur, and more. We offer an extensive library of learning materials, including interactive flashcards, comprehensive textbook solutions, and detailed explanations. The cutting-edge technology and tools we provide help students create their own learning materials. StudySmarter’s content is not only expert-verified but also regularly updated to ensure accuracy and relevance.
Learn more