Jump to a key chapter
Evidence Synthesis Definition and Examples
In the field of medicine, evidence synthesis is a pivotal process used to aggregate data from multiple studies to derive conclusions that inform healthcare decisions. By collating evidence from various sources, evidence synthesis provides a comprehensive overview, often illuminating insights that would not be evident from individual studies alone.
Understanding Evidence Synthesis
Evidence synthesis is crucial in the realm of medical research. It allows you to combine data from different studies to answer specific questions. This process involves numerous methodologies, each tailored to different types of research questions. Here's a general breakdown of the most common methodologies used in evidence synthesis:
- Systematic Reviews: These reviews compile all empirical evidence that fits pre-specified eligibility criteria to answer a specific research question. Systematic reviews aim to minimize bias by using explicit, systematic methods.
- Meta-Analysis: This method combines the results of several studies addressing a set of related research hypotheses. By statistically pooling data, a meta-analysis can provide a more precise estimate of the effects of healthcare interventions.
- Narrative Reviews: Unlike systematic reviews, narrative reviews provide a summary of what is known on a topic, typically without strict methodological rules.
Evidence Synthesis: A procedure for collating data from various research studies to construct comprehensive insights and conclusions.
An example of evidence synthesis in action is when researchers perform a systematic review of clinical trials to evaluate the effectiveness of a new drug. By synthesizing results from multiple trials, they can determine the drug's efficacy and safety profile more accurately than any single study could.
Diving deeper into the processes, you might encounter complex statistical techniques involved in meta-analysis, such as random-effects and fixed-effects models. These models address variability between study results. For instance, the random-effects model considers that different studies are estimating somewhat different treatment effects and assumes a distribution of the true effects across studies. Meanwhile, the fixed-effects model operates under the assumption that all studies are estimating the same underlying effect, with variations due to chance.
Understanding the difference between systematic reviews and narrative reviews is key to distinguishing evidence synthesis approaches effectively.
Key Examples in Medical Research
Evidence synthesis is extensively used in medical research to enhance understanding and guide clinical practice. Below are some notable examples illustrating its application:
- Evaluating Treatment Efficacy: In areas such as oncology, evidence synthesis has been used to evaluate the efficacy of chemotherapy regimens by combining data from various trials to determine which treatments offer the best survival benefits.
- Guideline Development: For creating clinical guidelines, organizations often use systematic reviews and meta-analyses to compile and evaluate evidence to provide recommendations for practice, such as the management of chronic illnesses.
- Health Policy Making: Policymakers utilize evidence synthesis to understand the effectiveness and cost-effectiveness of interventions, aiding in resource allocation and public health strategies.
An example can be seen in the development of COVID-19 treatment protocols. During the pandemic, healthcare organizations used evidence synthesis to analyze data from global clinical studies regarding the use of antiviral drugs, helping to form guidelines that improved patient outcomes.
Evidence Synthesis Methods
In the context of medicine, evidence synthesis involves integrating findings from multiple studies to draw comprehensive conclusions. This methodology not only provides a complete picture of the research landscape but also enhances the reliability of healthcare decisions.
Systematic Reviews
Systematic reviews are structured approaches to collating research evidence that addresses a specific question, often about the efficacy of a treatment or intervention. These reviews utilize strict protocols to ensure that all available evidence is considered, ultimately minimizing bias.
Example of a Systematic Review:An example can be found when evaluating new diabetes treatment options. When several randomized controlled trials (RCTs) provide data on a new drug, a systematic review would aggregate these to determine the average effectiveness by minimizing potential biases from single studies.
Delving deeper into systematic reviews reveals the use of methodological tools such as the PRISMA (Preferred Reporting Items for Systematic Reviews and Meta-Analyses) statement, which provides a set of standards for reporting evidence synthesis. This ensures transparency and reproducibility in the review process.Additionally, systematic reviews often use complex search strategies and inclusion/exclusion criteria to refine the studies considered for the review. This involves conducting exhaustive searches across multiple databases and sources. The goal is to ensure no relevant study is missed, therefore providing a robust conclusion. Often, statistical techniques are integrated to handle conflicting study results, providing clarity through the synthesis of diverse evidence.
Systematic reviews often serve as the foundation for developing clinical guidelines, ensuring evidence-based practice in healthcare.
Meta-Analysis Techniques
Meta-analysis serves as an extension of systematic reviews by statistically combining results from multiple studies. This process provides a quantitative estimate of the effect size, increasing precision in evaluating treatment effects.
In meta-analysis, two main statistical models are employed: the fixed-effects model and the random-effects model. Each model handles the variability among studies differently.
- Fixed-Effects Model: Assumes all studies are measuring the same underlying effect size, with differences arising purely from sampling error. Suitable when studies are homogeneous.
- Random-Effects Model: Assumes that the effect sizes vary between studies due to heterogeneity in study populations or interventions. This model is used when there is significant variability across studies.
Example of Meta-Analysis:Consider meta-analysis evaluating the effect of aspirin therapy in preventing stroke. By combining results from different studies, researchers apply statistical techniques to derive an overall effect size, adjusting for variables such as dosage and patient demographics, to determine its efficacy.
Mathematically, meta-analysis combines data using specific formulas. A common method of combining results from studies is the weighted average:\[\text{Combined Effect Size} = \frac{\sum_{i=1}^n (w_i \cdot es_i)}{\sum_{i=1}^n w_i}\]where \(w_i\) represents the weight of the \(i^{th}\) study and \(es_i\) denotes the effect size of the \(i^{th}\) study. This equation supports the integration of varied study outputs into a singular, comprehensive outcome, thereby enhancing the robustness of medical conclusions.
Techniques of Evidence Synthesis in Medicine
In medicine, evidence synthesis involves combining data from different research studies to produce a coherent and comprehensive conclusion. This process is essential for developing effective healthcare strategies and making informed clinical decisions.
Qualitative Synthesis Techniques
Qualitative synthesis techniques focus on combining textual data from diverse studies to produce a narrative understanding of the research topic. Unlike quantitative synthesis, this technique doesn't involve statistical analysis but rather explores the depth and complexity of human experiences.
Qualitative Synthesis: A non-numeric approach used to integrate insights and findings from different qualitative research studies.
In qualitative synthesis, you may come across methodologies such as:
- Thematic Synthesis: Involves identifying patterns or themes within studies to present a comprehensive account of a topic.
- Framework Synthesis: Utilizes existing theories or frameworks to categorize and interpret data from multiple studies.
- Meta-Ethnography: Focuses on interpreting cultural and social phenomena by synthesizing qualitative studies.
Example of Thematic Synthesis:An illustrative example of thematic synthesis might involve exploring patient narratives on the experience of living with chronic pain. Researchers could gather data from various qualitative studies to extract common themes, such as coping strategies, impacts on daily life, and interactions with healthcare systems.
A deeper exploration into qualitative synthesis reveals the use of advanced techniques for addressing varying study qualities. Such methods include confidence statements, which are used to indicate the robustness of synthesized findings based on the quality and quantity of data. Also, qualitative synthesis may employ triangulation to enhance validity, combining data from different sources, methods, and researchers.
Quantitative Synthesis Techniques
Quantitative synthesis, frequently achieved through meta-analysis, focuses on statistical techniques to combine numerical data from multiple studies. This technique provides a precise estimate of treatment effects, allowing for stronger and more reliable conclusions.
Quantitative Synthesis: A statistical approach used to integrate and summarize numerical data from different research studies.
Quantitative synthesis uses several statistical models, including:
- Fixed-Effects Model: Combines data assuming all studies estimate the same effect size, attributing variations to chance.
- Random-Effects Model: Accounts for variability among studies, considering differences due to real effects across diverse populations or settings.
Example of Meta-Analysis:To illustrate, consider a meta-analysis investigating the efficacy of an anti-hypertensive drug. By synthesizing results from numerous clinical trials, researchers calculate an overall effect size that indicates the average reduction in blood pressure, accounting for study sample sizes and variability.
Advanced quantitative synthesis often involves sensitivity analysis. This is used to test the robustness of the meta-analysis results by examining how varying assumptions affect outcomes. For instance, sensitivity analysis might assess the impact of excluding certain studies, ensuring that the results are robust and not heavily influenced by outliers or particular study designs.
Applications of Evidence Synthesis in Medical Research
The application of evidence synthesis in medical research is vast and multifaceted. It serves as a crucial tool in transforming individual study results into comprehensive insights that inform healthcare decisions on a global scale.
Real-World Impact
Evidence synthesis has made significant real-world impacts in the medical field. By providing aggregated data analysis, the process has contributed to wide-scale improvements in patient care and treatment outcomes.
- Drug Development: By synthesizing evidence from clinical trials, pharmaceutical companies can make informed decisions on the efficacy of drug candidates, leading to faster and more effective treatment options for patients.
- Policy Making: Health policymakers leverage evidence synthesis to develop guidelines and policies that have a substantial impact on public health, such as vaccination programs and disease prevention strategies.
- Resource Allocation: By understanding the effectiveness and cost-efficiency of various interventions through synthesized evidence, healthcare systems can optimize the allocation of resources, ensuring they provide the most benefit to patient populations.
Example of Real-World Impact:Consider the global response to the COVID-19 pandemic, where evidence synthesis played a pivotal role. With rapid accumulation and synthesis of data from diverse studies worldwide, health organizations could swiftly develop and adjust guidelines for treatment protocols and public health measures to combat the pandemic effectively.
Evidence synthesis helps bridge the gap between research and practice, ensuring that healthcare decisions are based on a solid foundation of analyzed data rather than isolated study results.
Improving Medical Guidelines
One of the primary applications of evidence synthesis in medicine is the formulation and enhancement of medical guidelines. These guidelines serve as authoritative recommendations to direct clinical practice, ensuring consistent and evidence-based patient care.
- Guideline Development: Organizations such as the World Health Organization (WHO) and the National Institute for Health and Care Excellence (NICE) rely on systematic reviews and meta-analyses of clinical data to develop comprehensive medical guidelines.
- Updating Guidelines: As new research is continually conducted, evidence synthesis allows guidelines to be promptly updated, reflecting the latest evidence and ensuring optimal patient care.
- Interdisciplinary Collaboration: By synthesizing evidence from various specialties, guidelines can address complex medical issues that require a multidisciplinary approach, fostering integrated care.
At the core of improving medical guidelines through evidence synthesis lies the GRADE (Grading of Recommendations, Assessment, Development, and Evaluations) approach. This framework is instrumental in assessing the quality of evidence and strength of recommendations in healthcare. It integrates factors like study quality, consistency of results, and overall effect size, providing a systematic approach for synthesizing evidence.The adoption of the GRADE system ensures transparency and clarity in guideline development, offering a robust mechanism for translating research findings into actionable clinical recommendations. Furthermore, it empowers clinicians with clear guidance, helping them navigate complex clinical decisions with confidence, thereby improving patient care outcomes on a global scale.
Steps in Conducting Evidence Synthesis
Conducting a thorough evidence synthesis involves several critical steps, each designed to ensure comprehensive data aggregation from various studies. By following these steps, researchers can collate, analyze, and present data effectively, thus influencing healthcare decisions.
Formulating Research Questions
The first essential step in evidence synthesis is to clearly formulate focused and relevant research questions. A well-defined research question sets the direction for the entire synthesis process and dictates the types of studies and data that will be included.To formulate a research question, consider:
- Population: Identify the specific group, disease, or population of interest.
- Intervention: Determine the treatment, procedure, or condition to be examined.
- Comparison: Establish what the intervention will be compared against (e.g., a placebo or different treatment).
- Outcome: Define the expected results or effects of the intervention.
- Timeframe: Consider the duration over which the outcomes will be measured.
PICO: A structured framework used to formulate specific research questions, encompassing Population, Intervention, Comparison, and Outcome.
Example of a Research Question:For instance, a formulated research question may be, 'In adults with hypertension (Population), does a low-sodium diet (Intervention) as compared to a standard diet (Comparison) reduce blood pressure (Outcome) over six months (Timeframe)?' This question is specific, measurable, and directly guides the selection of relevant studies.
Using the PICO framework not only clarifies your research question but also aids in database search strategies for relevant studies.
Data Collection and Analysis Techniques
For example, calculating a pooled effect size:\[\text{Effect Size}_p = \sum_{i=1}^n \frac{(w_i \cdot es_i)}{\sum_{i=1}^n w_i}\]where \(w_i\) is the weight assigned to each study as a function of its size or precision, and \(es_i\) is the effect size of each study. This pooling is done to provide an overall effect estimate with increased statistical power.
Dive deeper into data collection, and you will find that selection bias can be a thorny issue in evidence synthesis. To mitigate this, researchers employ techniques such as the publication of protocols prior to data collection, ensuring transparency and reproducibility of the process. Additionally, software tools like Covidence or RevMan are often used to facilitate efficient management and synthesis of large volumes of study data. These platforms assist in ensuring a streamlined, unbiased approach to evidence synthesis.Furthermore, sensitivity analyses often complement meta-analyses by testing the robustness of the findings against variability in study designs or populations. This includes leaving certain studies out of the analysis to see if the overall conclusions hold, thereby enhancing the reliability of the synthesized evidence.
evidence synthesis - Key takeaways
- Evidence Synthesis Definition: A process of collating data from multiple studies to create comprehensive insights and conclusions in medical research.
- Common Methods: Systematic reviews, meta-analysis, and narrative reviews are key methodologies in evidence synthesis, each with specific applications and methodologies.
- Applications in Medicine: Used for evaluating treatment efficacy, developing clinical guidelines, and informing health policy decisions.
- Meta-Analysis Models: Fixed-effects and random-effects models are used to handle variability between study results in meta-analysis.
- Steps in Conducting Evidence Synthesis: Involves formulating research questions, collecting data, analyzing results, and using techniques like PRISMA for transparency.
- Real-World Examples: Evidence synthesis was crucial in developing guidelines for COVID-19 treatment and evaluating new drug trials in other diseases.
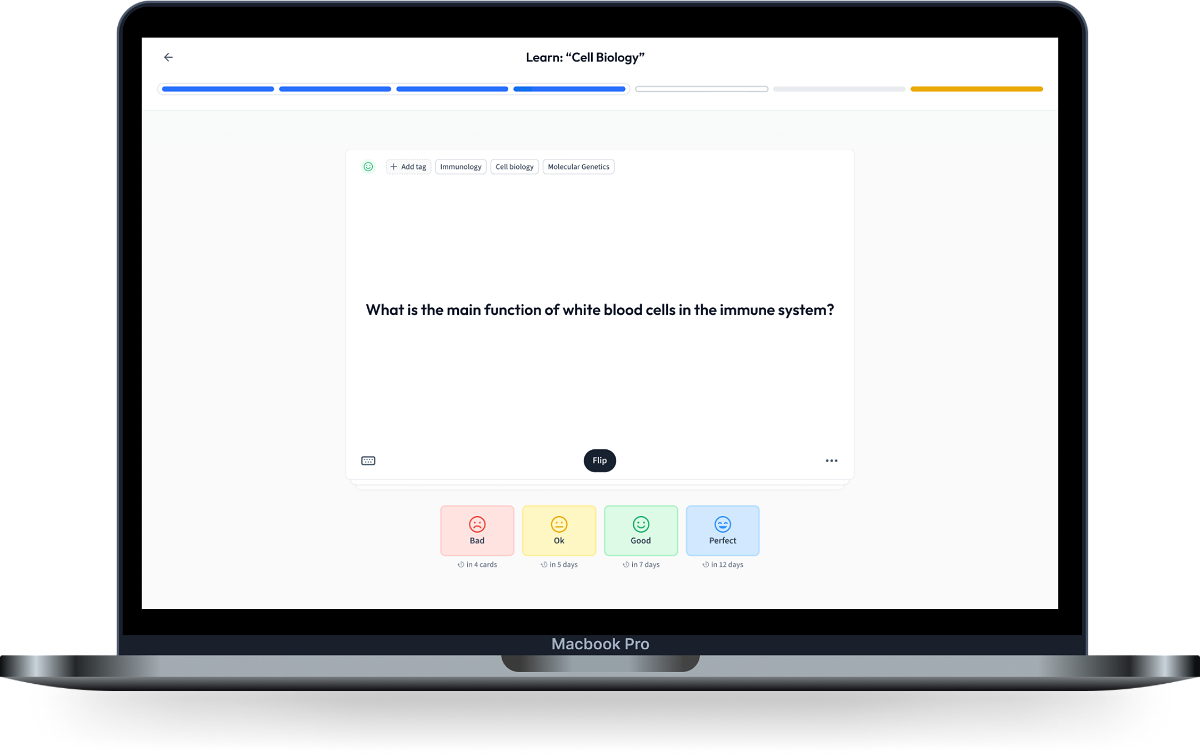
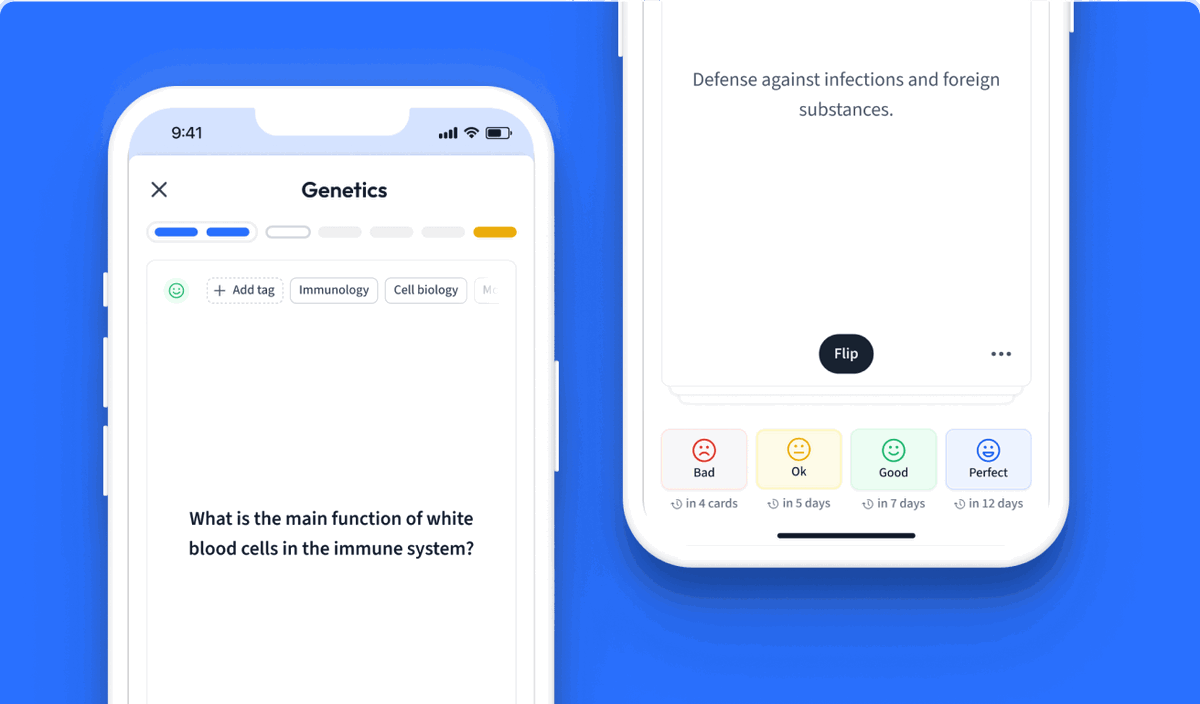
Learn with 10 evidence synthesis flashcards in the free StudySmarter app
Already have an account? Log in
Frequently Asked Questions about evidence synthesis
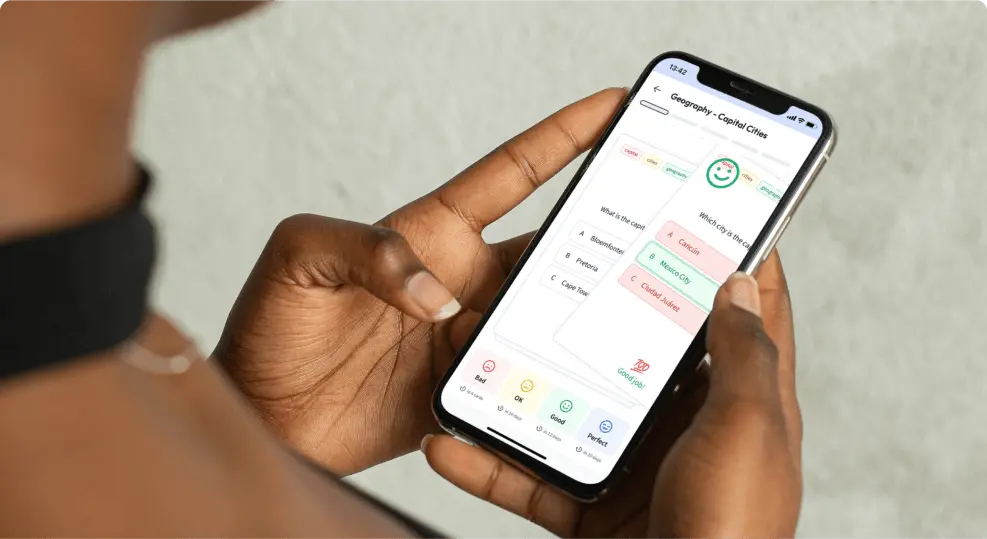
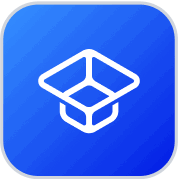
About StudySmarter
StudySmarter is a globally recognized educational technology company, offering a holistic learning platform designed for students of all ages and educational levels. Our platform provides learning support for a wide range of subjects, including STEM, Social Sciences, and Languages and also helps students to successfully master various tests and exams worldwide, such as GCSE, A Level, SAT, ACT, Abitur, and more. We offer an extensive library of learning materials, including interactive flashcards, comprehensive textbook solutions, and detailed explanations. The cutting-edge technology and tools we provide help students create their own learning materials. StudySmarter’s content is not only expert-verified but also regularly updated to ensure accuracy and relevance.
Learn more