Jump to a key chapter
What is Quantitative Analysis in Medicine
Quantitative analysis in medicine refers to the systematic application of mathematical and statistical methods to study and understand various health-related phenomena. This approach provides invaluable insights into medical research, diagnosis, and treatment, enhancing your ability to make informed healthcare decisions.
Understanding Quantitative Analysis
Quantitative analysis essentially involves numerical measurement and the subsequent analysis of data. In medicine, this method allows you to:
- Identify patterns and relationships within health data
- Predict disease outcomes
- Evaluate the effectiveness of treatments
- Support evidence-based decision-making
Quantitative Analysis:
The process of using mathematical and statistical techniques to analyze numerical data, aiming to understand and interpret complex health phenomena.
Mathematical Applications in Medicine
Using mathematics is crucial in quantitative analysis. Critical mathematical models include:
- Linear Regression: Helps predict outcomes based on variable relationships.
- Logistic Regression: Used for binary outcomes like disease presence or absence.
- Survival Analysis: Useful in understanding time-to-event data, like the progression of disease.
Imagine researchers are studying a new drug's effect on blood pressure. They collect systolic blood pressure levels from 100 participants before and after administering the drug. By applying quantitative analysis techniques like a paired t-test, they assess if the drug significantly reduces blood pressure.
Quantitative analysis plays an essential role in personalized medicine. By analyzing genetic data from patients, researchers can tailor treatments based on an individual's unique genetic makeup. Statistical models predict how a patient will respond to specific drugs, optimizing treatment plans for better outcomes. Techniques like genome-wide association studies (GWAS) are used, where large datasets of genetic variants are analyzed to find associations with particular diseases.
Did you know? The application of quantitative analysis extends beyond human medicine. It's also used in veterinary studies, agricultural health, and even environmental health research to assess the impact of pollutants on public health.
Explain Quantitative Analysis Techniques in Medicine
In medicine, the use of quantitative analysis techniques is pivotal for interpreting complex data accurately. Understanding these techniques enables you to make informed decisions that affect patient care and treatment outcomes. Below, we delve into key statistical methods and their applications within medical research and data analysis.
Statistical Methods in Medical Research
Statistical methods form the backbone of quantitative analysis in medical research. They help analyze and interpret data collected from clinical trials and studies to ensure scientific accuracy and validity. Here are a few commonly used methods:
- Descriptive Statistics: Summarizes data points and provides insights into the average, distribution, and variability using means, medians, and standard deviations.
- Inferential Statistics: Allows you to make predictions or inferences about a population based on a sample. Confidence intervals and hypothesis testing are integral components.
- Chi-Square Tests: Tests the association between categorical variables, often used in clinical trials to compare groups.
- ANOVA (Analysis of Variance): Used when comparing three or more groups for statistical differences.
\[\bar{x} = \frac{\text{Sum of all data points}}{\text{Number of data points}}\]
Here, \(\bar{x}\) represents the mean value, a fundamental concept in assessing data distribution.
Ensure your sample size is adequate before applying inferential statistics to increase the reliability of your results.
In a study comparing the efficacy of three different antibiotics, researchers use ANOVA to determine if there are statistically significant differences in effectiveness. They calculate the F-statistic based on the variability between and within treatment groups.
Statistical methods have extended into Bayesian analysis, a powerful technique that incorporates prior knowledge, or previous research findings, into the current analysis. This iterative process provides a dynamic framework for decision-making. For instance, Bayesian statistics can update the probability of a disease presence after considering new medical test results, offering a refined predictive model.
Quantitative Data Analysis Applications
Applications of quantitative data analysis in medicine are vast and varied, ranging from patient data management to public health policy formulation. Here’s a look at some areas where these techniques are commonly applied:
- Clinical Data Management: Assist in organizing, managing, and analyzing patient data for improved healthcare delivery.
- Epidemiology: Analyze the spread of diseases in populations to forecast outbreaks and assess intervention effectiveness.
- Pharmacogenomics: Use genetic data to predict how individual patients will respond to drugs, personalizing medicine.
\[ P(Y=1|X) = \frac{e^{\beta_0 + \beta_1X_1 + \beta_2X_2 + ... + \beta_nX_n}}{1 + e^{\beta_0 + \beta_1X_1 + \beta_2X_2 + ... + \beta_nX_n}} \]
Where \(Y\) is the outcome, \(X_n\) are predictors, and \(\beta_n\) are coefficients found through analysis. This powerful model helps in clinical decision-making by predicting health outcomes.
Application of Quantitative Analysis in Clinical Studies
Quantitative analysis plays a crucial role in clinical studies by providing a framework for analyzing numerical data. This helps in drawing valid conclusions about health-related phenomena. As you navigate through the complexities of clinical data, quantitative analysis tools such as statistical models, charts, and graphs become indispensable in assessing treatment outcomes and identifying trends in patient data.
Benefits of Quantitative Analysis in Healthcare
There are numerous benefits to implementing quantitative analysis in healthcare:
- Precision: Offers accurate and reliable results, minimizing errors in diagnosis and treatment planning.
- Data-Driven Decisions: Supports evidence-based decision-making by providing robust statistical evidence.
- Efficiency: Enhances the speed of data processing and interpretation, especially with large datasets.
- Outcome Prediction: Facilitates the prediction of disease progression and treatment outcomes.
Evidence-Based Decision-Making:
A process that involves making decisions based on the careful integration of the best available evidence, clinical expertise, and patient values.
Quantitative analysis can uncover hidden trends by using data visualization techniques, such as histograms and scatterplots, to make complex data more accessible.
Consider a study evaluating the effectiveness of a flu vaccine. Researchers collect data on incidences of flu in vaccinated versus unvaccinated groups and use logistic regression to determine the vaccine's effectiveness, modeling it with the formula:
\[ P(Y=1|X) = \frac{e^{\beta_0 + \beta_1X}}{1 + e^{\beta_0 + \beta_1X}} \]
Where \(Y\) indicates flu occurrence and \(X\) represents vaccination status. Through this method, the probability of contracting the flu despite vaccination is assessed, guiding future immunization strategies.
Real-world Examples of Quantitative Studies
Quantitative analysis has been applied in numerous real-world clinical studies, offering critical insights and contributing to medical advancements.
Some notable examples include:
- Randomized Controlled Trials (RCTs): Used extensively in testing new medications or treatment protocols. By assigning subjects randomly to either a treatment group or a control group, researchers employ quantitative methods to assess the efficacy of interventions, often analyzing outcomes using chi-square tests or ANOVA.
- Cohort Studies: Observational studies that follow a group of people over time, evaluating the impact of various risk factors on health outcomes. Quantitative analysis in cohort studies often includes survival analysis methods, such as the Cox proportional hazards model, where the hazard function can be expressed as:
\[ h(t) = h_0(t)e^{\beta_1X_1 + \beta_2X_2 + ... + \beta_nX_n} \]
The use of such models helps in understanding how specific variables, like smoking or exercise, affect health over time.
In recent years, quantitative analysis has extended to genomic studies, particularly in the analysis of large-scale genome data. Using techniques such as genome-wide association studies (GWAS), researchers analyze millions of genetic markers to find correlations with diseases. This process incorporates multiple layers of statistical testing to ensure accuracy, often stepping into advanced realms of computation to handle vast amounts of data. Such analyses underpin the growing field of personalized medicine, where treatment is tailored to individual genetic profiles to maximize efficacy and minimize side effects.
Quantitative Analysis Tools and Software in Medicine
Advancements in technology have significantly enhanced quantitative analysis in medicine. Specialized software tools make it easier and more efficient to analyze complex medical data, aiding in research advancements and improved patient outcomes. Explore how these tools are applied in medicine, focusing on commonly used software and the integration of quantitative analysis in medical research.
Common Software Used in Quantitative Analysis
There are various software programs specifically designed to facilitate quantitative analysis in medicine. Each tool offers unique capabilities that help streamline the analysis of medical data.
Some commonly used software include:
- SPSS: Statistical Package for the Social Sciences is widely used for managing and analyzing large datasets. It offers a range of statistical functions for descriptive statistics, hypothesis testing, and data visualization.
- R: An open-source programming language popular among statisticians and data analysts for its comprehensive analytical packages and flexibility in statistical modeling.
- SAS: Statistical Analysis System provides advanced analytics and predictive analytics, making it a preferred choice in epidemiological studies and clinical trials.
- MATLAB: Often used for complex mathematical computations and modeling, especially when linear algebra or advanced mathematical functions are necessary.
A research team working on a large-scale clinical trial might use SAS to manage thousands of patient records, perform intensive logistic regression analyses, and create comprehensive reports on treatment effectiveness. The logistic regression formula used could be:
\[ P(Y=1|X) = \frac{e^{\beta_0 + \beta_1X}}{1 + e^{\beta_0 + \beta_1X}} \]
Where \(Y\) is the outcome (e.g., treatment success) and \(X\) represents various predictors such as age, gender, and baseline health status.
When choosing statistical software, consider the specific requirements of your study, such as the complexity of the analysis and the volume of data.
Integrating Quantitative Analysis in Medical Research
Integrating quantitative analysis in medical research ensures that data-driven decisions support clinical and public health initiatives. This integration is vital for validating study outcomes and formulating evidence-based policies.
Incorporating quantitative analysis involves several steps:
- Data Collection: Utilizing electronic health records and patient databases to gather relevant data.
- Data Cleaning: Ensuring all data are accurate and formatted correctly for analysis.
- Data Analysis: Employing software like SPSS or R to perform statistical tests and models on the collected data.
- Interpretation: Drawing conclusions from the statistical findings and assessing their impact on clinical practice.
The integration of big data analytics with quantitative analysis tools has opened new frontiers in precision medicine. By analyzing large volumes of patient data, researchers can uncover patterns previously undetectable with traditional methods. Machine learning algorithms, for instance, can predict disease outbreaks or responses to treatment by analyzing non-linear relationships in datasets. This computational approach enhances the robustness of quantitative analysis, offering greater predictive capabilities and personalized treatment options.
quantitative analysis - Key takeaways
- Quantitative Analysis: Systematic use of mathematical and statistical methods to study and understand health-related phenomena, aiding informed healthcare decisions.
- Quantitative Analysis Techniques: Involves numerical measurement, allowing for the identification of patterns, prediction of outcomes, and support for decision-making in medicine.
- Statistical Methods in Medical Research: Includes descriptive statistics, inferential statistics, chi-square tests, and ANOVA for analyzing clinical trial data.
- Applications in Clinical Studies: Quantitative analysis provides frameworks for analyzing data, drawing conclusions, and supporting evidence-based healthcare decisions.
- Quantitative Data Analysis: Applied in clinical data management, epidemiology, and pharmacogenomics to improve healthcare delivery and treatment personalization.
- Tools and Software: SPSS, R, SAS, and MATLAB are essential for conducting complex statistical analyses in medical research.
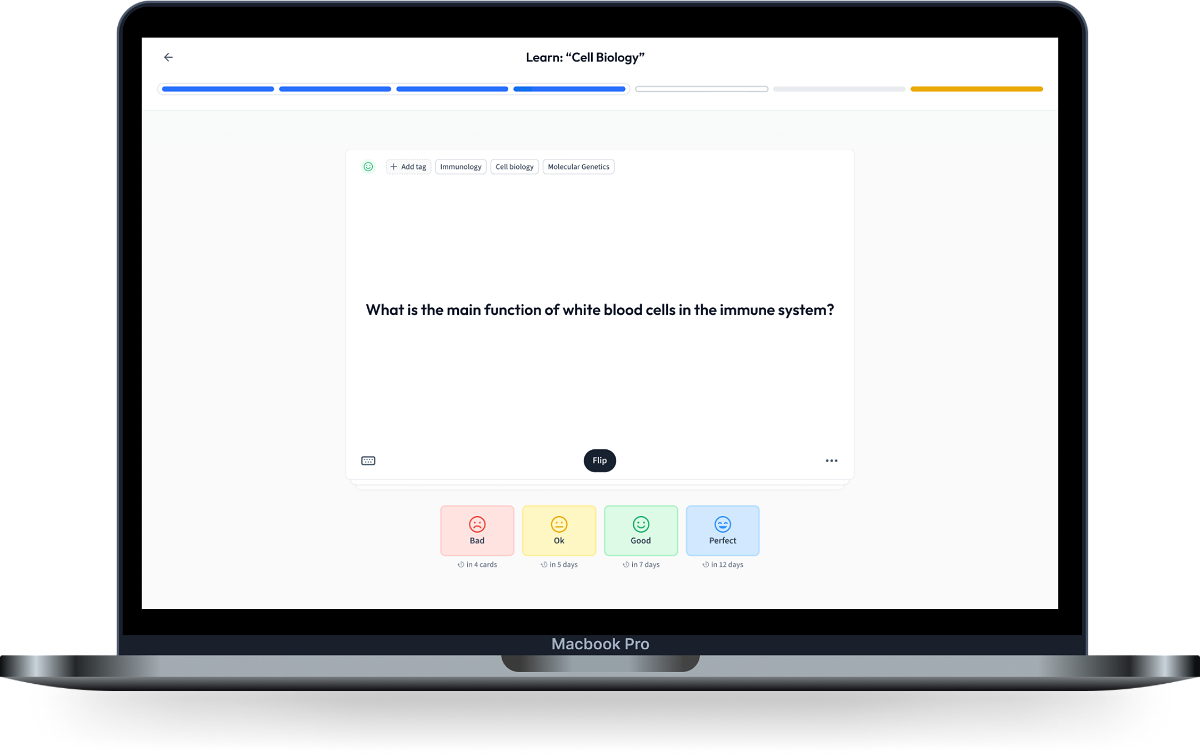
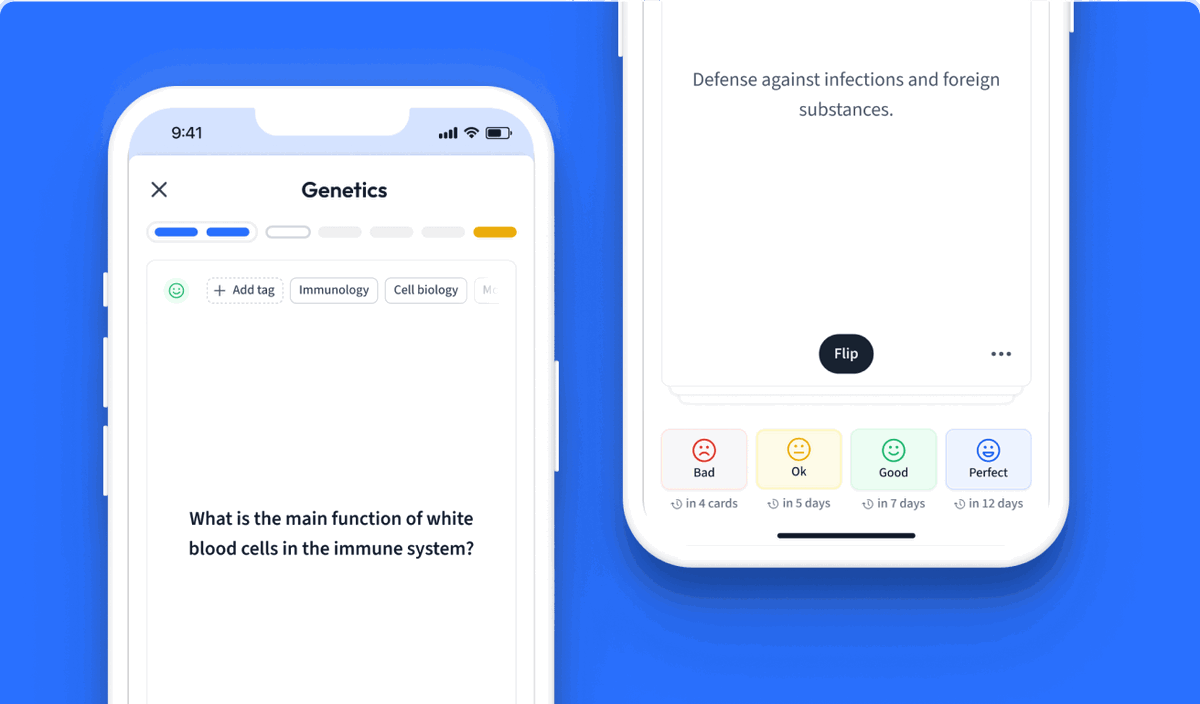
Learn with 12 quantitative analysis flashcards in the free StudySmarter app
Already have an account? Log in
Frequently Asked Questions about quantitative analysis
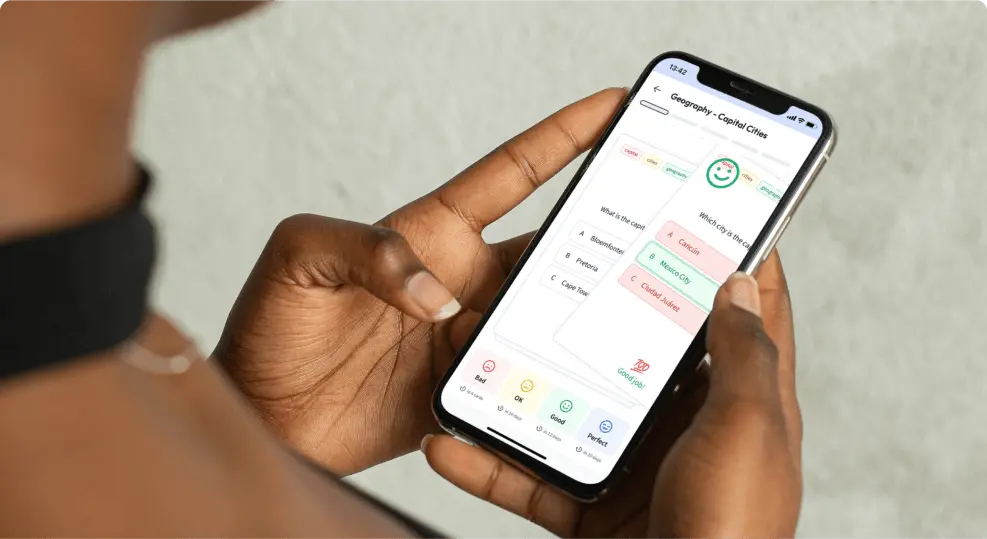
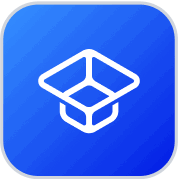
About StudySmarter
StudySmarter is a globally recognized educational technology company, offering a holistic learning platform designed for students of all ages and educational levels. Our platform provides learning support for a wide range of subjects, including STEM, Social Sciences, and Languages and also helps students to successfully master various tests and exams worldwide, such as GCSE, A Level, SAT, ACT, Abitur, and more. We offer an extensive library of learning materials, including interactive flashcards, comprehensive textbook solutions, and detailed explanations. The cutting-edge technology and tools we provide help students create their own learning materials. StudySmarter’s content is not only expert-verified but also regularly updated to ensure accuracy and relevance.
Learn more