Jump to a key chapter
Variance Analysis Definition in Medicine
In medical research, variance analysis plays a critical role in understanding and interpreting data. It is a statistical technique used to identify the sources of variability in a dataset, which helps in assessing the differences between groups. By examining variance, you can determine the significance of these differences in clinical trials, medical efficacy studies, and other healthcare-related researches.
Understanding Variance Analysis in Medical Context
To understand variance analysis within a medical context, it's essential to recognize its function in assessing the reliability and variability of medical data. In practical terms, variance analysis aids in determining whether the observed differences between treatment groups in a study are attributable to the treatment itself or random chance. This technique involves calculating the variance of each group's data and comparing them to a presumed model.
Variance analysis in medicine often involves the ANOVA (Analysis of Variance) technique, which divides the overall variance present in data into contributing factors. For instance, when comparing the effectiveness of different drugs, ANOVA can help you understand how much variance each drug contributes to the overall effectiveness.
When you conduct variance analysis, a significant result implies that at least one group mean is different from others. The mathematical representation of variance analysis might include:
- Between-group variance: Measures how much the sample means differ.
- Within-group variance: Measures the variability within each group.
Mathematically, you express total variance (\text{Total Variance}) as follows:
\[\text{Total Variance} = \text{Between-group Variance} + \text{Within-group Variance}\]
The precise calculation of variance requires knowledge of standard deviation, which is the square root of the variance.
Statistical Concepts Behind Variance Analysis
Completing a variance analysis requires a solid foundation in several statistical concepts. A vital component involves understanding the null hypothesis and alternative hypothesis. In variance analysis, the null hypothesis usually posits that there are no differences among group means, while the alternative suggests that differences do exist.
Another critical concept is F-distribution, which is used to ascertain the significance of the variance analysis. In practice, the F-statistic is computed, comparing the ratio of between-group variance to within-group variance:
\[F = \frac{\text{Between-group Variance}}{\text{Within-group Variance}}\]
If the calculated F-value is greater than the critical value derived from F-distribution tables at a specific significance level, you reject the null hypothesis, indicating significant differences between group means.
Variance analysis also makes frequent use of computational techniques like mean squares (MS). The mean square of a factor quantifies its contribution to the total variance and is calculated as:
\[\text{MS} = \frac{\text{Sum of Squares}}{\text{Degrees of Freedom}}\]
Understanding these statistical concepts ensures that the variance analysis is both accurate and meaningful for medical research findings.
Variance Analysis Example in Medical Research
When conducting medical research, the use of variance analysis is instrumental in dissecting the variability within your data sets. This statistical tool helps determine whether observed differences are significant or have occurred by chance.
Real-life Applications of Variance Analysis
In real-life medical scenarios, variance analysis is applicable in numerous contexts. For example, researchers often apply it to compare the effectiveness of different treatment regimes. Consider a study investigating the impact of three types of drugs on blood pressure levels.
Suppose the purpose is to determine whether Drug A, Drug B, or Drug C results in the most significant reduction in blood pressure. ANOVA can be employed to analyze the variance among the treatment groups. This process will involve:
- Calculating the mean blood pressure reduction for each group.
- Computing between-group and within-group variances.
- Deriving the F-statistic to test group differences.
The formula to compute the F-statistic is as follows:
\[ F = \frac{\text{MS}_{\text{between}}}{\text{MS}_{\text{within}}} \]
This analysis allows you to determine if the differences between treatments are statistically significant, guiding clinicians in making informed decisions about which treatment to recommend.
Treatment Group | Mean Blood Pressure Reduction |
Drug A | 3.5 mmHg |
Drug B | 4.2 mmHg |
Drug C | 5.0 mmHg |
For instance, in a clinical trial comparing three heart disease medications, variance analysis could reveal that Medication X reduces cholesterol significantly more compared to Medication Y and Z, assisting healthcare professionals in choosing the optimal treatment.
Remember, conducting variance analysis requires careful consideration of assumptions like normality, homogeneity of variances, and independent samples.
Case Studies: Implementing Variance Analysis in Medicine
Variance analysis has been effectively implemented in various medical case studies to extract meaningful insights from complex data. It proves essential in clinical trials where researchers aim to compare multiple treatment effects.
Take, for example, a case study examining the effects of dietary interventions on diabetic patients’ blood glucose levels. Researchers divide subjects into three diet groups: low-carb, Mediterranean, and the traditional diabetic diet. Variance analysis could reveal which diet produces the most significant blood glucose control over six months.
Here's how it works:
- Data collection includes monthly blood glucose readings.
- Variance analysis calculates the effect of each diet.
- MS values differentiate diets' impacts.
The collected data table might look like this:
Diet Group | Avg. Blood Glucose Improvement |
Low-Carb | 15% |
Mediterranean | 12% |
Traditional | 8% |
If the analysis yields a significant F-statistic, it may suggest the low-carb diet having a superior effect on blood glucose levels as compared to others.
In-depth exploration of variance analysis in healthcare reveals its potential beyond traditional treatment comparisons. Innovative applications include genomics research, where it helps identify gene expressions linked to disease vulnerabilities. Variance analysis enables researchers to manage substantial variabilities in genetic data, offering insights that pave the way for personalized medicine. Furthermore, this approach is advancing the effectiveness of public health surveillance, tracking health trends in populations based on environmental and socio-economic variables, thus facilitating targeted interventions.
Repeated Measures Analysis of Variance
Understanding Repeated Measures Analysis of Variance (RM ANOVA) is essential in medical research when analyzing data collected from the same subjects across multiple conditions or time points. It provides statistical insights into both inter-subject effects and intra-subject effects, making it an invaluable tool for analyzing longitudinal study data and crossover trials in the healthcare sector.
Purpose and Functionality in Medical Studies
The primary purpose of using Repeated Measures ANOVA in medical studies is to assess how a treatment affects subjects over time. This technique helps determine if observed changes in patient outcomes are statistically significant, potential sources of time-related variation, and overall treatment efficacy.
RM ANOVA is beneficial in numerous clinical research scenarios, such as:
- Tracking the progression of a disease under different treatment conditions.
- Evaluating the impact of behavioral interventions on mental health over several weeks.
- Examining biochemical changes in patients following surgical procedures.
In these studies, RM ANOVA helps you answer critical questions like whether a treatment effect is consistent across different time points, or if there are interaction effects between time and treatment.
The mathematical representation for RM ANOVA involves partitioning variance into three components:
- Subject variance: Accounts for the individual differences.
- Treatment variance: Reflects the effect of the independent variables.
- Residual variance: Represents the unexplained difference.
RM ANOVA: A statistical technique used to evaluate the significance of mean differences across multiple time points or conditions within the same participants.
Advanced applications of RM ANOVA in pharmacological studies have revolutionized drug efficacy testing. Researchers now utilize mixed models with repeated measures to accommodate incomplete data and account for covariance structures. Furthermore, RM ANOVA is being integrated with machine learning algorithms to analyze complex datasets in predictive personalized medicine, uncovering trends that may not be evident with traditional analyses.
Step-by-Step Guide for Students
Here's a simplified guide to performing Repeated Measures ANOVA for beginners:
- Select the Appropriate Data: Ensure your data meets criteria for RM ANOVA, such as normal distribution, sphericity, and equality of variances.
- State Hypotheses: Formulate a null hypothesis that assumes no differences across time points, and an alternative hypothesis suggesting significant differences exist.
- Calculate ANOVA: Split the total variance into variance due to repetition and variance due to subjects:
\[\text{Total Variance} = \text{Time Variance} + \text{Subject Variance} + \text{Error Variance}\]
- Conduct Post-hoc Tests: If ANOVA results are significant, conduct post-hoc comparisons to pinpoint which time points significantly differ from one another.
- Interpret Results: Evaluate the F-statistic and p-values to confirm statistical significance.
Consider a study analyzing the effect of a new diet on cholesterol levels over three months, with measurements taken monthly. RM ANOVA allows you to assess whether the diet produces a consistent cholesterol reduction over time.
Remember, RM ANOVA assumes that variances of the differences between all combinations of related groups (sphericity) are equal. Violations need corrections like Greenhouse-Geisser adjustments.
Importance of Variance Analysis in Medical Studies
In the realm of medical research, understanding the importance of variance analysis is essential for accurate data interpretation and decision-making. This statistical method helps you determine the sources of variability within your data set, which is crucial for evaluating the quality, efficacy, and safety of medical interventions.
Impact on Medical Research Outcomes
Variance analysis has a profound impact on medical research outcomes, allowing you to differentiate between meaningful treatment effects and random variation. By decomposing total variation into components associated with different sources, this technique assists in identifying the actual impact of treatments.
Here are key ways variance analysis influences research outcomes:
- Helps verify the statistical significance of differences among study groups.
- Enhances the reliability of clinical trials by minimizing error variance.
- Identifies sources of bias, leading to improved study designs.
In medical trials, a typical model used is the analysis of variance (ANOVA), which partitions the observed variance to understand the effectiveness of different treatments or interventions. The formula often used for ANOVA is:
\[ F = \frac{\text{Variance due to Treatment}}{\text{Variance due to Error}} \]
An F-ratio higher than the critical value rejects the null hypothesis, indicating a significant difference in treatment effects.
In a study comparing aspirin's effects on preventing heart attacks, variance analysis could demonstrate that aspirin significantly lowers heart attack incidence compared to a placebo.
Always ensure that your data meets the assumptions of ANOVA, such as normality, independence, and homogeneity of variance, to maintain the integrity of your results.
How Variance Analysis Influences Medical Conclusions
Variance analysis plays a crucial role in shaping medical conclusions. It provides a structured framework for interpreting complex data sets, helping you confirm or refute hypotheses based on a robust statistical basis.
Here's how variance analysis influences conclusions:
- Improving Accuracy: By reducing bias and error, variance analysis aids in drawing more precise conclusions.
- Determining Effect Size: It helps evaluate the magnitude of treatment effects, essential for clinical relevance.
- Explaining Variation: By analyzing different sources of variance, researchers can provide detailed explanations for observed effects.
In practice, interpreting variance can be visualized as a table partitioning variance sources as shown below:
Source | Degrees of Freedom | Sum of Squares |
Treatment | - 1 | SS_{treatment} |
Error | k(n-1) | SS_{error} |
Overall, variance analysis provides invaluable insights that enhance the reliability and validity of your medical research findings.
In the advancement of public health policies, variance analysis transcends its traditional use by aiding policymakers in understanding disease spread trends, vaccination impacts, and health behavior efficacy. It is integral in designing targeted interventions based on demographic data, allowing for more precise and effective health campaigns. Future applications may involve integration with AI to predict healthcare needs based on variance trends found in genomic data or electronic health records.
Kruskal Wallis Analysis of Variance
The Kruskal Wallis test is an essential tool in the analysis of variance, particularly for non-parametric data in medical research. It extends the capabilities of the Mann-Whitney U test for comparing more than two groups. This test is useful when the assumptions necessary for ANOVA are not met, such as normality and homoscedasticity of variances.
Non-parametric Approach in Medical Studies
In medical studies, data often do not meet the assumptions required for parametric tests, making the non-parametric Kruskal Wallis test a valuable alternative. Unlike its parametric counterpart, ANOVA, the Kruskal Wallis test does not assume that the data are normally distributed. Instead, it ranks the data and tests for differences in the median ranks between groups.
Here's how the Kruskal Wallis test is applied:
- Rank All Observations: Combine and rank all data points from the different groups.
- Compute Rank Sums: Calculate the sum of ranks for each group.
- Calculate the Test Statistic: Use the formula
\[H = \frac{12}{N(N+1)} \sum \frac{R_i^2}{n_i} - 3(N+1)\]
- Determine Significance: Compare the H statistic against a Chi-square distribution with k-1 degrees of freedom, where k is the number of groups.
This method proves particularly useful in clinical trials and epidemiological studies where data may be ordinal or not adhere to normal distribution.
The Kruskal Wallis test is especially helpful when dealing with small sample sizes where normal distribution cannot be assumed.
When to Use Kruskal Wallis in Medicine
In the medical field, recognizing when to apply the Kruskal Wallis test is crucial for obtaining valid results. It's particularly applicable in scenarios where you cannot satisfy the parametric test assumptions.
Consider using the Kruskal Wallis test when:
- The sample sizes are unequal and assumptions of homogeneity of variances are violated.
- The data are ordinal or contain outliers that could distort the results of parametric tests.
- You need to analyze categorical data at an ordinal level, such as pain scores or quality of life ratings across different treatment groups.
As an example, imagine a study comparing three new treatments for reducing anxiety levels among patients:
Treatment Group | Rank Sum of Anxiety Scores |
Treatment A | 65.5 |
Treatment B | 78.0 |
Treatment C | 53.0 |
You can employ the Kruskal Wallis test to evaluate if these rank sums differ significantly, indicating that at least one treatment effect diverges from the others.
Variance Analysis Explained for Students
Variance analysis is a cornerstone of statistical methods, widely used in fields such as medicine to understand data variability. This powerful tool helps you dissect and comprehend complex datasets by identifying the sources of differences within them.
Simplifying Complex Concepts for Better Understanding
Variance analysis may seem daunting at first, but breaking down its components simplifies the concept. Essentially, it involves understanding how data points differ from the mean and each other, uncovering hidden patterns or significant anomalies.
The key elements in variance analysis include:
- Standard Deviation: Measures the amount of variation or dispersion in a set of values.
- Analysis of Variance (ANOVA): Used to compare multiple group means to see if at least one is significantly different.
- F-statistic: A ratio used in ANOVA tests to determine if the variability between group means is larger than the variability within the groups.
For instance, ANOVA's equation is:
\[ F = \frac{\text{Mean Square Between Groups}}{\text{Mean Square Within Groups}} \]
When interpreted accurately, these components reveal how different factors contribute to overall variability, playing a significant role in decision-making processes in disciplines like medicine.
Variance: A statistical measurement of the spread between numbers in a data set; it measures how far each number in the set is from the mean and thus from every other number in the set.
Variance analysis isn't limited to just understanding data spread. It's also pivotal in hypothesis testing, reliability checks, and experimental design in the medical field. By utilizing detailed variance breakdowns, researchers can delineate between actual treatment effects and random noise, ensuring that conclusions drawn are not only statistically significant but clinically relevant.
Tips and Tricks for Mastering Variance Analysis
Mastering variance analysis requires practice and a good grasp of statistical principles. To aid in your understanding, we've outlined several tips and tricks:
- Visualize Data: Use graphs and charts to identify patterns or outliers.
- Understand Assumptions: Before applying ANOVA, ensure assumptions like normality and homogeneity of variances are not violated.
- Utilize Software: Statistical software can simplify complex calculations and reduce human error.
- Practice Interpretation: Regularly work on interpreting results as many times the numbers alone don’t tell the full story.
Understanding the formula for total variance will further reinforce your skills:
\[\text{Total Variance} = \text{Explained Variance} + \text{Unexplained Variance}\]
This indicates that variance is closely tied to factors both known (explained) and unknown (unexplained), equipping you with a foundational understanding to approach more intricate statistical challenges.
For instance, if you are analyzing the effect of three different drugs on blood pressure, variance analysis can help identify which drug most effectively lowers blood pressure with statistical significance.
When interpreting variance results, remember that a larger F-statistic value often implies a significant difference among group means, guiding you to delve deeper into the factors causing these differences.
variance analysis - Key takeaways
- Variance Analysis Definition in Medicine: A statistical technique to identify variability sources in datasets, essential for assessing group differences in medical studies.
- Analysis of Variance (ANOVA): A method dividing variance into components to understand group differences in medical research, significant for comparing treatments.
- Repeated Measures ANOVA: Used for analyzing data across multiple conditions/times within the same subjects, crucial for longitudinal medical research.
- Importance of Variance Analysis: Essential for accurate data interpretation in medical research, differentiating meaningful treatment effects from random variation.
- Kruskal Wallis Analysis of Variance: A non-parametric test for comparing more than two groups, useful when ANOVA assumptions are unmet.
- Variance Analysis Explained for Students: Involves breaking down data variability, explained using elements like standard deviation and ANOVA.
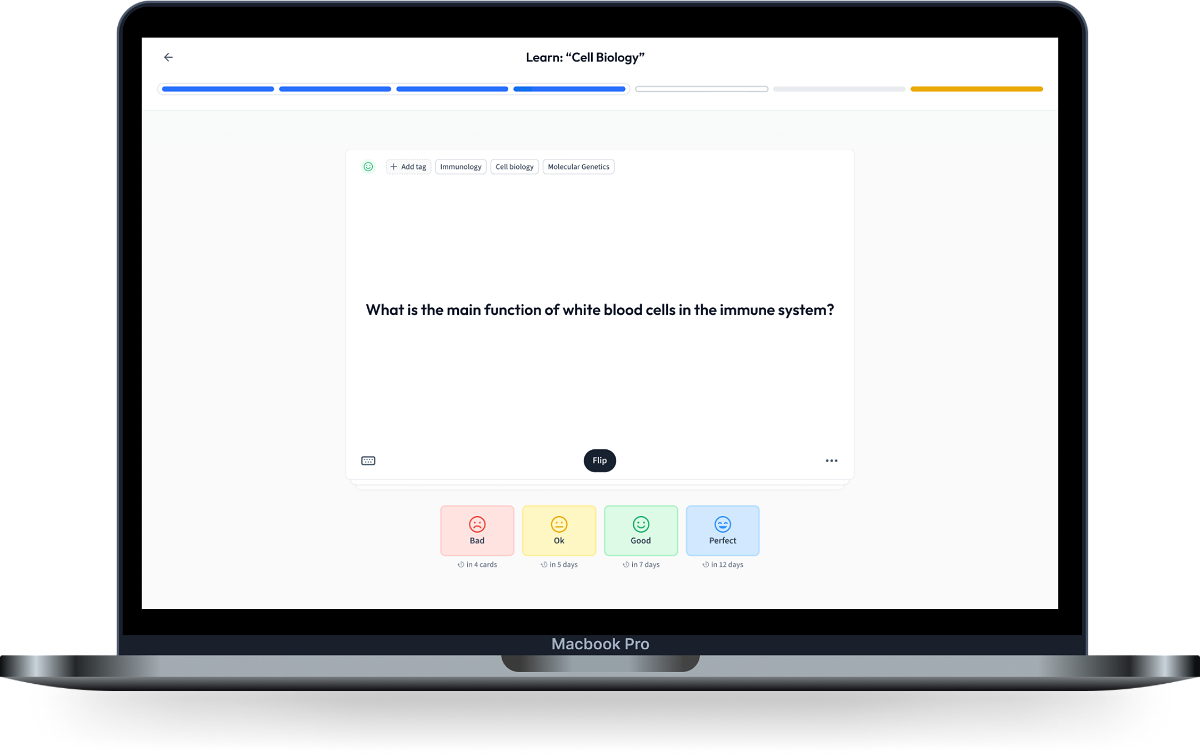
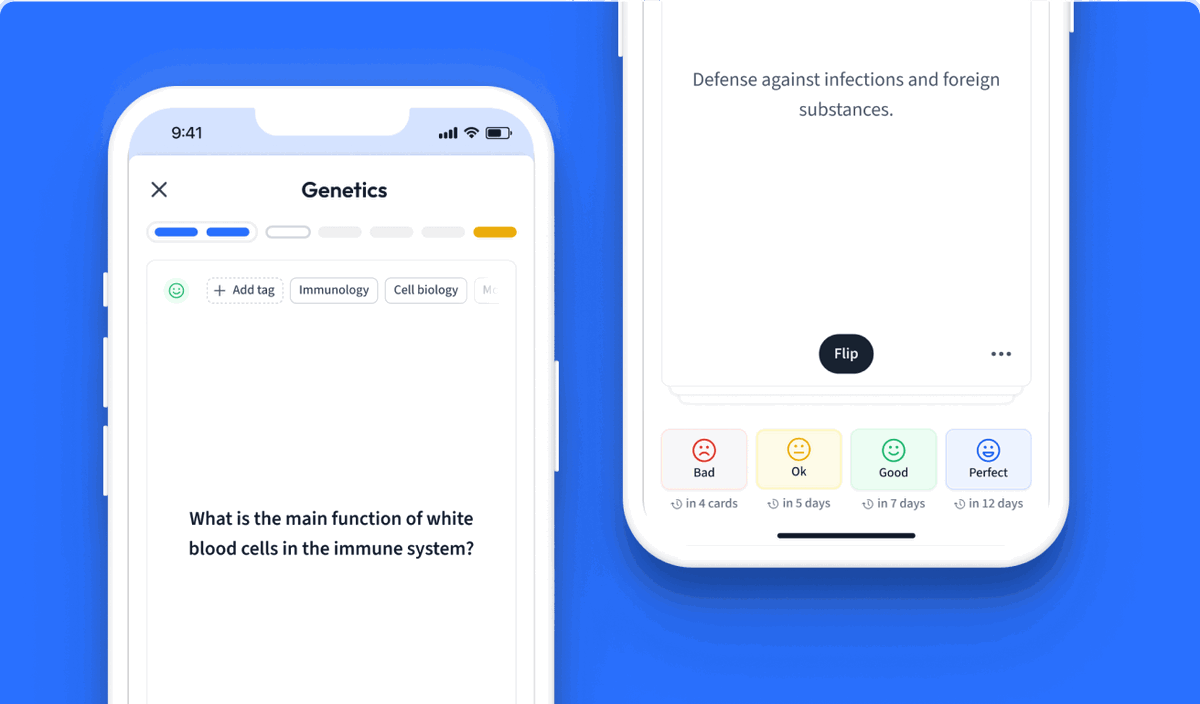
Learn with 12 variance analysis flashcards in the free StudySmarter app
We have 14,000 flashcards about Dynamic Landscapes.
Already have an account? Log in
Frequently Asked Questions about variance analysis
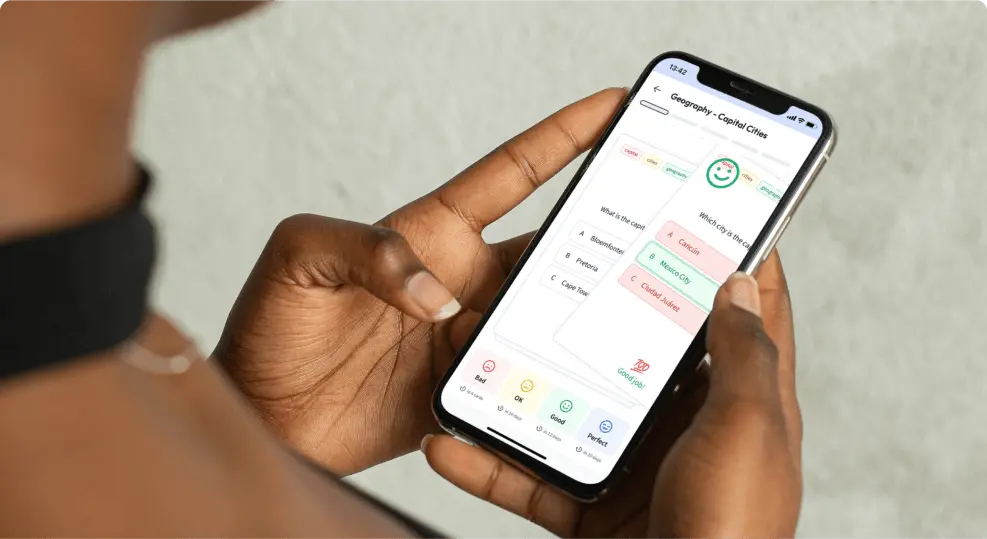
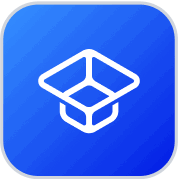
About StudySmarter
StudySmarter is a globally recognized educational technology company, offering a holistic learning platform designed for students of all ages and educational levels. Our platform provides learning support for a wide range of subjects, including STEM, Social Sciences, and Languages and also helps students to successfully master various tests and exams worldwide, such as GCSE, A Level, SAT, ACT, Abitur, and more. We offer an extensive library of learning materials, including interactive flashcards, comprehensive textbook solutions, and detailed explanations. The cutting-edge technology and tools we provide help students create their own learning materials. StudySmarter’s content is not only expert-verified but also regularly updated to ensure accuracy and relevance.
Learn more