Jump to a key chapter
Introduction to Epidemiological Data Analysis
Epidemiological data analysis plays a vital role in understanding and controlling health-related events. It forms the basis for policy development, ensuring informed decisions. In this article, you'll explore the types of data involved and how epidemiological studies are designed.
Types of Epidemiological Data
Epidemiological data can be classified into various types. Recognizing these helps in choosing appropriate analysis methods:
- Descriptive Data: This data provides information about the occurrence and frequency of diseases within a population. Examples include case reports and surveillance data.
- Analytical Data: You use this data to determine the causes and risk factors of diseases, often collected through case-control or cohort studies.
- Experimental Data: This involves data from controlled experiments like clinical trials.
Through categorizing, you'll better understand how to handle epidemiological data efficiently.
Epidemiological Data Analysis is the process of systematically applying statistical and analytical techniques to data collected during research studies, aiming to understand health patterns and discover causal relationships.
An important component of epidemiological data analysis is the mathematical modeling of disease transmission. For example, calculating the basic reproduction number, denoted as R₀, is crucial. The formula for \(R_0\) is: \[ R_0 = \frac{c \times p \times d}{k} \] where: - \(c\) is the number of contacts per unit time, - \(p\) the transmission probability per contact, - \(d\) the duration of infectiousness, - \(k\) the rate of recovery.This calculation helps in estimating the level of immunization needed to prevent epidemic spread.
Design of Epidemiological Studies
Designing a epidemiological study entails selecting the methodology best suited to answer research questions. There are several types to consider:
- Cross-sectional Studies: These studies assess both exposure and outcome within a particular timeframe. They do not establish causality but provide data on prevalence.
- Cohort Studies: Following a group over time, these studies offer insights into how certain factors affect outcomes. Potential biases like selection bias should be minimized.
- Case-control Studies: You examine past exposures in relation to current outcomes, usually estimating risk through odds ratios \(\left( OR = \frac{odds \ of \ event \ in \ exposed}{odds \ of \ event \ in \ non-exposed} \right)\).
- Randomized Controlled Trials (RCTs): Considered the gold standard, RCTs require random assignment to treatment or placebo groups to identify cause-effect relationships.
Each design has strengths and limitations, highlighting the need for careful planning to ensure valid and reliable results.
Example: Imagine conducting a cohort study to investigate if extended screen time impacts childhood obesity. You'd track a large group over time, documenting screen time habits and changes in body mass index (BMI), establishing links between variables.
Remember, in any epidemiological study, confounding variables can obscure the true relationship between exposure and outcome. Strategies like stratification and multivariate analysis are key in managing these variables.
Epidemiological Data Analysis Methods
Epidemiological data analysis methods are essential for extracting meaningful insights from health data. By applying appropriate techniques, you can identify trends and make informed decisions regarding public health strategies.
Statistical Techniques in Epidemiology
In epidemiology, statistical techniques are used to determine relationships between variables and predict potential outcomes. Here are some key methods:
- Descriptive Statistics: Used to summarize data and include measures such as mean, median, and mode. For instance, the average age of participants in a study.
- Probability: Determines the likelihood of an event, essential for risk assessment and confidence intervals.
- Regression Analysis: Helps in understanding relationships between variables, especially through linear regression, expressed as \(Y = \beta_0 + \beta_1X\), where \(Y\) is the outcome and \(\beta_1\) the coefficient.
- Survival Analysis: Focuses on the time until an event occurs, useful in analyzing treatment efficacy.
These techniques form the backbone of epidemiological research, facilitating robust data interpretation.
Example: In a study focusing on the correlation between air pollution and respiratory conditions, multiple regression analysis could be employed. Using the equation \(Y = \beta_0 + \beta_1X_1 + \beta_2X_2 + ... + \beta_nX_n\), you could examine how various pollutants (\(X_1, X_2, \ldots \)) affect health outcomes (\(Y\)).
Consider using software like R or Python for complex data analysis. They offer libraries like statsmodels
and pandas
for efficient statistical computations.
Interpretation of Epidemiological Data
Interpreting epidemiological data involves understanding the meaning of analysis results. This process has several steps:
- Assessing Validity: Examining if the study accurately measures what it sets out to measure, which includes evaluating bias and confounding factors.
- Generalizability: Determining if results can be applied to other populations beyond the sample.
- Statistical Significance: Checking if findings show a genuine effect rather than random chance, often using a p-value. For instance, a p-value < 0.05 indicates significance.
- Clinical Relevance: Assessing whether statistical findings have practical health implications.
These interpretation steps help convey research findings in a manner that policymakers and the public can understand.
Epidemiological Modeling often employs mathematical tools to forecast disease spread. A common model is the Susceptible-Infectious-Recovered (SIR) model, represented by differential equations: \[ \frac{dS}{dt} = -\beta SI \] \[ \frac{dI}{dt} = \beta SI - \gamma I \] \[ \frac{dR}{dt} = \gamma I \] Here, \(S, I,\) and \(R\) denote the fractions of susceptible, infectious, and recovered individuals, respectively, while \(\beta\) is the infection rate and \(\gamma\) the recovery rate.
Epidemiological Data Analysis Examples
Epidemiological data analysis is fundamental in understanding disease patterns and making informed public health decisions. By examining case studies and recognizing common analysis pitfalls, you will become adept at interpreting epidemiological data effectively.
Case Studies on Epidemiological Data Analysis
Case studies provide valuable insights into the application of epidemiological data analysis. Each study highlights different methodologies and outcomes:
- COVID-19 Pandemic Analysis: A model using SIR equations helped predict the spread and required interventions. The basic SIR model is given by:
- \(\frac{dS}{dt} = -\beta SI\)
- \(\frac{dI}{dt} = \beta SI - \gamma I\)
- \(\frac{dR}{dt} = \gamma I\)
- Smoking and Lung Cancer: A case-control study concluded that smoking significantly increased lung cancer risk. Odds ratios (OR) were calculated, showing: \[ OR = \frac{odds \ of \ cancer \ in \ smokers}{odds \ of \ cancer \ in \ non-smokers} \]
- Cholera Outbreak in Haiti: Analytical data identified contaminated water sources as a primary transmission vector, helping mitigate the outbreak.
These examples illustrate different approaches in analyzing epidemiological data to address specific health issues.
Example: In the 1854 cholera outbreak in London, John Snow used a dot map to identify water sources as the transmission route. This method was pivotal in developing modern epidemiological mapping techniques.
Remember, visual aids like maps and graphs are crucial in presenting epidemiological data clearly.
Common Pitfalls in Analyzing Epidemiological Data
Analyzing epidemiological data involves complexities that can introduce errors. Avoid common pitfalls with careful consideration:
- Confounding Variables: They can obscure true associations. Strategies like stratification can adjust for potential confounders.
- Bias: Sampling or measurement biases can distort findings. Ensuring random sampling and reliable measurement tools is vital.
- Multiple Testing: Increases false-positive results. Use Bonferroni correction or similar methods to reduce error rates.
Being aware of these pitfalls enhances the accuracy and reliability of epidemiological studies.
Mathematical and statistical modeling forms an integral part of overcoming these challenges:
- Bayesian Analysis: This approach incorporates prior knowledge in data analysis, updating probabilities as new data emerges. It is useful in handling missing data or integrating expert opinions.
- Machine Learning Techniques: Algorithms such as Random Forest and Support Vector Machines (SVM) help in predictive modeling, especially when handling large datasets.
These advanced techniques can refine disease prediction models and enhance overall study insights.
Regular updates and collaboration with interdisciplinary teams can mitigate analysis challenges.
Future Trends in Epidemiological Data Analysis
In the evolving field of epidemiology, future trends in data analysis are driven by technological advancements and novel methodologies. You'll see how data analysis in epidemiology is transforming to meet global health challenges.
Emerging Data Analysis Methods
New data analysis methods are reshaping how we approach epidemiological data. These novel techniques enhance our ability to predict, monitor, and respond to health issues.
- Machine Learning: Algorithms that process vast datasets to uncover patterns and make predictions without explicit programming.
- Artificial Intelligence (AI): Sophisticated systems that enhance decision-making and can identify non-obvious relationships in data.
- Spatial Analysis: Strengthened by Geographic Information Systems (GIS), this method visualizes data geographically, revealing patterns such as disease spread within regions.
These methods replace traditional analysis, offering greater accuracy and contextual insights. For example, AI models can predict outbreaks by analyzing social media trends combined with health data.
Example: A research team used machine learning to analyze influenza patterns, employing a model of \[ f(x) = x_1 + x_2^2 + e^{-x_3} \] to predict the peak season accurately, incorporating variables like temperature and humidity.
Machine learning requires large amounts of data; always ensure data quality and completeness to improve model performance.
DeepDive: With the rise of big data, epidemiologists are leveraging cloud computing to process and store vast datasets. This capacity enables real-time data processing and collaboration across international boundaries.
- Data Integration: Combined data from multiple sources allows for comprehensive modeling.
- Predictive Analytics: Uses past data to forecast future trends, crucial in preventing outbreaks.
The Role of Technology in Epidemiological Studies
Technology is transforming epidemiological studies, making data collection and analysis more efficient and accurate.
- Wearable Devices: Devices like smartwatches now monitor health indicators in real-time, providing continuous streams of patient data.
- Health Informatics: Systems that gather patient records digitally enhance the ability to track public health data seamlessly.
- Telemedicine: Improves access to healthcare services, especially in remote locations.
These technologies not only streamline data collection but also enable predictive analytics, improving epidemic preparedness and response.
Example: During the Ebola outbreak, health informatics systems were pivotal. Algorithms modeled transmission dynamics using the equation \( R_0 = \frac{c \times p \times d}{k} \) where \( R_0 \) is the basic reproduction number, aiding in deploying resources efficiently.
Always consider privacy and data protection, especially when using technology to collect health data.
epidemiological data analysis - Key takeaways
- Epidemiological Data Analysis: Involves systematically applying statistical and analytical techniques to epidemiological data to understand health patterns and causal relationships.
- Types of Epidemiological Data: Includes descriptive data (e.g., case reports), analytical data (e.g., data from cohort and case-control studies), and experimental data (e.g., clinical trials).
- Design of Epidemiological Studies: Includes cross-sectional, cohort, case-control studies, and randomized controlled trials, each with unique strengths and methodological approaches.
- Statistical Techniques in Epidemiology: Descriptive statistics, probability, regression analysis, and survival analysis are key methods to explore relationships and predict outcomes.
- Interpretation of Epidemiological Data: Involves assessing validity, generalizability, and significance of findings, ensuring clear communication for public health decisions.
- Epidemiological Data Analysis Examples: Case studies like COVID-19 spread using SIR models, smoking-related lung cancer risk, and the cholera outbreak demonstrate practical applications of data analysis.
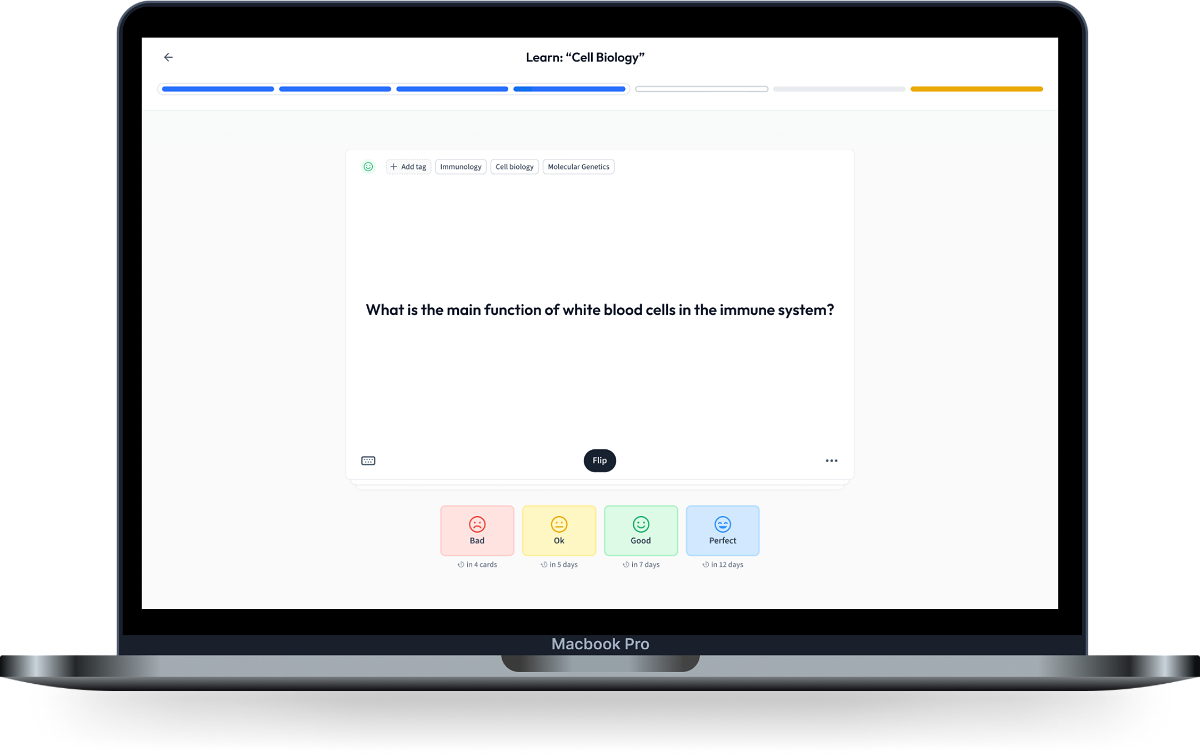
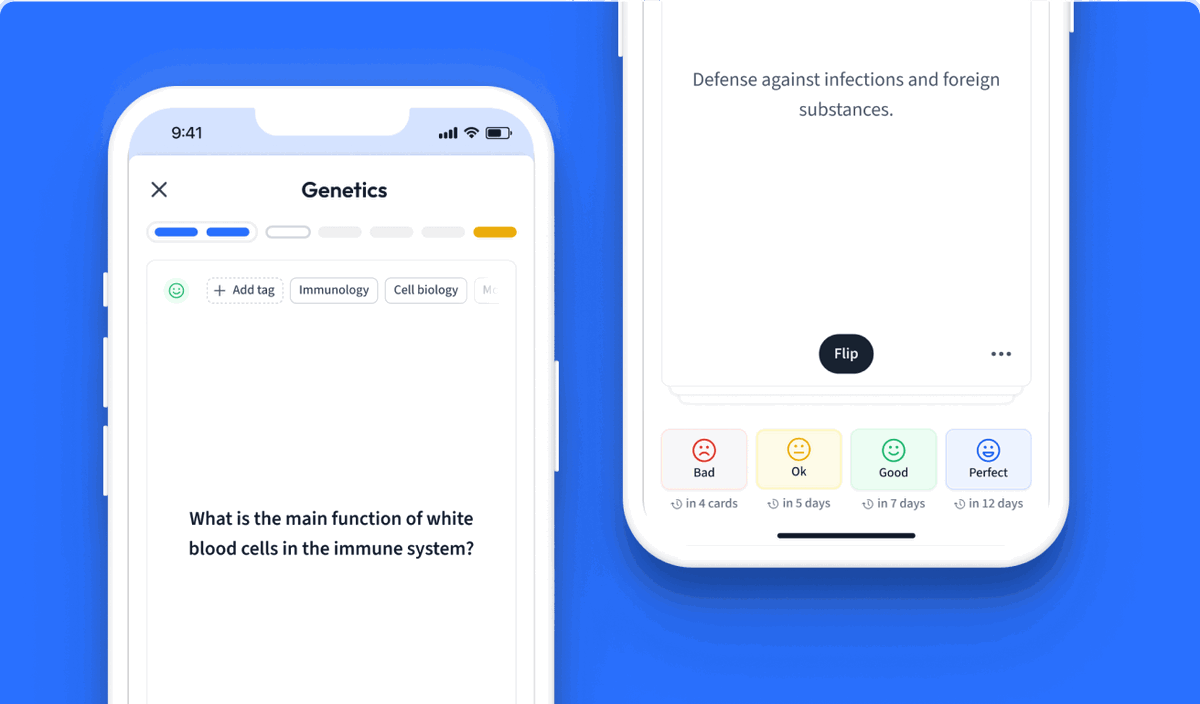
Learn with 12 epidemiological data analysis flashcards in the free StudySmarter app
We have 14,000 flashcards about Dynamic Landscapes.
Already have an account? Log in
Frequently Asked Questions about epidemiological data analysis
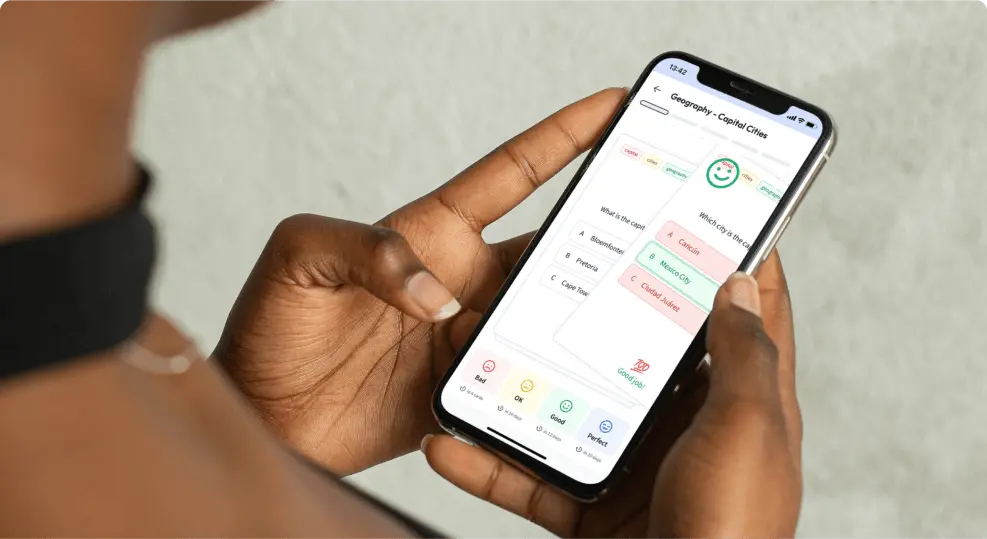
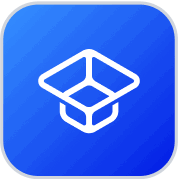
About StudySmarter
StudySmarter is a globally recognized educational technology company, offering a holistic learning platform designed for students of all ages and educational levels. Our platform provides learning support for a wide range of subjects, including STEM, Social Sciences, and Languages and also helps students to successfully master various tests and exams worldwide, such as GCSE, A Level, SAT, ACT, Abitur, and more. We offer an extensive library of learning materials, including interactive flashcards, comprehensive textbook solutions, and detailed explanations. The cutting-edge technology and tools we provide help students create their own learning materials. StudySmarter’s content is not only expert-verified but also regularly updated to ensure accuracy and relevance.
Learn more