Jump to a key chapter
Quantitative Methods Definition
Quantitative methods involve the use of numerical data and mathematical models to analyze and interpret phenomena. By using statistics, researchers and medical professionals can extract meaningful patterns and predictions from complex datasets.
A quantitative method is a research technique that outputs data in numerical form, enabling precise measurements, evaluations, and analyses across different domains, such as medicine.
Significance of Quantitative Methods in Medicine
In the medical field, quantitative methods are crucial because they allow healthcare practitioners to:
- Diagnose diseases by interpreting lab results and imaging data.
- Track patient outcomes through numerical assessments over time.
- Analyze the efficacy of different treatment options using controlled studies.
In clinical trials, researchers often use statistical models to compare the effects of a new drug versus a placebo. If a drug is statistically proven to reduce symptoms by a significant percentage, this provides a strong argument for its efficacy.
Methods and Techniques
Some common quantitative techniques used in medicine include:
- Descriptive statistics, such as mean, median, and standard deviation, provide a summary of data.
- Inferential statistics, such as t-tests and chi-square tests, allow hypotheses testing on datasets.
- Regression analysis predicts relationships between variables, like linking smoking to lung cancer incidence.
Quantitative methods offer a foundation not just for interpreting current data but also for predicting future trends.
The use of machine learning is advancing the application of quantitative methods. With techniques like deep learning, large datasets such as genomic sequences can be exponentially analyzed. These methods use algorithms to detect patterns and make predictions, revolutionizing personalized medicine.
Statistical Methods in Epidemiology
Epidemiology relies heavily on statistical methods to analyze health data. These methods help in identifying patterns, causes, and effects of health and disease conditions in specific populations. By applying statistics, researchers can make evidence-based decisions and predictions about public health.
Types of Statistical Methods in Epidemiology
There are several types of statistical methods used in epidemiology, which include:
- Descriptive statistics: These summarize the basic features of datasets. Constructs like mean, median, and mode give a snapshot of the data.
- Inferential statistics: These allow for hypothesis testing and infer conclusions from data samples. Methods like t-tests and chi-square tests fall in this category.
- Regression analysis: Used to examine relationships between variables, such as determining risk factors for a disease.
- Survival analysis: This method handles time-to-event data, crucial in understanding life expectancy and disease prognosis.
Suppose researchers are studying the relationship between physical activity and heart disease. Using regression analysis, they might discover that increased activity reduces heart disease risk by a significant margin, leading to actionable public health policies.
Some advanced methods like Bayesian statistics integrate prior knowledge with new evidence to refine analysis. This approach is particularly useful in epidemiology when real-time data is limited and reliance on past studies enhances predictive capabilities.
Statistical significance is crucial in these analyses, generally determined with a p-value less than 0.05.
Application of Statistical Methods in Epidemiology
Statistical methods play a pivotal role in epidemiology, seen in various applications such as:
- Disease surveillance: Tracking disease incidence and prevalence.
- Outbreak investigation: Identifying sources and spread of infectious diseases.
- Evaluating public health interventions: Analyzing data to determine the effectiveness of health programs.
- Risk factor analysis: Associating lifestyle or genetic factors with diseases.
\[ \text{Logit}(p) = \beta_0 + \beta_1X_1 + \cdots + \beta_nX_n \]
to model the probability of an event, like the occurrence of a disease.
Epidemiologists often model potential improvements based on historical data, enhancing future strategies.
The use of meta-analysis combines data from multiple studies, offering a more comprehensive understanding of health issues. It mitigates the limitations of single studies by pooling their findings, increasing the reliability of results in public health.
Data Analysis in Epidemiology
Data analysis in epidemiology aims to extract meaningful patterns from health data. This involves the application of various statistical and analytical methods that help in decision-making for disease control and prevention. Through quantitative methods, epidemiologists can predict trends and identify risk factors.
Tools and Techniques for Epidemiology Data Analysis
To effectively analyze data, epidemiologists use an arsenal of tools and techniques:
- **Statistical Software**: Tools like SPSS, R, and SAS are crucial for handling complex data sets.
- **Data Visualization**: Techniques such as graphs and charts help in illustrating data faults and conclusions.
- **Descriptive Methods**: These summarize data using measures of central tendency and variability.
- **Inferential Methods**: They support hypothesis testing and the prediction of outcomes. For instance, the Chi-square test helps determine relationships between categorical variables.
Machine learning is making headway in epidemiology by analyzing vast amounts of data. Algorithms filter and categorize health data, predicting outbreaks and aiding in precision medicine. The technique of ensemble learning improves prediction by combining multiple machine learning models.
During an influenza outbreak, health departments may use time-series analysis to predict the peak of infections. For instance, using historical data, a model might forecast a peak by mid-January, helping direct resource allocation.
Best Practices for Data Analysis in Epidemiology
Adopting best practices in data analysis bolsters the validity and reliability of epidemiological findings.
- **Data Cleaning**: This involves correcting or removing inaccurate records to improve data quality.
- **Accurate Modeling**: Selecting and validating the correct models prevents oversights in data interpretation.
- **Transparency**: Clearly documenting data collection methods and analyses allows for reproducibility.
Epidemiological Study: A research investigation conducted to uncover health patterns in specific populations.
When modeling diseases, always consider potential confounding variables to avoid biased results.
Longitudinal studies are fundamental in epidemiology, as they track changes in a population over time, yielding crucial insights into the development and progression of diseases. The use of **cohort studies** is particularly beneficial because they establish a timeline of exposure and outcome, enhancing cause-and-effect interpretations.
Epidemiological Data Interpretation
Interpreting epidemiological data is crucial for understanding health patterns, identifying causative factors, and formulating effective public health policies. This process involves the application of statistical methods to deduce meaningful conclusions from raw data.
Challenges in Epidemiological Data Interpretation
Interpreting epidemiological data comes with several challenges, including:
- Data Quality: Inaccuracies and inconsistencies in data can skew results. Ensuring high-quality data is critical for reliable analysis.
- Confounding Variables: These are external factors that can distort the apparent connection between the variables under study.
- Bias: Selection or information bias may lead to incorrect conclusions. Implementing rigorous study designs is crucial to mitigate bias effects.
- Complex Disease Dynamics: Diseases with multifactorial causes, such as diabetes, can complicate data interpretation.
When analyzing the relationship between smoking and lung cancer, confounding factors like air pollution and occupational hazards must be considered. Adjusting for such variables ensures the association between smoking and lung cancer remains clear and unbiased.
The role of multivariable regression in navigating confounding is substantial. This statistical approach quantifies the relationship between multiple predictors and the outcome variable, adjusting for confounders. Consider the model:\[ Y = \beta_0 + \beta_1X_1 + \beta_2X_2 + \ldots + \beta_nX_n + \epsilon \]where \(Y\) is the dependent variable, \(X\) are independent variables, \(\beta\) are coefficients, and \(\epsilon\) is the error term.
Always verify the validity of the data source before diving into data analysis.
Strategies for Accurate Epidemiological Data Interpretation
To achieve accurate interpretation of epidemiological data, employ these strategies:
- Data Cleaning: Ensure data consistency and accuracy by removing or correcting erroneous records before analysis.
- Use of Control Groups: Control groups help in understanding the baseline and minimize bias.
- Adjust for Confounders: Techniques like stratification or multivariate analysis help control potential confounding variables.
- Sensitivity Analysis: This involves testing the robustness of your results by examining how different assumptions affect the outcomes.
Regularly update models and approaches to keep up with new data and evolving understanding of disease dynamics.
Advanced techniques, such as Bayesian data analysis, are gaining traction in epidemiology. Bayesian models incorporate prior distributions, providing a probabilistic framework that enhances decision-making in the face of uncertain data. This approach ensures dynamic model updating as new evidence emerges, offering a robust framework for accurate data interpretation.
Quantitative Techniques in Medicine
In the realm of medicine, quantitative techniques provide a foundational framework for analyzing medical data. These techniques are essential for synthesizing complex datasets into actionable insights, guiding everything from diagnostics to treatment planning and public health strategies.
Role of Quantitative Techniques in Medical Research
Quantitative techniques serve as the backbone of medical research, supporting the development of clinical trials, epidemiological studies, and healthcare policy simulations.
- Clinical Trials: Through numerical data analysis, the efficacy of new drugs and treatments can be evaluated with precision. This often involves statistical tests like the Chi-square test or ANOVA, which help determine any significant differences between experimental and control groups.
- Epidemiological Studies: Quantitative methods allow for the analysis of disease spread, identifying potential causes, and evaluating population health indicators.
In a clinical trial evaluating a new cancer drug, researchers might use a t-test to compare the tumor reduction rates between the treatment and placebo groups. If results show a statistically significant reduction in the treatment group, this supports the drug's potential efficacy.
Incorporating a diverse range of quantitative techniques enhances the robustness of medical research findings.
Advanced quantitative techniques such as machine learning and artificial intelligence are being increasingly integrated into medical research for tasks such as predicting disease progression and personalizing treatment plans. These technologies leverage large volumes of data to uncover patterns not readily apparent through traditional analysis, enabling precision medicine.
Advancements in Quantitative Analysis in Medical Research
Recent technological developments have significantly enhanced quantitative analysis capabilities in medical research. Key advancements include:
- Big Data Analytics: The ability to process massive datasets allows researchers to draw insights from electronic health records and other large-scale health data.
- Genomic Sequencing: Quantitative analysis techniques are crucial for processing genomic data, aiding in identifying genetic markers for diseases.
Using big data analytics, researchers have successfully identified risk factors for chronic diseases like diabetes and cardiovascular disease, leading to more targeted prevention and intervention strategies.
The integration of computational biology with quantitative techniques is revolutionizing the field. Computational models simulate physiological processes, allowing for in-depth exploration of hypotheses that are impractical to test in real-world settings. This approach enables scientists to simulate drug interactions and disease progression scenarios computationally, greatly enhancing our understanding of complex biological systems.
quantitative methods - Key takeaways
- Quantitative Methods Definition: These methods use numerical data and mathematical models for precise analysis and predicting phenomena in various fields, especially medicine.
- Statistical Methods in Epidemiology: Essential for identifying patterns and cause-effect relationships in health data, enabling evidence-based public health decisions and policies.
- Data Analysis in Epidemiology: Focuses on extracting meaningful patterns from health data to guide disease control and prevention strategies.
- Epidemiological Data Interpretation: Involves deducing meaningful conclusions from health data while addressing challenges like data quality, confounding variables, and biases.
- Quantitative Techniques in Medicine: Provide a foundation for analyzing medical data, influencing diagnostics, treatment planning, and public health strategies.
- Advancements in Quantitative Analysis in Medical Research: Includes big data analytics and genomic sequencing, enhancing patient care by identifying genetic markers and risk factors.
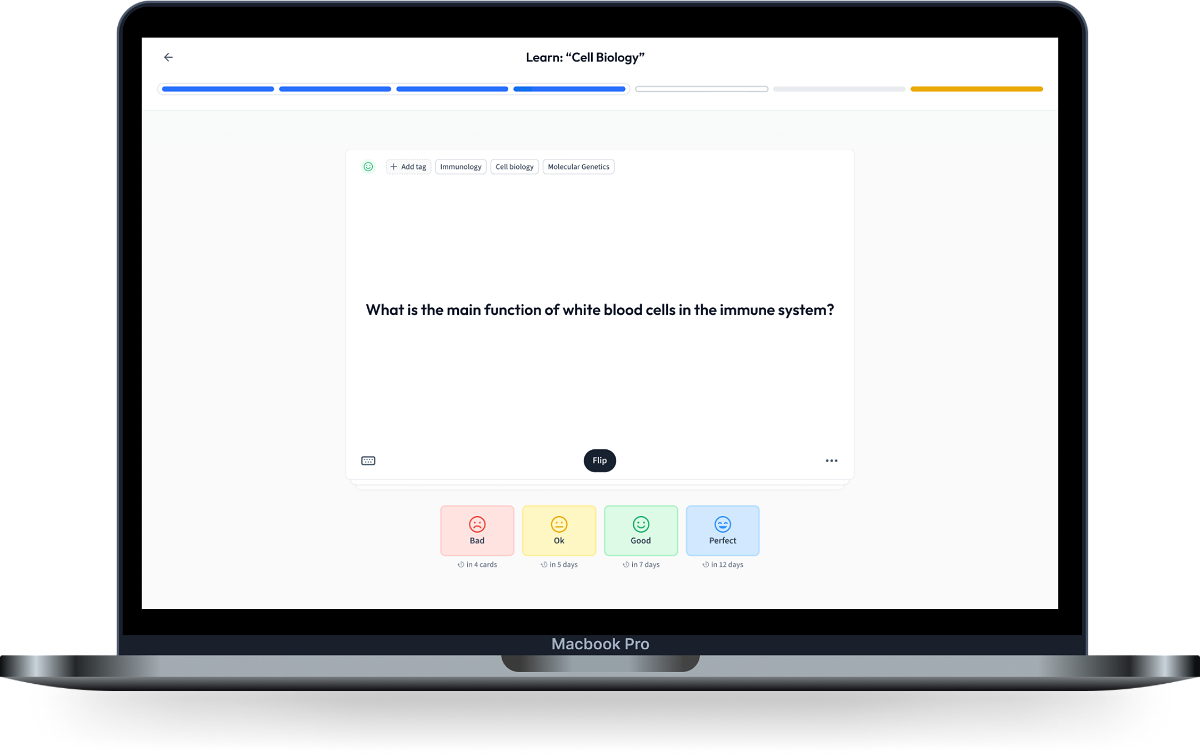
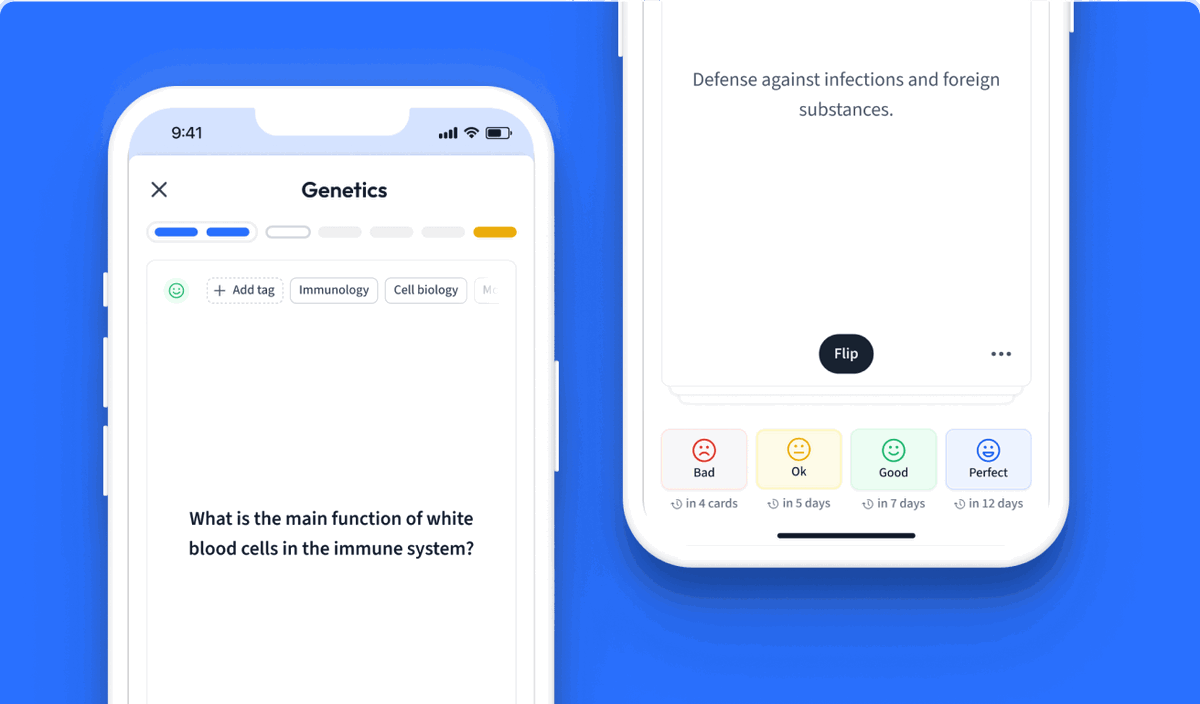
Learn with 10 quantitative methods flashcards in the free StudySmarter app
We have 14,000 flashcards about Dynamic Landscapes.
Already have an account? Log in
Frequently Asked Questions about quantitative methods
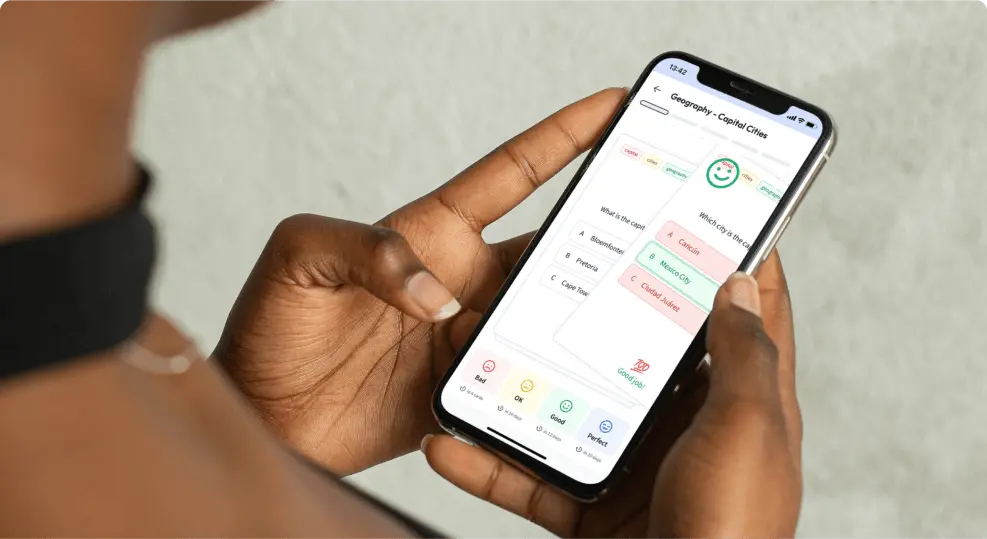
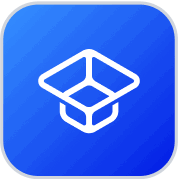
About StudySmarter
StudySmarter is a globally recognized educational technology company, offering a holistic learning platform designed for students of all ages and educational levels. Our platform provides learning support for a wide range of subjects, including STEM, Social Sciences, and Languages and also helps students to successfully master various tests and exams worldwide, such as GCSE, A Level, SAT, ACT, Abitur, and more. We offer an extensive library of learning materials, including interactive flashcards, comprehensive textbook solutions, and detailed explanations. The cutting-edge technology and tools we provide help students create their own learning materials. StudySmarter’s content is not only expert-verified but also regularly updated to ensure accuracy and relevance.
Learn more