Jump to a key chapter
Time to Event Analysis Definition
Time to event analysis is a statistical technique used in medical research to estimate the time until an event of interest occurs. This analysis is crucial when you want to study the duration until a specific health event happens, such as time until recurrence of a disease, time to death, or even time until recovery.
Basic Concepts
In time to event analysis, you will often encounter concepts such as survival functions and hazard functions. The survival function, denoted as \( S(t) \), describes the probability of the event happening after a certain time \( t \). Thus, it is the likelihood that the event of interest has not occurred by time \( t \). The formula for survival function is: \[ S(t) = P(T > t) \] Where \( T \) is the random variable representing the time to the event occurrence. On the other hand, the hazard function, denoted as \( \lambda(t) \), represents the event rate at time \( t \) given that the individual has survived up to time \( t \). The hazard function can be expressed as: \[ \lambda(t) = \lim_{\Delta t \to 0} \frac{P(t \leq T < t + \Delta t \mid T \geq t)}{\Delta t} \] This formula gives you the instantaneous risk of the event occurring at time \( t \).
Survival Analysis and Time to Event Analysis
Time to event analysis is an essential part of medical statistics. It helps you assess the probability and the time duration of specific health-related events. It is crucial to understand its core concepts for accurate analysis and interpretation.
Survival Function and Hazard Function
Survival Function \( S(t) \): Describes the probability that a subject survives beyond time \( t \). Mathematically, it is represented as: \[ S(t) = P(T > t) \] where \( T \) is the time of the event.
Hazard Function \( \lambda(t) \): Represents the instant failure rate or risk of the event at time \( t \), given that the subject has survived until time \( t \). This is expressed as: \[ \lambda(t) = \lim_{\Delta t \to 0} \frac{P(t \leq T < t + \Delta t \mid T \geq t)}{\Delta t} \]
Example of Survival Function: Suppose you are studying the time until the recurrence of a disease. If \( S(4) = 0.6 \), this means there is a 60% probability that the disease has not recurred by the fourth year after treatment.
The relationship between survival and hazard functions can be complex. It often requires more advanced statistical techniques to interpret effectively.
Deep Dive into the RelationshipThe survival function and hazard function are not independent. The hazard function can provide insights into the shape of the survival curve. For example, you can estimate the survival function if the hazard function \( \lambda(t) \) is known: The cumulative hazard function \( \Lambda(t) \) is defined as the integral of the hazard function: \[ \Lambda(t) = \int_{0}^{t} \lambda(u) \, du \] The survival function \( S(t) \) is then derived using the cumulative hazard function: \[ S(t) = e^{-\Lambda(t)} \] This relationship is crucial in translating the understanding of risk into survival probability.
Remember, while conducting time to event analysis, assumptions about the statistical model being used can significantly influence the outcome interpretations.
Kaplan-Meier Estimator in Time to Event Analysis
The Kaplan-Meier estimator is a non-parametric statistic used to estimate the survival function from lifetime data. It's particularly useful when you want to analyze data in which participants have different follow-up times, allowing you to estimate the probability of surviving past certain time points.
Understanding Kaplan-Meier Curves
A Kaplan-Meier curve is a step function that provides a visual representation of the survival function. These curves illustrate how survival probabilities change over time as different participants experience the event of interest. The main advantage of Kaplan-Meier curves is their ability to incorporate censored data, which occurs when the event has not happened for some participants during the study period.The Kaplan-Meier estimator is defined as:\[ \hat{S}(t) = \prod_{t_i \leq t} \left(1 - \frac{d_i}{n_i} \right) \]Where:\
- \
- \( \hat{S}(t) \) is the estimated survival probability at time \( t \) \
- \( d_i \) is the number of events (e.g., deaths) at time \( t_i \) \
- \( n_i \) is the number of individuals at risk just before time \( t_i \) \
Example of Kaplan-Meier EstimatorImagine a clinical trial studying the effectiveness of a new drug. During the trial, some patients drop out or do not experience the event before the study ends. The Kaplan-Meier estimator can provide the probability of survival for those remaining in the study at various time points, despite the censored data.
Kaplan-Meier estimators can be particularly powerful in medical research fields, where variable follow-up times and censoring often occur.
Deep Dive: Limitations and Strengths of Kaplan-MeierWhile the Kaplan-Meier estimator is a robust tool for survival analysis, it has limitations. For instance, it does not account for covariates that might affect the survival rate, such as age or treatment dosage. However, its strength lies in its simplicity and ability to handle right-censored data effectively.The estimator’s graphical representation allows for easy comparison between groups by plotting their survival curves, which can be tested for statistical significance using methods like the log-rank test. This provides a deeper insight into understanding the survival differences among various populations under study when censored and uncensored data is present.
Cox Proportional Hazards Model and Time to Event Data Interpretation
The Cox Proportional Hazards Model is a crucial statistical technique in the field of time to event analysis. It's widely used in medical research for analyzing survival data and focuses on the effect of several variables on the time a specified event takes to happen. Unlike other models, the Cox model is semi-parametric and doesn't require assumptions about the baseline hazard function, making it versatile and powerful.
Censoring in Clinical Trials and Time to Event Analysis
Censoring occurs when the complete information about a subject's event time is not available. In clinical trials, this is a common scenario due to the study ending, subjects dropping out, or the event not occurring by the study's conclusion. The proper handling of censored data is critical to accurate time to event analysis, as it reflects subjects who are still at risk of the event when the study ends.There are primarily three types of censoring in time to event analysis:
- Right Censoring: Most common, occurs if the event has not happened before the study ends or if a participant leaves early.
- Left Censoring: When the event has already occurred before the participant enters the study.
- Interval Censoring: If the event occurs between two observations but the exact time is unknown.
Right Censoring: A scenario in clinical trials where the event of interest has not occurred by the termination of the study or loss of follow-up.
Example of Right CensoringConsider a study investigating the time to recovery after surgery. If a participant has not yet recovered when the study concludes, or if they withdraw from the study prior, their data is right-censored. The analysis must account for their last known status without knowing their eventual outcome.
Censoring, especially right censoring, is a fundamental concept in survival analysis. Improper handling can lead to biased results and misinterpretation.
Deep Dive into Proportional Hazards AssumptionThe Cox model is built on the proportional hazards assumption, where the hazard ratios between groups are constant over time. In mathematical terms, this means: \[ \frac{\lambda_i(t)}{\lambda_j(t)} = \exp(\beta_1 x_1 + \beta_2 x_2 + \cdots + \beta_p x_p) \]where \( \lambda_i(t) \) and \( \lambda_j(t) \) are the hazard functions for individuals \( i \) and \( j \) respectively, and \( \beta_1, \beta_2, \ldots, \beta_p \) are the coefficients denoting the effect of covariates \( x_1, x_2, \ldots, x_p \) on the hazard. It's essential in verifying this assumption because any deviations can lead to misinterpretation of the model's results. Various statistical tests and diagnostic plots, like Schoenfeld residuals, help assess the validity of this assumption, ensuring accurate interpretation of the impact of exploratory variables on survival times.
time to event analysis - Key takeaways
- Time to event analysis definition: A statistical technique used to estimate the time until a health-related event occurs.
- Survival analysis: Assessing probability and duration of specific health events through time to event data.
- Kaplan-Meier estimator: A non-parametric statistic to estimate survival function with censored data.
- Cox proportional hazards model: Analyzes survival data to understand the effect of variables on event timing.
- Censoring in clinical trials: Occurs when incomplete information on the event time is available, critical for accurate analysis.
- Time to event data interpretation: Involves understanding survival and hazard functions, and the handling and incorporation of censored data.
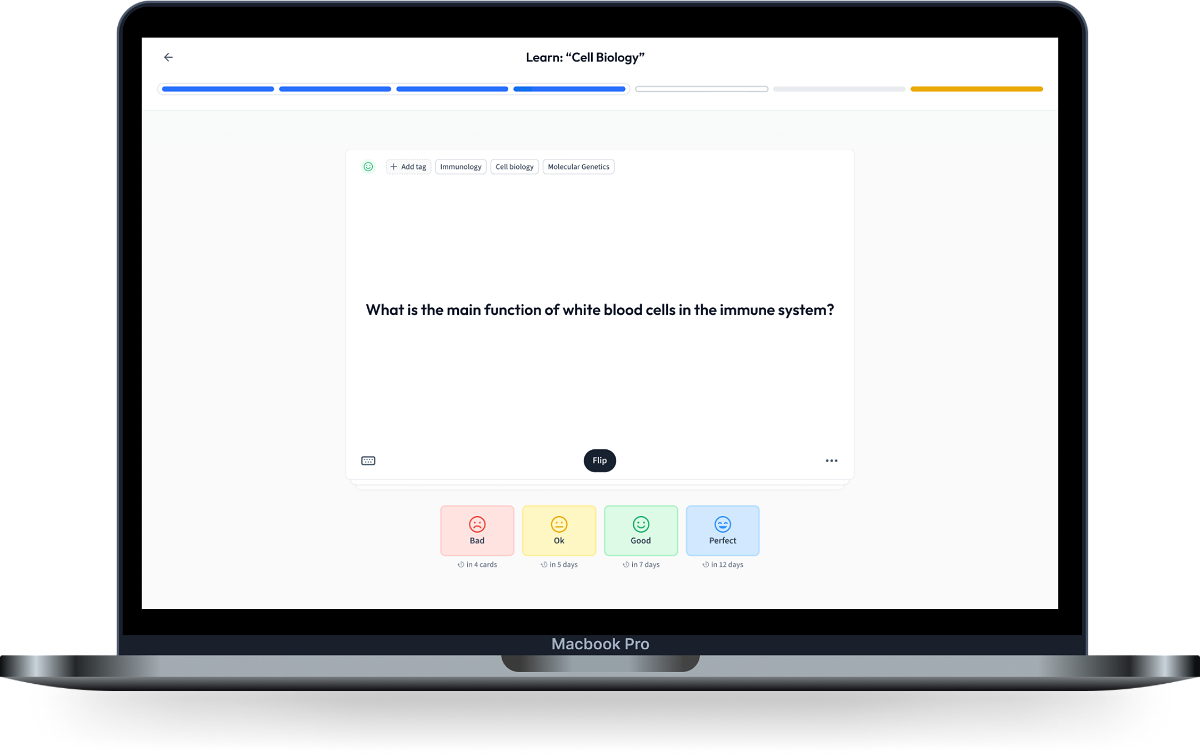
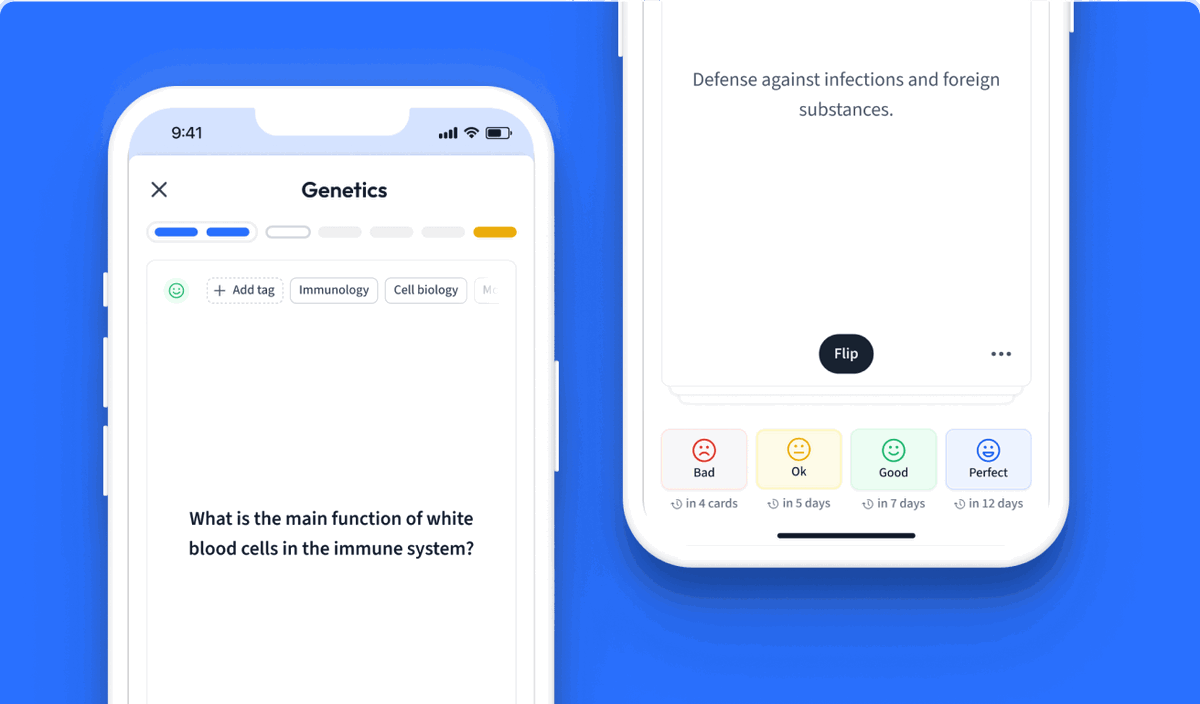
Learn with 12 time to event analysis flashcards in the free StudySmarter app
We have 14,000 flashcards about Dynamic Landscapes.
Already have an account? Log in
Frequently Asked Questions about time to event analysis
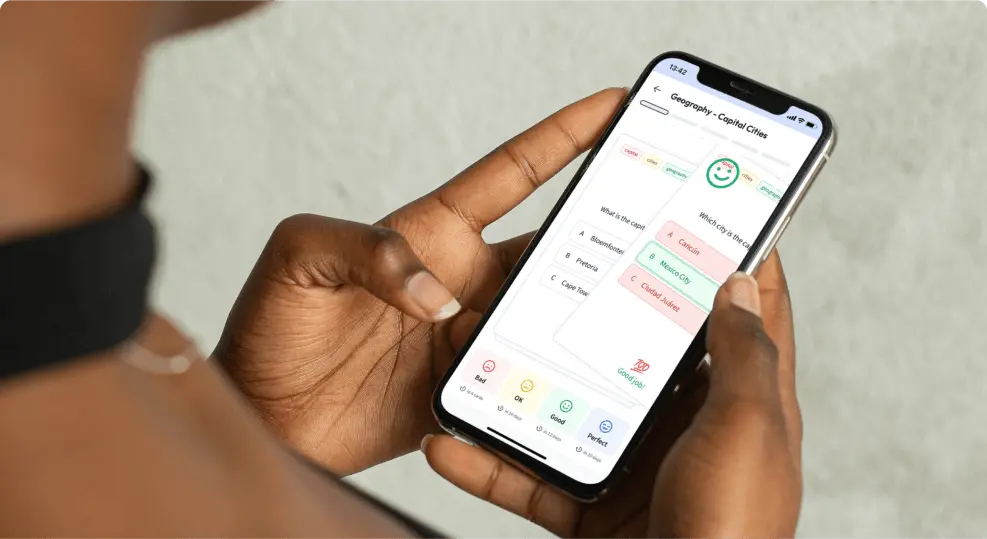
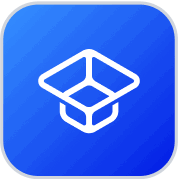
About StudySmarter
StudySmarter is a globally recognized educational technology company, offering a holistic learning platform designed for students of all ages and educational levels. Our platform provides learning support for a wide range of subjects, including STEM, Social Sciences, and Languages and also helps students to successfully master various tests and exams worldwide, such as GCSE, A Level, SAT, ACT, Abitur, and more. We offer an extensive library of learning materials, including interactive flashcards, comprehensive textbook solutions, and detailed explanations. The cutting-edge technology and tools we provide help students create their own learning materials. StudySmarter’s content is not only expert-verified but also regularly updated to ensure accuracy and relevance.
Learn more