Jump to a key chapter
Neuroimaging Genetics Definition
Neuroimaging genetics is an interdisciplinary field combining brain imaging techniques and genetic analysis. The aim is to understand how genetic variations influence brain structure, function, and disorders. By integrating data from these two modalities, researchers can pinpoint genetic factors associated with variations in brain development and function.Studies in this field help in identifying genetic markers that could indicate susceptibility to neurological or psychiatric disorders. This can lead to personalized treatment plans tailored to an individual's genetic profile. Neuroimaging techniques include methods such as MRI (Magnetic Resonance Imaging), fMRI (Functional Magnetic Resonance Imaging), and PET (Positron Emission Tomography). These tools provide detailed brain images, showing structural differences and activity patterns. Coupled with genetic data, these images help to build a comprehensive understanding of the brain's complexity.
Magnetic Resonance Imaging (MRI) is a technique used in neuroimaging genetics that provides high-resolution images of brain structures, helping to visualize differences in brain anatomy associated with genetic variations.
Consider a study focusing on genetic influences on brain volume. Researchers might identify a specific gene variant that appears more frequently in individuals with larger hippocampal volumes. Imagine they observe that individuals with the gene variant DRD2 often show altered activation patterns in the prefrontal cortex during cognitive tasks. This association might suggest that variations in the DRD2 gene influence brain function, particularly in regions responsible for decision-making and attention. Such findings can be illustrated by equations, for instance, the calculation of genetic influence on brain volume could be expressed as \[V = G + E + G \times E\]where V is the brain volume, G represents genetic factors, E refers to environmental influences, and G \times E indicates the interaction between genetics and environment.
Neuroimaging genetics also explores how genetic variants impact neuronal connectivity. One fascinating aspect is the study of brain connectivity networks, often examined through techniques like diffusion tensor imaging (DTI). DTI allows researchers to trace white matter pathways in the brain, providing insights into how certain genetic profiles might influence the organization of neural networks. Researchers use computational models to simulate how different genetic configurations could affect synaptic connectivity and network efficiency. Such models can be quite complex, often relying on sophisticated mathematical frameworks: \[C = \frac{L}{\langle k \rangle N} \]where C is the network connectivity, L is a characteristic path length, \langle k \rangle is the average degree of nodes, and N is the total number of nodes in the network. This quantitative approach offers a deeper understanding of how subtle genetic differences can lead to significant variations in cognitive function and behavior.
Neuroimaging and Genetic Assessment for Early Diagnosis of Alzheimer's Disease
Early diagnosis of Alzheimer's disease (AD) is crucial for effective management and treatment. By integrating neuroimaging genetics, scientists are able to uncover biomarkers that aid in identifying AD before clinical symptoms appear. This approach combines imaging techniques with genetic data to better understand the disease's underlying mechanisms.
Key Neuroimaging Techniques
Several neuroimaging techniques play a pivotal role in the early diagnosis of Alzheimer's disease:
- MRI (Magnetic Resonance Imaging): Provides detailed images of brain structures, helping to detect atrophy in areas like the hippocampus, which is commonly affected in AD.
- PET (Positron Emission Tomography): Measures brain metabolism and amyloid plaque deposition, a hallmark of Alzheimer's disease.
- fMRI (Functional Magnetic Resonance Imaging): Assesses brain activity by detecting changes associated with blood flow.
Amyloid Plagues are protein deposits found in the brains of individuals with Alzheimer's disease, forming between nerve cells and impairing their function.
Suppose researchers conduct a study using PET scans to observe amyloid deposition in individuals with a family history of Alzheimer's. These scans reveal that those carrying the APOE-e4 allele—a known genetic risk factor—exhibit increased amyloid accumulation even when they are asymptomatic. This finding supports the role of genetic markers in predicting Alzheimer's risk.
Genetic Markers and Their Role
Genetic markers provide crucial insights into identifying susceptibility to Alzheimer's disease. Key genetic factors include:
- APOE-e4: The strongest known genetic risk factor for late-onset Alzheimer's.
- Presenilin 1 and 2 (PSEN1/2): Mutations in these genes are linked to early-onset familial Alzheimer's.
- APP (Amyloid Precursor Protein): Variations can lead to abnormal amyloid processing, contributing to AD development.
The presence of the APOE-e4 allele does not guarantee the development of Alzheimer's disease, highlighting the importance of considering both genetic and environmental factors.
When it comes to integrating neuroimaging and genetic data, computational models become invaluable. These models can help simulate disease progression:
Genetic Profile | Imaging Biomarker | Predictive Outcome |
APOE-e4 Positive | High Amyloid Load | Increased Risk |
APOE-e4 Negative | Normal Amyloid Levels | Lower Risk |
Enhancing Neuroimaging Genetics through Meta-Analysis
Meta-analysis is a statistical approach used to synthesize data from multiple studies, providing a comprehensive overview of a research field. In the context of neuroimaging genetics, meta-analysis can be a powerful tool for enhancing the understanding of how genetic variations influence brain structures and functions. By pooling data from various sources, researchers can identify patterns and correlations that might not be evident in individual studies.
The Role of Meta-Analysis in Neuroimaging Genetics
By combining data from various studies, meta-analysis increases the statistical power to detect genetic effects on brain imaging traits. This approach helps to:
- Identify previously undetected genetic associations with brain phenotypes.
- Improve the reliability and robustness of genetic findings in neuroimaging.
- Quantify the magnitude of genetic effects on brain characteristics.
Meta-Analysis is a statistical method that involves combining data from multiple independent studies to derive overarching conclusions and improve statistical power.
Consider a meta-analysis focusing on the relationship between hippocampal volume and genetic markers. A series of independent studies may independently find small effects; however, when combined, the analysis reveals a significant association between the BDNF gene variant and hippocampal size.This example illustrates how meta-analysis can provide more definitive answers than isolated studies. Equations like the following may often be used in such analyses to model the combined effects:\[Z = \frac{a - b}{\sqrt{s_a^2 + s_b^2}}\]where a and b are sample effect sizes and s_a and s_b are standard errors from individual studies.
Meta-analysis in neuroimaging genetics not only helps with hypothesis testing but also can be used to explore heterogeneity across studies. Heterogeneity refers to the variation in study outcomes beyond random error, indicating potential differences in participant populations, methodologies, or measurements.Statistical techniques such as meta-regression are employed to examine these differences:\[Q = \sum_{i=1}^{k} w_i (x_i - \bar{x})^2 \]where Q represents the heterogeneity statistic, w_i are weights, and x_i are effect sizes from individual studies.Unveiling the sources of heterogeneity can guide future research, suggesting avenues for standardizing methodologies or focusing on particular sub-populations for more tailored genetic insights.
Meta-analyses in neuroimaging genetics often use public databases to access large-scale data, improving the scope and accuracy of the analysis.
Genetic Variation and Neuroimaging Measures in Alzheimer Disease
The interplay between genetic variation and neuroimaging measures provides valuable insights into Alzheimer's disease. Understanding these relationships is pivotal in mapping how genetic predispositions contribute to the progression and manifestation of Alzheimer’s.
Neuroimaging Genetics Cross-Validation
Cross-validation is a statistical method used to assess the reliability and accuracy of predictive models combining neuroimaging data and genetic information. In Alzheimer's research, cross-validation ensures that findings are reliable across different datasets.This process typically involves partitioning the data into training and testing sets:
- The training set is used to develop the model.
- The testing set is used to evaluate model performance.
Cross-Validation is a technique for assessing how the results of a statistical analysis will generalize to an independent dataset, crucial for validating models in neuroimaging genetics.
Consider a scenario where a model predicts cognitive decline in Alzheimer's patients using hippocampal volume (from MRI scans) and presence of the APOE-e4 allele, a known genetic risk factor.In cross-validation, the dataset could be split into five parts (5-fold cross-validation). The model is trained on four parts and tested on the fifth, repeated five times, using each part as the test set once. The resulting average accuracy provides a robust estimate of model performance.An expression for cross-validation error might be:\[CV(\theta) = \frac{1}{k} \sum_{i=1}^{k} L_i(x_i, y_i; \theta)\]where \(k\) is the number of folds, \(L\) is the loss function, \(x_i\) are the inputs, \(y_i\) are the observed outputs, and \(\theta\) represents model parameters.
Techniques in Neuroimaging Genetics
The field of neuroimaging genetics employs several sophisticated techniques to explore how genetic differences manifest in brain imaging. These include:
- Genome-wide association studies (GWAS): Used to scan entire genomes for small variations that occur more frequently in individuals with a particular trait, such as Alzheimer's disease.
- Polygenic risk scoring: Aggregates effects of multiple genetic variants to calculate an individual’s genetic predisposition to Alzheimer's.
- Multimodal imaging: Combines different imaging modalities like MRI, fMRI, and PET to provide a comprehensive view of brain anatomy and function.
A deeper look into neuroimaging genetics reveals the integration of machine learning (ML) models to enhance the precision and accuracy of predictions. ML algorithms analyze patterns across genetic and imaging data, identifying combinations of factors that most significantly impact Alzheimer’s progression. Machine learning approaches, like support vector machines or neural networks, are particularly useful for:
- Classifying individuals’ risk of Alzheimer’s based on complex datasets.
- Predicting the rate of cognitive decline.
- Personalizing medicine strategies for Alzheimer's patients.
Polygenic risk scoring provides a summary measure of genetic risk for Alzheimer's, offering insights that extend beyond single-gene analyses.
neuroimaging genetics - Key takeaways
- Neuroimaging Genetics Definition: An interdisciplinary field combining brain imaging techniques and genetic analysis to understand genetic variations affecting brain structure, function, and disorders.
- Early Diagnosis of Alzheimer's: Uses neuroimaging genetics to identify biomarkers for early detection of Alzheimer's, before clinical symptoms appear.
- Key Neuroimaging Techniques: MRI, PET, and fMRI are crucial for detecting structural and functional brain changes relevant to Alzheimer's disease.
- Enhancing Neuroimaging Genetics: Meta-analysis methods improve understanding by synthesizing data across studies, increasing statistical power in detecting genetic associations.
- Neuroimaging Genetics Cross-Validation: A technique for ensuring robustness and reliability of predictive models using genetic and imaging data in Alzheimer's research.
- Techniques in Neuroimaging Genetics: Includes GWAS, polygenic risk scoring, and multimodal imaging, providing comprehensive insights into genetic and neuroimaging interactions.
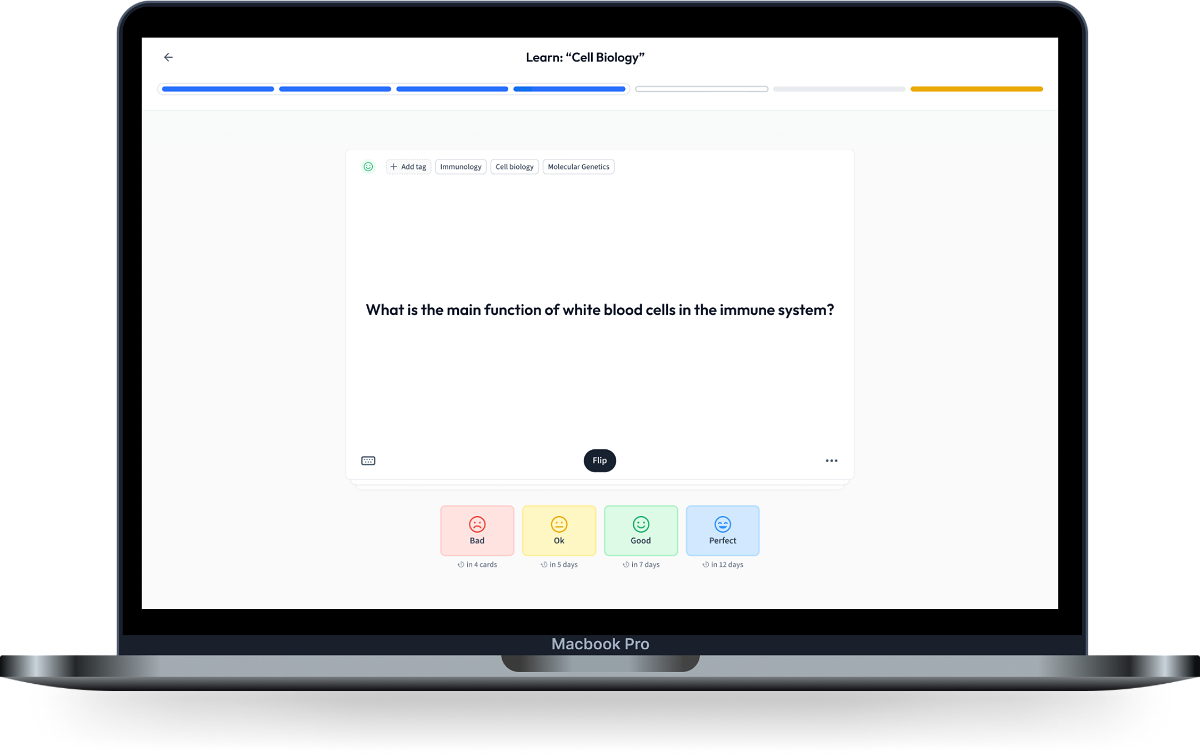
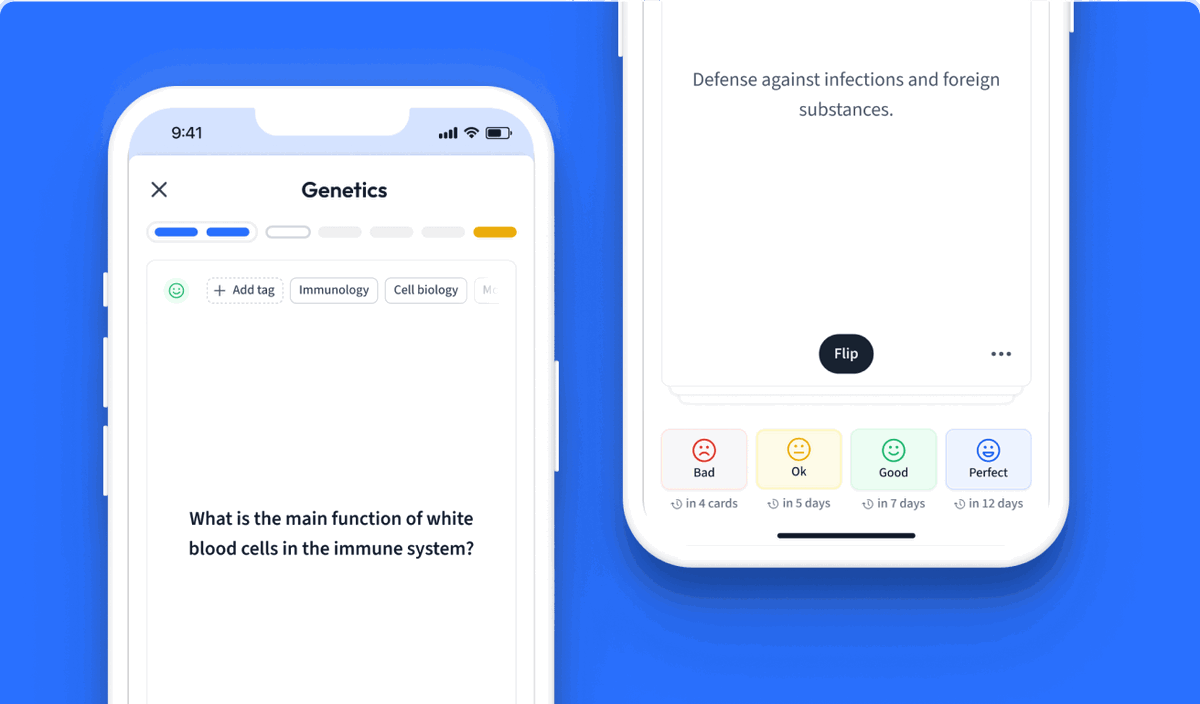
Learn with 12 neuroimaging genetics flashcards in the free StudySmarter app
Already have an account? Log in
Frequently Asked Questions about neuroimaging genetics
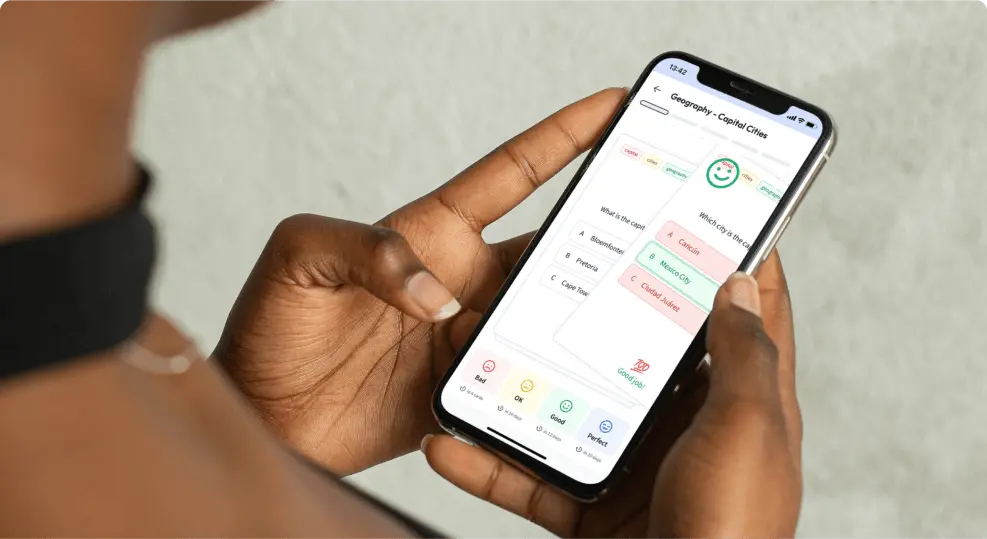
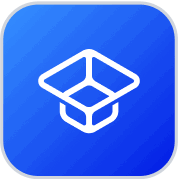
About StudySmarter
StudySmarter is a globally recognized educational technology company, offering a holistic learning platform designed for students of all ages and educational levels. Our platform provides learning support for a wide range of subjects, including STEM, Social Sciences, and Languages and also helps students to successfully master various tests and exams worldwide, such as GCSE, A Level, SAT, ACT, Abitur, and more. We offer an extensive library of learning materials, including interactive flashcards, comprehensive textbook solutions, and detailed explanations. The cutting-edge technology and tools we provide help students create their own learning materials. StudySmarter’s content is not only expert-verified but also regularly updated to ensure accuracy and relevance.
Learn more