Jump to a key chapter
Introduction to Digital Image Analysis in Medicine
Digital Image Analysis is an essential tool in modern medicine, playing a significant role in diagnostics, treatment planning, and research. As a student, understanding this technology can pave the way for numerous advancements in your medical career.
Definition and Purpose of Digital Image Analysis
Digital Image Analysis refers to the process of using computer algorithms to process, analyze, and interpret digital images. It involves extracting meaningful information from images for use in decision-making and scientific research.
Digital Image Analysis in medicine is primarily used to:
- Detect and classify anomalies in medical images such as X-rays, MRIs, and CT scans.
- Measure physical structures and determine relationships in the data.
- Automate repetitive tasks, improving efficiency and accuracy in diagnostics.
Digital Image Analysis leverages techniques from fields like Artificial Intelligence (AI) and Machine Learning (ML) to improve its capabilities. For instance, convolutional neural networks (CNNs) are commonly used for image recognition tasks, allowing for high accuracy in detecting diseases and conditions from radiographic images. The use of digital image analysis is not limited to diagnostics. It can also assist in tissue segmentation, monitor disease progression, and help perform virtual biopsies.
Importance for Medical Students
As a medical student, gaining proficiency in Digital Image Analysis can significantly benefit your career. Here are several reasons why it's important:
- Enhanced Diagnostic Skills: The ability to interpret digital images and use analytical tools is crucial for identifying patient issues accurately and efficiently.
- Integration With Technological Developments: Being familiar with the latest technologies helps ensure you're at the forefront of medical innovations.
- Research and Development Opportunities: With a firm grasp of image analysis, you can contribute to groundbreaking research leading to improved healthcare outcomes.
Familiarity with Digital Image Analysis allows you to collaborate across multidisciplinary teams, combining insights from radiology, pathology, and AI.
Applications in Medicine
Digital Image Analysis is widely used in multiple medical areas:
- Radiology: It enhances the accuracy of evaluating imaging results, aiding in the early detection of conditions such as tumors or fractures.
- Pathology: Utilization of image analysis allows for automatic identification of cellular structures and anomalies, which facilitates faster pathological assessments.
- Ophthalmology: Through the analysis of retinal images, it assists in diagnosing diseases like diabetic retinopathy.
For example, in cardiology, digital image analysis can measure the dimensions of the heart and blood vessels using CT scans, providing insights into cardiovascular health and guiding treatment options.
Digital Image Analysis Techniques in Medicine
In the realm of modern medicine, Digital Image Analysis plays an indispensable role. By converting visual information into quantitative data, it assists healthcare professionals in making informed decisions. The process goes beyond what the human eye can discern, offering objective results that enhance patient care.
Common Techniques Used in Medicine
Different techniques are applied in digital image analysis to retrieve, process, and interpret medical images effectively. Some of the most common techniques include:
- Image Segmentation: Divides an image into multiple parts or segments to simplify analysis. It helps in isolating important structures such as organs or lesions.
- Pattern Recognition: Utilizes algorithms to recognize patterns and structures within images. This is critical in diagnosing diseases from radiological scans.
- Machine Learning Applications: Employs AI algorithms to improve image interpretation over time, learning from new data for enhanced accuracy.
For an in-depth understanding of segmentation, consider how thresholding methods segment based on intensity values. More advanced approaches, like region-based and edge detection techniques, further refine segmentation by using the inherent properties and boundaries within an image. This can greatly aid in distinguishing tumor boundaries in oncology.
A practical example of pattern recognition can be found in dermatology, where digital image analysis helps to classify skin lesions based on texture and form. This assists dermatologists in differentiating between benign and malignant moles, improving diagnostic accuracy.
Advances and Innovations
With the rise of technology, the field of Digital Image Analysis is continuously evolving. Recent innovations have introduced:
- Deep Learning: A subset of machine learning that focuses on neural networks, particularly beneficial in analyzing complex and large medical datasets.
- 3D Imaging: Allows for volumetric analysis, providing a more comprehensive view of anatomical structures.
- Real-time Image Processing: Facilitates immediate analysis during surgeries or diagnostic procedures, assisting in time-critical decision-making.
Keep an eye on how augmented reality (AR) is being explored to visualize 3D medical images in real-time, which could revolutionize surgical planning and education.
Digital Pathology Image Analysis
Digital Pathology Image Analysis is transforming how medical professionals approach diagnostics and research. By converting glass slides into digital images, this technology offers new opportunities for precision and efficiency in pathology.
Role in Pathology
Pathology, the study of diseases through organ, tissue, and body fluid examination, relies heavily on image analysis. Digital methods enhance traditional techniques by providing:
- Improved Accuracy: Computer algorithms can detect patterns and anomalies that might be missed by human eyes.
- Faster Turnaround: Digital slides can be analyzed and shared quickly, speeding up diagnostic processes.
- Remote Consultations: Pathologists can collaborate globally by sharing digital slides and analysis results online.
For instance, in breast cancer pathology, digital image analysis helps in identifying and quantifying HER2 receptors in tumor cells, assisting in determining the right treatment plan.
Interesting developments revolve around the integration of artificial intelligence (AI) with digital pathology. AI systems can learn to recognize patterns in vast datasets, aiding in predicting patient outcomes and personalizing treatments. This capability could potentially revolutionize pathology by enabling highly accurate and predictive models of disease behavior.
Tools and Technologies
A variety of tools and technologies are available to enhance Digital Pathology Image Analysis. These include:
- Whole Slide Imaging (WSI): Converts entire histopathology slides into high-resolution digital formats for comprehensive analysis.
- Image Management Systems: Facilitate the storage, retrieval, and analysis of large volumes of digital slides.
- Analysis Software: Employ advanced algorithms for tasks such as cell counting, tissue classification, and marker quantification.
Open-source software options like ImageJ and QuPath offer extensive tools for analyzing microscopic images and are favored by researchers for custom analysis tasks.
Whole Slide Imaging (WSI) is a technology that transforms entire pathological slides into detailed digital images, enabling more thorough and accurate assessments.
An example of analysis software is Aperio Imagescope which provides features like panning, zooming, and annotations to facilitate detailed image evaluation.
Applications of Digital Image Analysis in Medicine
Digital Image Analysis holds great promise across various domains in the medical field. By transforming visual data into actionable insights, it vastly enhances clinical efficiency, research potential, and future healthcare models.
Clinical Applications
In clinical settings, Digital Image Analysis is pivotal for:
- Early Disease Detection: Algorithms can identify early signs of diseases in medical images, such as tumors in MRI scans, which might not be immediately apparent to the human eye.
- Automated Diagnostics: With machine learning, systems can learn from substantial data, improving diagnostic accuracy and consistency.
- Treatment Planning: Image analysis helps in measuring lesions and anatomical structures, aiding in precise radiation therapy targeting.
For example, in ophthalmology, digital image analysis of retinal scans can detect diabetic retinopathy early on, allowing for timely intervention.
Machine Learning models are specifically trained on large datasets and tend to excel in recognizing complex patterns from medical images.
An advanced application is in imaging biomarkers, where image features are used to predict pharmacogenomic interactions. This approach can provide personalized treatment plans by assessing how different patients might respond to medication based on image-derived analytics.
Research and Development
In research, Digital Image Analysis accelerates discoveries with applications such as:
- Cellular Analysis: Helps quantify and characterize cellular components, essential for understanding pathological processes.
- Genomics Integration: Combines imaging data with genomic information to uncover insights about disease mechanisms.
- Therapeutic Development: Enhances the development of new drugs by analyzing tissue responses at a microscale level.
A fascinating development within research is the 'virtual biopsies' enabled by high-resolution imaging technologies. By analyzing live digital images, these virtual biopsies may reduce the need for invasive procedures by providing detailed tissue analysis in vivo.
Future Prospects and Trends
Looking ahead, various trends are shaping the future of digital image analysis in medicine:
- Integration with AI: Artificial intelligence continues to bolster image analysis, moving towards fully automated diagnostic systems.
- Increased Accessibility: Cloud-based platforms are making digital analysis tools more widely accessible to remote areas.
- Enhanced Precision: The trend towards precision medicine is supported by more detailed and specific image analyses, refining patient healthcare.
For instance, the rise of wearable technology with integrated sensors can provide real-time health data and digital images for continuous monitoring, potentially revolutionizing patient care.
Keep watch for developments in quantum computing, which may significantly accelerate data processing capabilities in digital image analysis.
With advancements in 5G technology, real-time, high-quality digital imaging and analysis can be conducted remotely, opening avenues for telemedicine and instant diagnostic services beyond borders.
digital image analysis - Key takeaways
- Digital Image Analysis is the use of computer algorithms to process and interpret digital images for decision-making and research, playing a key role in modern medicine.
- Techniques in digital image analysis like image segmentation, pattern recognition, and machine learning enhance its application in detecting diseases and conditions from radiological images.
- Medical students benefit from understanding digital image analysis by improving diagnostic skills, staying abreast with technological advances, and contributing to research.
- Applications include radiology for evaluating imaging results, pathology for cellular structure identification, and ophthalmology for diagnosing retinal diseases.
- Advances in digital image analysis technologies include deep learning, 3D imaging, and real-time image processing, impacting clinical workflows and personalized medicine.
- Digital Pathology Image Analysis increases accuracy, speeds up diagnostic processes, and facilitates remote consultations through tools like Whole Slide Imaging and analysis software.
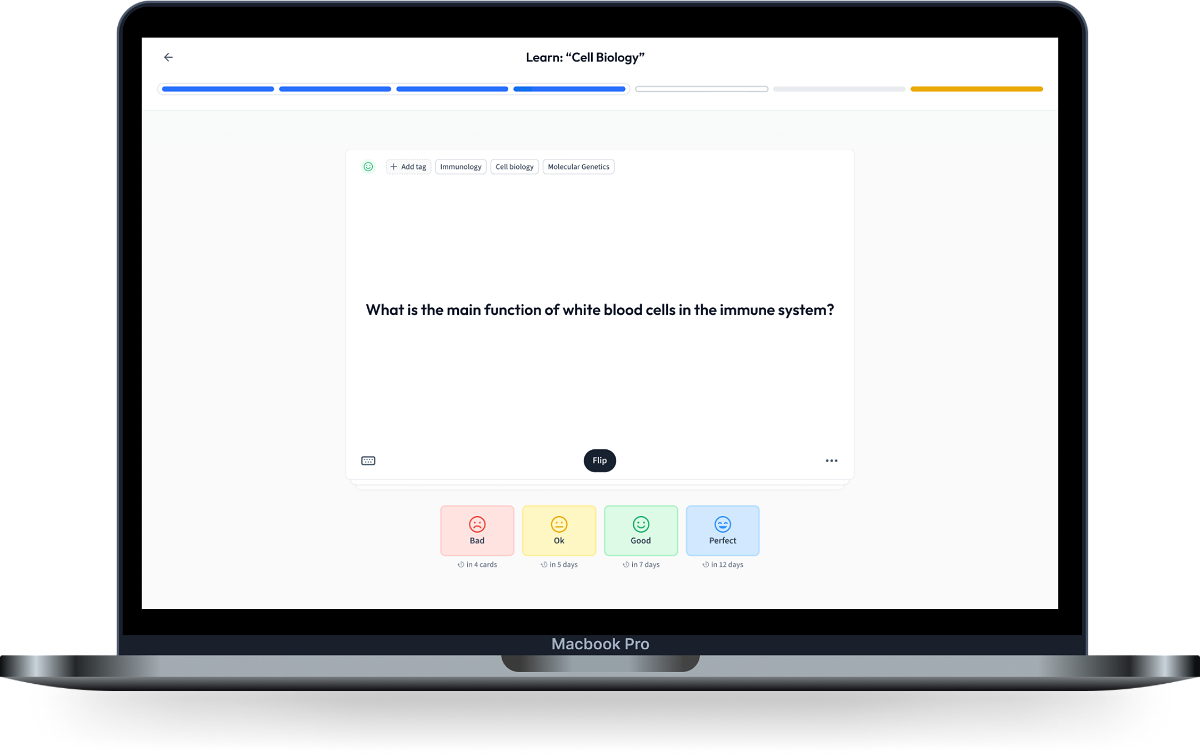
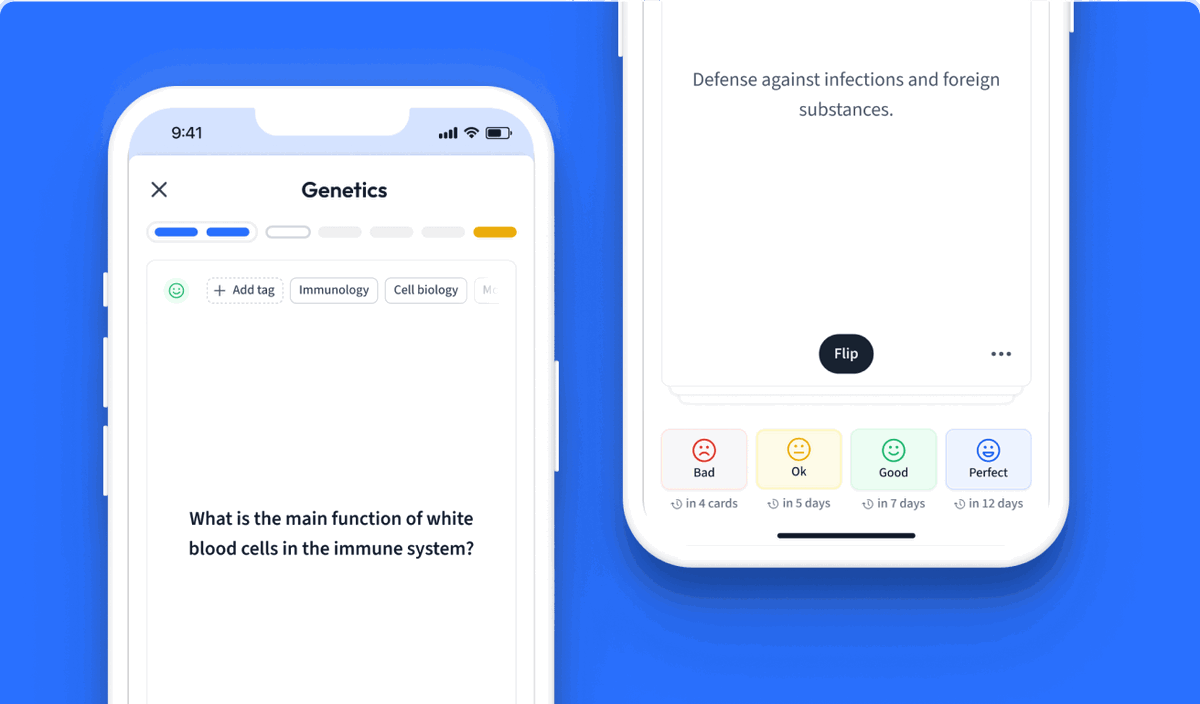
Learn with 24 digital image analysis flashcards in the free StudySmarter app
We have 14,000 flashcards about Dynamic Landscapes.
Already have an account? Log in
Frequently Asked Questions about digital image analysis
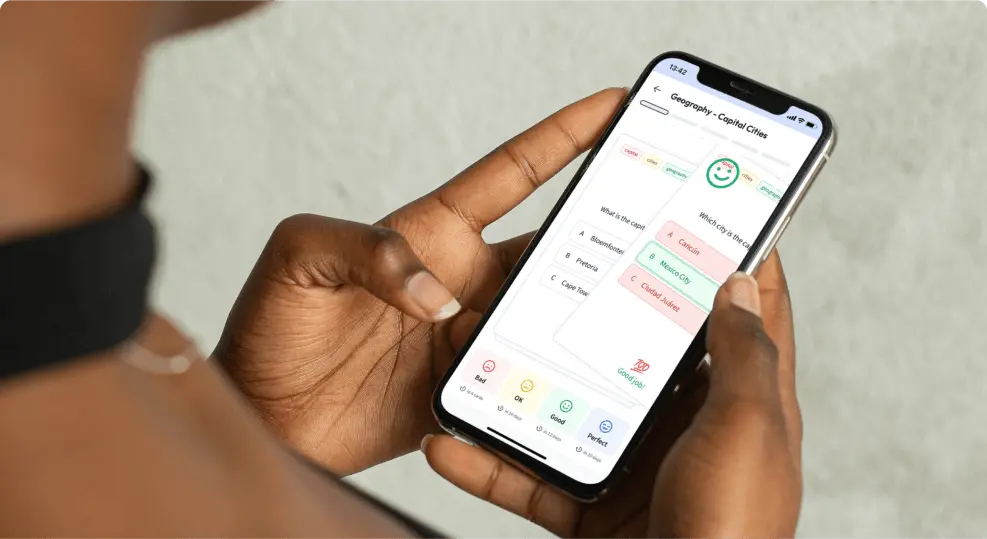
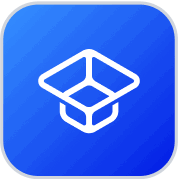
About StudySmarter
StudySmarter is a globally recognized educational technology company, offering a holistic learning platform designed for students of all ages and educational levels. Our platform provides learning support for a wide range of subjects, including STEM, Social Sciences, and Languages and also helps students to successfully master various tests and exams worldwide, such as GCSE, A Level, SAT, ACT, Abitur, and more. We offer an extensive library of learning materials, including interactive flashcards, comprehensive textbook solutions, and detailed explanations. The cutting-edge technology and tools we provide help students create their own learning materials. StudySmarter’s content is not only expert-verified but also regularly updated to ensure accuracy and relevance.
Learn more