Jump to a key chapter
Introduction to Molecular Modeling in Medicine
Molecular modeling is a powerful tool in the field of medicine, providing insights into the structural and functional aspects of biomolecules. This plays a crucial role in drug design, understanding disease mechanisms, and developing new therapies.
Molecular Modeling Basics for Students
Molecular modeling involves computational techniques that simulate the behavior of molecules. These models help predict the interaction between different molecules, which is vital for understanding various biological processes.A basic understanding of chemistry, biochemistry, and physics is necessary to appreciate the intricacies of molecular modeling. Key concepts include:
- Atomic and molecular structure
- Chemical bonding
- Thermodynamics
- Quantum mechanics
Molecular modeling: A computational technique used to model and predict the structure and behavior of molecules.
Consider water (H2O), a simple molecule. Using molecular modeling, you can predict its molecular geometry, bond angles, and potential energy using computational methods.
While the basic principles of molecular modeling make use of classical physics, advanced molecular modeling goes deeper into quantum mechanics. The density functional theory (DFT) is a widely used quantum mechanical modelling method. It considers electron density rather than wavefunctions to solve quantum mechanical problems. The advantage is its efficiency in computing the properties of large systems.Moreover, machine learning methods are now being integrated into molecular modeling to enhance prediction accuracy and speed. These algorithms can analyze vast amounts of data, learning patterns and making correlations that enhance traditional molecular modeling approaches.
Molecular Modeling Techniques in Medicine
Molecular modeling in medicine is primarily utilized in drug development and the study of biomolecular interactions.Drug design benefits significantly from molecular modeling as it helps design new drugs by simulating their interaction with target proteins. This process often involves in silico experiments that reduce the need for costly laboratory testing. Here are a few techniques:
- Molecular Dynamics: It examines the physical movements of atoms and molecules, providing insights into the stability and dynamics of molecular structures over time.
- Docking simulations: These predict how drugs bind to their target proteins. The binding energy calculated can give an indication of the effectiveness of a drug.
- Quantitative Structure-Activity Relationship (QSAR): This involves the statistical analysis of chemical structures to find patterns between the constitution of a chemical compound and its biological activity.
Remember, molecular modeling can significantly reduce the time and cost involved in developing new drugs.
Applications of Molecular Modeling in Medicine
Molecular modeling has become an indispensable tool in medicine due to its ability to simulate molecular interactions and predict the behavior of biological molecules. Two major areas where it has a tremendous impact are drug discovery and pharmacology.
Molecular Modeling in Drug Discovery
The role of molecular modeling in drug discovery is pivotal. It accelerates the process of discovering new compounds that can act as potential drugs. By simulating how a drug interacts with a target protein, researchers can identify the best candidates for further development.Key Processes in drug discovery using molecular modeling include:
- Structure-Based Drug Design: This involves designing a drug based on the three-dimensional structure of its target.
- Ligand-Based Drug Design: Uses knowledge of molecules that bind to a particular protein to design new compounds.
Method | Use |
Molecular Docking | Predicts the preferred orientation of a small molecule when bound to a target protein. |
Pharmacophore Modeling | Identifies the structural features necessary for a molecule to interact with a specific target. |
Binding Energy: The energy required to disassemble a molecule into separate entities.
Consider the design of HIV protease inhibitors. Molecular modeling has enabled the design of drugs that fit precisely into the active site of the HIV protease, thus blocking its activity and preventing the virus from maturing.
Molecular modeling tools can simulate millions of molecules in a fraction of the time needed for laboratory testing, thereby speeding up drug discovery.
Molecular Modeling in Pharmacology
In pharmacology, molecular modeling helps understand drug action mechanisms, predict drug interactions, and assess safety profiles. By modeling molecular interactions, researchers can explore how different drugs influence biological pathways and enzymes.Molecular Dynamics Simulations is a technique frequently applied in pharmacology to study:
- The influence of a drug on cell membranes and its penetration processes.
- Conformational changes in receptor proteins after drug binding.
Molecular modeling in pharmacokinetics can predict how effectively a drug will reach its target and remain there.
Advanced applications of molecular modeling in pharmacology include the integration of artificial intelligence (AI) and machine learning algorithms. These technologies analyze vast datasets to improve the accuracy of pharmacokinetic predictions and identify novel drug candidates. By leveraging AI, researchers can:
- Enhance the prediction of complex molecular interactions.
- Identify optimized molecular pathways for drug efficacy.
- Reduce the time and cost associated with traditional experimental methodologies.
Molecular Modeling Techniques in Medicine
Molecular modeling is an integral part of modern medical research, allowing scientists to simulate and visualize molecular and atomic interactions. This ability to model at such small scales aids in drug discovery, protein synthesis, and understanding the fundamental aspects of biological functions.Molecular modeling encompasses several computational methods that help visualize molecular structures and dynamics.
Core Techniques in Molecular Modeling
- Molecular Dynamics (MD): This simulates the physical movements of atoms and molecules over time, providing insights into the structural dynamics and thermodynamics of molecular systems.
- Quantum Mechanics (QM): Uses the principles of quantum theory to accurately predict the behavior of electrons in molecules, crucial for understanding chemical reactions.
- Docking Studies: These predict the preferred orientation of molecules when they form a complex. It is particularly important for drug design.
Molecular Dynamics (MD): A simulation method in computational chemistry that allows the observation of the movement of molecules.
In drug discovery, MD simulations can help determine the stability of a drug-protein complex, which is crucial for assessing the therapeutic potential of new drug candidates.
Docking studies are essential for virtual screening of large compound libraries in searching for new drugs.
One advanced application of molecular modeling in medicine is the study of protein-ligand interactions, which play key roles in cellular processes. Combining QM and MD through hybrid approaches known as QM/MM (Quantum Mechanics/Molecular Mechanics) allows for accurate simulations of biomolecular systems by capturing electron-level detail within a macromolecule.
- QM/MM allows for simulations of chemical reactions within enzymes, where the reacting part of the enzyme is modeled using quantum mechanics, while the rest is treated using molecular mechanics.
Molecular Modeling in Drug Discovery
Molecular modeling plays a pivotal role in drug discovery by offering insights into the interaction between drugs and their biological targets. This technology helps identify ideal candidates for drug development by simulating molecular structures and dynamics. By reducing the reliance on trial-and-error methods, molecular modeling allows for a more targeted approach to drug design.In drug discovery, techniques such as docking simulations and structure-based drug design (SBDD) are commonly utilized. Docking simulations predict the preferred orientation of a drug molecule when bound to a target protein, providing a strong indication of its effectiveness. This can be expressed mathematically by calculating the binding energy, often given by:
Binding Energy: The energy difference between the bound and unbound states of a molecule, calculated as:\[ E_{\text{binding}} = E_{\text{complex}} - (E_{\text{protein}} + E_{\text{ligand}}) \]
An example of molecular modeling in drug discovery is the development of aspirin analogs. By using computational docking techniques, researchers can predict which analogs are likely to bind effectively to the cyclooxygenase enzyme and inhibit its function, thus providing anti-inflammatory effects.
Beyond traditional techniques, modern drug discovery leverages machine learning in molecular modeling. Algorithms can analyze large datasets to recognize patterns, improving the prediction accuracy of molecular interactions. This integration enhances the discovery of drug candidates by identifying trends that were not previously observable.
- Machine Learning Models: These models can predict the pharmacological profiles of new compounds faster than conventional methods.
- The use of artificial intelligence improves the success rate of predicting absorption, distribution, metabolism, and excretion (ADME) properties.
Role of Molecular Modeling in Pharmacology
Molecular modeling is crucial in pharmacology as it provides a better understanding of how drugs interact with biological systems, paving the way for more effective treatments. Through simulations like molecular dynamics, scientists can observe the movements and conformational changes of molecules over time, which is essential for understanding drug efficacy.In pharmacology, molecular modeling is used to:
- Predict potential drug interactions by modeling binding sites on target proteins such as G-protein coupled receptors.
- Analyze metabolic pathways to identify potential side effects and adverse reactions.
Pharmacological studies benefit from molecular modeling by reducing the time and cost of experimental drug trials.
Advances in Molecular Modeling Methods in Medicine
Advancements in molecular modeling methods have transformed medicinal research, especially with improvements in computational power and techniques. These developments have enabled more detailed and extensive simulations, allowing researchers to model larger biological systems more accurately.Key advancements include:
- Hybrid QM/MM Approaches: These combine quantum mechanics and molecular mechanics to model reactions occurring within biosystems. By maintaining accuracy in reactive regions and efficiency in non-reactive regions, this approach has revolutionized enzyme analysis.
- Enhanced Sampling Techniques: These methods, such as metadynamics and umbrella sampling, allow for the exploration of rare events and free energy landscapes, providing deeper insights into protein folding and drug binding pathways.
molecular modeling - Key takeaways
- Molecular Modeling: Involves computational techniques to simulate the behavior and interactions of molecules, critical in medicine for drug design and understanding disease mechanisms.
- Molecular Modeling Basics for Students: Requires understanding atomic and molecular structure, chemical bonding, thermodynamics, and quantum mechanics, with tools like the Schrodinger equation for predicting molecular behaviors.
- Applications in Medicine: Used extensively in drug development for predicting drug interactions with target proteins (e.g., docking simulations, QSAR) and studying disease mechanisms, especially for cancer and genetic disorders.
- Molecular Modeling Techniques in Medicine: Key techniques include Molecular Dynamics (MD) for simulating atomic movements, Quantum Mechanics (QM) for electron behavior, and novel approaches like QM/MM for enzyme reactions.
- Drug Discovery Applications: Accelerates drug discovery through structure-based and ligand-based drug design, and predicts binding energies to identify lead compounds.
- Role in Pharmacology: Enhances understanding of drug action, prediction of drug interactions, and optimization of pharmacokinetic properties like ADME, often enhanced by AI and machine learning.
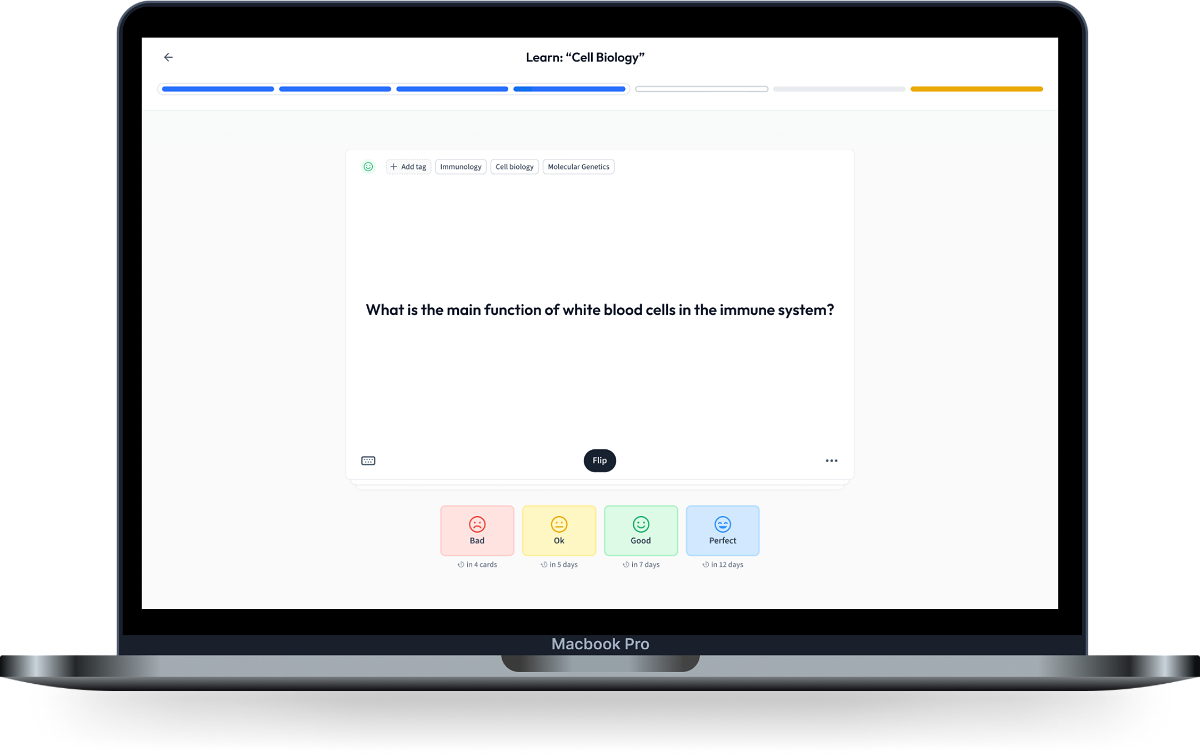
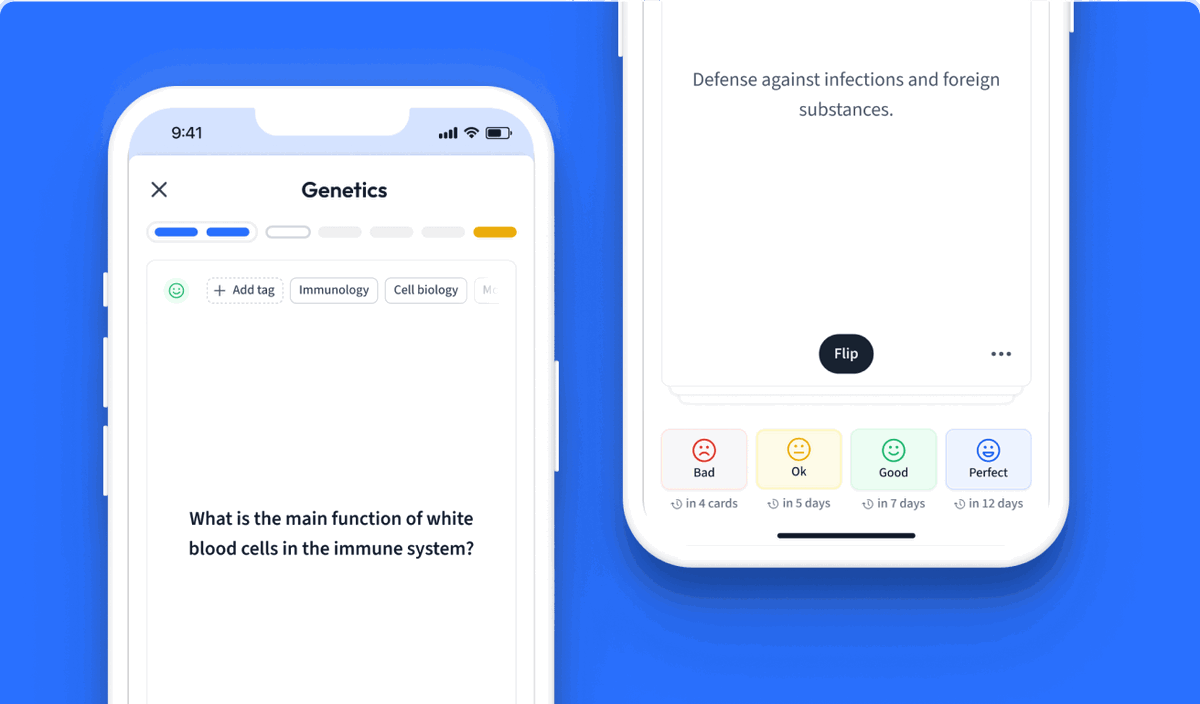
Learn with 12 molecular modeling flashcards in the free StudySmarter app
We have 14,000 flashcards about Dynamic Landscapes.
Already have an account? Log in
Frequently Asked Questions about molecular modeling
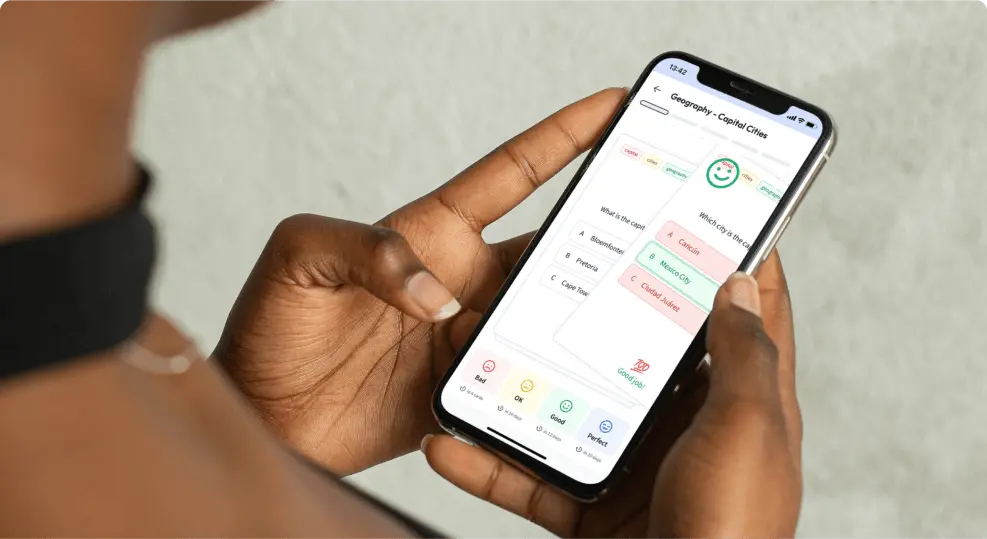
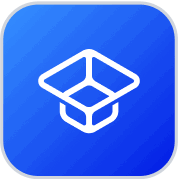
About StudySmarter
StudySmarter is a globally recognized educational technology company, offering a holistic learning platform designed for students of all ages and educational levels. Our platform provides learning support for a wide range of subjects, including STEM, Social Sciences, and Languages and also helps students to successfully master various tests and exams worldwide, such as GCSE, A Level, SAT, ACT, Abitur, and more. We offer an extensive library of learning materials, including interactive flashcards, comprehensive textbook solutions, and detailed explanations. The cutting-edge technology and tools we provide help students create their own learning materials. StudySmarter’s content is not only expert-verified but also regularly updated to ensure accuracy and relevance.
Learn more