Jump to a key chapter
Research & Analysis in Medicine
Research and analysis are essential components of medical science, providing a foundation for evidence-based practice and clinical decision-making. They involve various methods and tools that ensure the reliability and validity of medical findings.
Meta-analysis in Medical Research
Meta-analysis in medical research involves combining and analyzing data from multiple studies to identify patterns, differences, or treatment effects. By pooling data, researchers can overcome the limitations of small individual studies, increasing statistical power and precision. Meta-analyses typically include the following steps:
- Defining the research question.
- Searching for relevant studies.
- Selecting studies based on inclusion criteria.
- Extracting and analyzing data.
- Interpreting results.
An example of meta-analysis is pooling data from numerous clinical trials to evaluate the efficacy of a new cancer drug. By consolidating these results, researchers can better understand the drug's overall effect.
When conducting a meta-analysis, it's crucial to use consistent and appropriate statistical methods to ensure the results are accurate.
Systematic Reviews in Medicine
A systematic review is a methodical and comprehensive literature review focused on a specific research question in medicine. It identifies, appraises, and synthesizes all relevant studies on a particular topic, providing an exhaustive summary of current evidence.Key features of systematic reviews include:
- Comprehensive literature search strategies.
- Transparent and replicable methods.
- Critical appraisal of study quality.
Systematic reviews can sometimes include a meta-analysis if they quantitatively combine data from different studies.
Research Methodology in Medicine
The research methodology in medicine includes a variety of methods and techniques used to gather, analyze, and interpret data. Scientific research can be categorized into observational and experimental studies, each with unique characteristics.Common methodologies include:
- Randomized Controlled Trials (RCTs): Considered the gold standard for testing interventions.
- Cohort Studies: Longitudinal studies observing outcomes in defined groups over time.
- Case-Control Studies: Compare patients with a condition to those without to identify risk factors.
Understanding different research methodologies allows researchers to choose the most appropriate design for their study. For instance, observational studies might be selected when ethical or practical issues prevent conducting RCTs. In contrast, RCTs are ideal when the aim is to assess the efficacy of a treatment by randomly assigning participants into groups.
Medical Research Ethics
Ethics in medical research are principles and standards guiding the conduct of research to ensure integrity and protect participants. Ethical considerations include informed consent, risk minimization, and confidentiality.The core principles of medical research ethics are:
- Respect for Persons: Recognizes autonomy and the need for informed consent.
- Beneficence: Obligation to minimize harm and maximize benefits.
- Justice: Fair distribution of research benefits and burdens.
For instance, during clinical trials, participants must be provided with comprehensive information about the study's purpose, risks, benefits, and their rights before consenting to participate.
Epidemiological Studies in Medicine
Epidemiological studies are pivotal in understanding the distribution, patterns, and determinants of health and disease conditions in defined populations. They provide crucial insights that inform public health policy and practices.
Role of Epidemiological Studies in Research & Analysis
The role of epidemiological studies in research and analysis is multifaceted, contributing significantly to our understanding of health outcomes. These studies help to:
- Identify risk factors for diseases and conditions.
- Determine optimal prevention and treatment strategies.
- Inform public health policies and interventions.
\[Rate = \frac{Number\;of\;new\;cases}{Population\;at\;risk\;during\;time\;period}\]
For example, a study examining the relationship between smoking and lung cancer uses epidemiological research to track the development of cancer in smokers versus non-smokers over several years.
Designing Epidemiological Studies
Designing epidemiological studies requires careful planning to ensure reliable results. Consider the following steps in designing these studies:
- Define the study question and objectives clearly.
- Select an appropriate study design (e.g., cohort, case-control).
- Determine the sample size to ensure statistical power.
- Control for confounding factors through stratification or multivariable analysis.
- Ensure ethical considerations and obtain necessary permissions.
Using random sampling techniques can help reduce selection bias in epidemiological studies.
Understanding **confounding variables** is crucial in study design as they can distort the apparent effect of the exposure on the outcome. For instance, if you're studying the relationship between exercise and heart disease, age could be a confounder because it is associated with both the likelihood of exercising and heart disease risk. The formula to adjust for confounders might involve stratified analysis techniques or multivariate models. Considerations like matching, stratification, and using control groups are methods to handle confounding.
Challenges in Epidemiological Research
Epidemiological research faces several challenges that can complicate study execution and interpretation:
- Bias: Both selection bias and information bias can affect outcomes.
- Confounding: Distorts the relationship between exposure and outcome.
- Variability: Differences in data collection methods and definitions across studies.
- Ethical concerns: Protecting privacy and ensuring informed consent.
When interpreting epidemiological data, consider potential sources of error and bias to make informed conclusions.
Data Analysis in Qualitative Research
Data analysis in qualitative research involves examining non-numeric data to understand concepts, opinions, and experiences. It is an iterative and non-linear process, offering deep insights into complex phenomena.
Tools for Data Analysis in Qualitative Research
Various tools assist researchers in analyzing qualitative data, facilitating the organization, coding, and thematic analysis of text and multimedia data.
- NVivo: Enables researchers to manage and analyze qualitative data from interviews, articles, and journals.
- ATLAS.ti: Provides powerful tools for coding and mapping text data relationships.
- MAXQDA: Supports qualitative, quantitative, and mixed methods data analysis.
Coding: The process of categorizing qualitative data by assigning codes or labels, enabling themes to emerge.
For instance, when analyzing interview transcripts, coding might involve tagging sentences related to 'stress factors' with a specific code.
Many qualitative analysis tools offer visualization features, such as word clouds, to help identify frequent themes quickly.
Approaches for Data Interpretation
Qualitative data interpretation involves making sense of coded data to answer research questions. Several approaches can be applied:
- Grounded Theory: Develops theories based on data collected, suitable for exploratory studies.
- Thematic Analysis: Identifies themes or patterns within the data, emphasizing what is common across a data set.
- Content Analysis: Quantifies the presence of certain themes objectively, providing a systematic insight.
In a deeper exploration of thematic analysis, researchers go beyond just coding text. They iteratively refine and organize codes into themes and sub-themes, emphasizing both manifest content (directly observable) and latent content (underlying meaning). This method allows researchers to look for patterns across data by constantly comparing sections of text.
Case Studies: Qualitative Data Analysis
Case studies in qualitative research involve detailed, in-depth analysis of a specific case or cases, offering enriched insights. They often utilize multiple data sources like interviews, observations, and documents to form a comprehensive understanding.
- Single-case studies provide profound insights into a unique phenomenon.
- Multiple-case studies permit comparative analysis across different settings.
- Intrinsic case studies aim to understand the case itself, whereas instrumental case studies examine broader issues through the case.
An example of a case study approach is examining a specific community's response to a public health initiative, utilizing interviews and observation to gather diverse perspectives.
Triangulation, or using multiple data sources and methods, enhances the credibility and depth of a case study analysis.
Systematic Reviews and Meta-Analysis Techniques
In the medical field, systematic reviews and meta-analysis techniques are fundamental for synthesizing research evidence. They enhance the quality of clinical decisions by providing comprehensive and reliable summaries of current knowledge.
Systematic Reviews in Medicine: Steps and Protocols
Systematic reviews in medicine are characterized by rigorous protocols and structured steps to ensure thoroughness and minimize bias. These steps are:
- Formulating the Research Question: Define a clear and specific question based on the PICO (Population, Intervention, Comparison, Outcome) framework.
- Developing a Protocol: Plan the methods before conducting the review to enhance transparency and reproducibility.
- Comprehensive Literature Search: Conduct systematic searches across multiple databases to ensure all relevant studies are included.
- Study Selection: Apply predefined inclusion and exclusion criteria to identify eligible studies.
- Data Extraction and Quality Assessment: Extract data using standardized forms and assess study quality using tools like the Cochrane Risk of Bias Tool.
- Data Synthesis: Qualitatively or quantitatively summarize findings, often leading to meta-analysis.
Developing a protocol in advance minimizes the influence of bias during the review process.
A systematic review of randomized controlled trials (RCTs) may use a protocol to ensure consistent identification and evaluation of studies comparing the efficacy of different antihypertensive medications in reducing blood pressure.
Comparing Systematic Reviews and Meta-analysis
While systematic reviews and meta-analyses are closely related, they serve somewhat different roles in research synthesis. A systematic review identifies and evaluates all relevant research on a certain topic, providing a qualitative overview. In contrast, a meta-analysis statistically combines data from multiple studies, producing a quantitatively synthesized result.Key differences include:
- Systematic reviews may not always include a meta-analysis, particularly if studies are too heterogeneous.
- Meta-analysis provides a single statistical measure (e.g., an effect size) reflecting the strength of evidence.
- Systematic reviews offer more comprehensive insights, including context, quality, and study characteristics.
For example, a meta-analysis could calculate a combined effect size using the formula: \[\text{Effect Size} = \frac{\sum \left( w_i \times x_i \right)}{\sum w_i}\] where \( w_i \) is the weight for each study, and \( x_i \) is the effect size of the individual study.
A deeper examination of meta-analytical methods reveals diverse approaches for pooling data, such as fixed-effect and random-effects models. Fixed-effect models assume that one true effect size underlies all studies, while random-effects models account for variability by assuming that effect sizes vary between studies. The random-effects model is particularly useful when study populations or interventions are expected to differ across trials.
Tools for Conducting Meta-analysis in Medical Research
Conducting a meta-analysis requires specific tools that streamline data collection, analysis, and visualization. These tools are integral for managing the complex task of aggregating and analyzing large datasets:
- RevMan: Developed by Cochrane, this software supports the entire review process, including writing protocols and performing meta-analyses.
- Comprehensive Meta-Analysis (CMA): Offers extensive features for handling different types of data and effect sizes.
- MedCalc: Facilitates statistical calculations and graphical displays for meta-analysis.
- Stata and R Packages (e.g., metafor, meta): Provide powerful programming solutions for custom analyses.
Research & Analysis - Key takeaways
- Research & Analysis: Key components in medical science used for evidence-based practice and decision-making include various methods and tools to ensure reliability and validity.
- Meta-analysis in Medical Research: Combines data from multiple studies to enhance statistical power and precision, involving steps like defining research questions, searching and selecting studies, and interpreting results.
- Systematic Reviews in Medicine: Comprehensive literature reviews focused on specific medical questions, summarizing evidence, featuring thorough search strategies, and critical appraisals.
- Epidemiological Studies in Medicine: Studies that analyze health patterns and inform public health policy, involving designs like cross-sectional, cohort, and case-control studies to identify disease causes and prevention.
- Data Analysis in Qualitative Research: Involves examining non-numeric data using tools like NVivo and ATLAS.ti to identify themes, with methods like grounded theory and thematic analysis, for deep insights into research data.
- Medical Research Ethics: Principles guiding ethical research conduct in medicine, ensuring integrity and participant protection, focusing on informed consent, minimization of harm, and equitable benefit distribution.
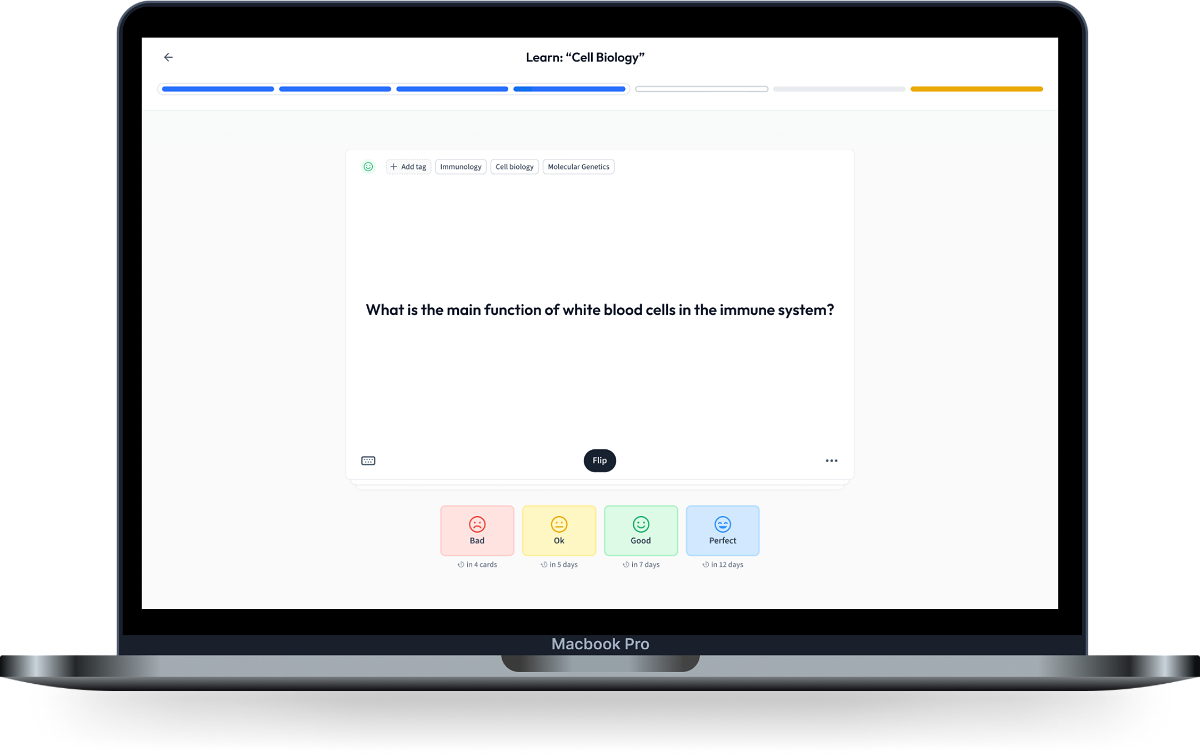
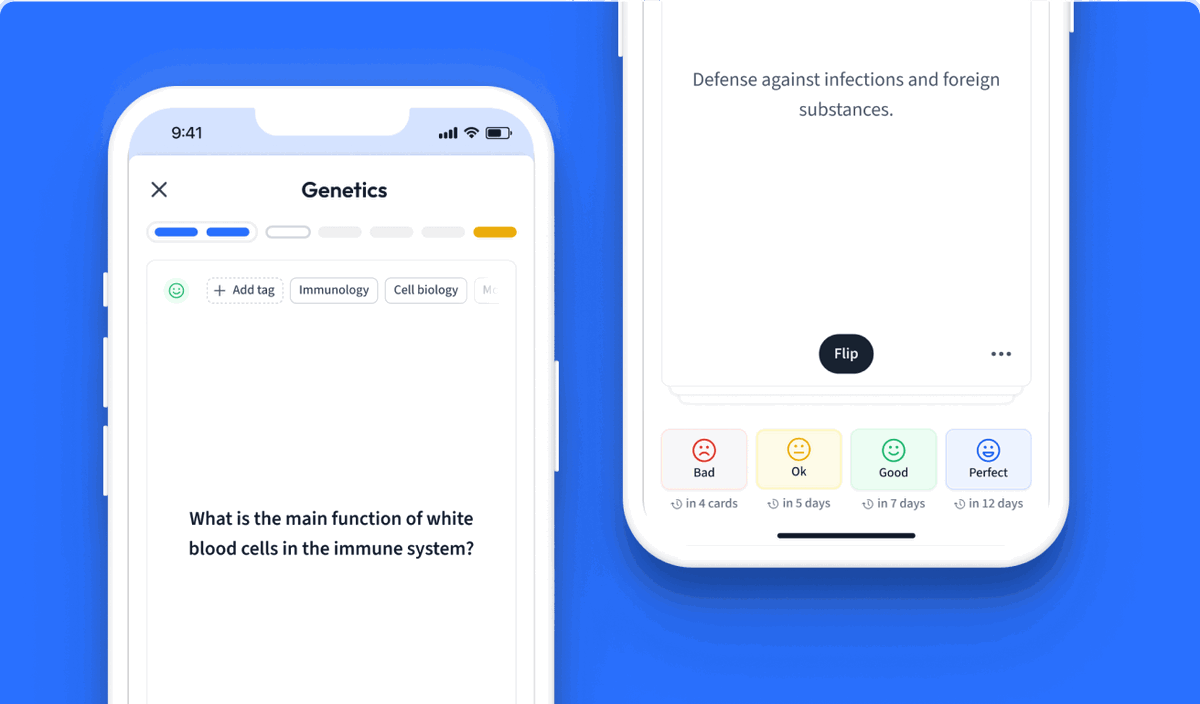
Learn with 12 Research & Analysis flashcards in the free StudySmarter app
We have 14,000 flashcards about Dynamic Landscapes.
Already have an account? Log in
Frequently Asked Questions about Research & Analysis
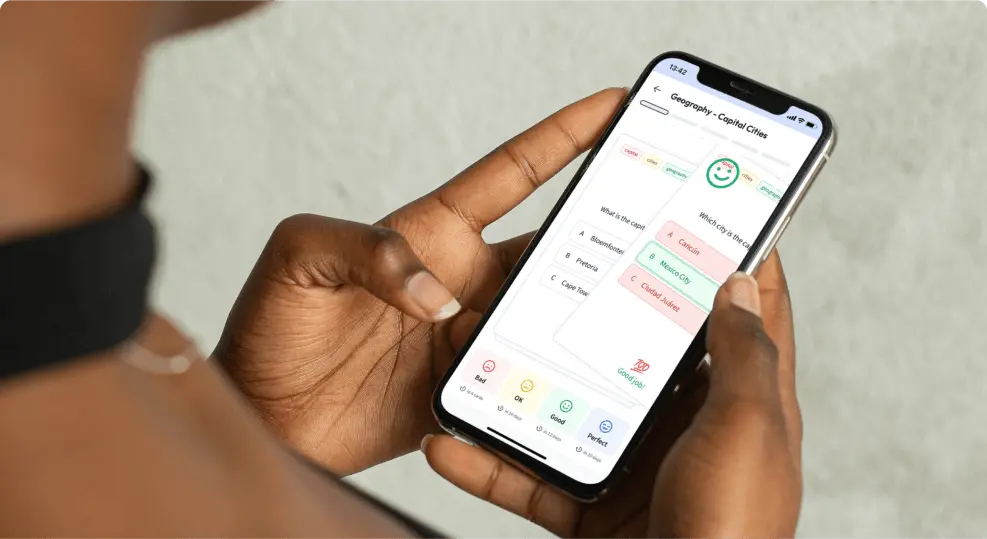
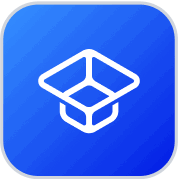
About StudySmarter
StudySmarter is a globally recognized educational technology company, offering a holistic learning platform designed for students of all ages and educational levels. Our platform provides learning support for a wide range of subjects, including STEM, Social Sciences, and Languages and also helps students to successfully master various tests and exams worldwide, such as GCSE, A Level, SAT, ACT, Abitur, and more. We offer an extensive library of learning materials, including interactive flashcards, comprehensive textbook solutions, and detailed explanations. The cutting-edge technology and tools we provide help students create their own learning materials. StudySmarter’s content is not only expert-verified but also regularly updated to ensure accuracy and relevance.
Learn more