Jump to a key chapter
Definition of Epidemiological Modeling
Epidemiological Modeling is a crucial tool used in public health to study the spread of diseases within populations. Through the use of mathematical and statistical techniques, these models aim to predict the progression of disease outbreaks and propose strategies to contain them.
What is Epidemiological Modeling?
Epidemiological modeling involves creating representations of how infectious diseases spread. These models can help you understand potential outcomes and make decisions regarding healthcare interventions. They typically consist of mathematical equations and simulations that replicate real-world scenarios. There are several types of epidemiological models, including:
- Deterministic models: These use fixed inputs and provide a specific outcome. They focus on average behaviors rather than individual variations.
- Stochastic models: These incorporate random variations to mimic real-world unpredictability in disease spread.
- Compartmental models: Simplify the population into compartments like \textbf{S}usceptible, \textbf{I}nfected, and \textbf{R}ecovered (SIR models) to simulate the flow of individuals between different states.
Epidemiological Modeling is defined as the use of mathematical, statistical, and computational techniques to predict the spread of diseases in human populations and implement control strategies.
An example of a basic epidemiological model is the SIR model. This model categorizes a population into three groups: Susceptible (S), Infected (I), and Recovered (R). The transitions between these states can be represented by the following differential equations:\[\frac{dS}{dt} = -\beta SI\]\[\frac{dI}{dt} = \beta SI - \gamma I\]\[\frac{dR}{dt} = \gamma I\]where \( \beta \) is the transmission rate and \( \gamma \) is the recovery rate.
In epidemiological modeling, the basic reproduction number, \( R_0 \), is a crucial metric. It represents the expected number of secondary infections from a single infection. When \( R_0 > 1 \), the infection will likely spread; when \( R_0 < 1 \), it will likely die out.
Deterministic models are crucial in simplified disease spread assumptions but lack real-world unpredictability. For instance, in such models, the progression of the disease is determined completely by the parameters with no randomness involved. This makes them suitable for large populations where individual variations average out. However, stochastic models, which include randomness and are better for smaller populations or when more precision is needed, can sometimes provide more accurate predictions. For example, a stochastic version of the SIR model considers uncertainties in contacts and disease outcomes. This involves probabilities instead of fixed rates, adding more complexity and accuracy to predictions:\[P_{\text{Susceptible}} = P_s\]\[P_{\text{Infected}} = 1 - (1 - P_s)^{\text{contact rate} \times I}\]Here, P stands for probability, giving a more nuanced view of how epidemics could progress.In complex scenarios, these models become critical in policy-making, allowing governments to simulate potential interventions and their effectiveness.
Types of Epidemiological Models
Epidemiological models come in various forms, each designed to explain the intricacies of disease spread. Understanding these types is vital to grasp the impact and control of diseases in populations.
Deterministic Models
Deterministic models in epidemiology are based on fixed variables and provide consistent outcomes given the same initial parameters. They work on average population behavior, assuming that every individual in the population behaves the same way.These models are relatively simple and are effective for large population studies where random fluctuations are negligible. Transition rates between states are expressed with differential equations, such as the SIR model:\[\frac{dS}{dt} = -\beta SI\]\[\frac{dI}{dt} = \beta SI - \gamma I\]\[\frac{dR}{dt} = \gamma I\]where \( \beta \) represents the transmission rate and \( \gamma \) the recovery rate.
A Deterministic Model is a type of epidemiological model where outcomes are fully determined by a set of given parameters without randomness.
Stochastic Models
Stochastic models differ from deterministic ones by incorporating elements of randomness, making them suitable for small populations or more complex scenarios.In these models, parameters such as infection rates might vary based on probability distributions. This accounts for natural variability and uncertainty in disease spread. An example of such a model is a stochastic version of the SIR model where transition probabilities replace fixed rates:
- Probabilities consider random contact and infection events.
- These models are useful for modeling rare events or outbreaks in small communities.
Consider a stochastic model that simulates an outbreak in a small town. The variability of disease spread can result in outcomes ranging from full containment to widespread infection, depending on probabilities set for infection and recovery.Stochastic simulations often run multiple iterations to see various potential outcomes, allowing for a better comprehension of potential risks. Such insights are crucial for planning healthcare responses and informing policy decisions.
Compartmental Models
Compartmental models are essential in breaking down the population into distinct categories or compartments like Susceptible (S), Infected (I), and Recovered (R). Each group interacts based on defined rules, allowing epidemiologists to understand the flow of population across these compartments.Commonly used compartmental models include:
- SIR Model: Tracks movement among Susceptible, Infected, and Recovered groups.
- SIS Model: Accounts for possible reinfection after recovery.
- SEIR Model: Adds an Exposed category for latency periods before infection.
In an SEIR model, an extra compartment for 'Exposed' allows for modeling diseases with incubation periods. Here are the equations governing transitions:\[\frac{dS}{dt} = -\beta SE\]\[\frac{dE}{dt} = \beta SE - \sigma E\]\[\frac{dI}{dt} = \sigma E - \gamma I\]\[\frac{dR}{dt} = \gamma I\]where \( \sigma \) is the rate at which exposed individuals become infectious.
Understanding the basic reproduction number, \( R_0 \), in these models is crucial. It reflects the number of new infections caused by an infectious individual. When \( R_0 \) is greater than 1, an outbreak is likely.
Techniques in Epidemiological Modeling
Epidemiological modeling employs various techniques to study the spread and control of diseases. Each technique is chosen based on specific goals and population characteristics.
Compartmental Models
Compartmental models divide populations into compartments, such as Susceptible (S), Infected (I), and Recovered (R), to simulate the flow of individuals through these stages. This method captures the dynamics of disease progression clearly and concisely.In a typical SIR model, these compartments interact through defined rates:
- Transition from S to I determined by the transmission rate \( \beta \).
- Transition from I to R determined by the recovery rate \( \gamma \).
Consider an SEIR model, which adds an 'Exposed' compartment to account for an incubation period. The state changes are driven by the following equations:\[\frac{dS}{dt} = -\beta SE\]\[\frac{dE}{dt} = \beta SE - \sigma E\]\[\frac{dI}{dt} = \sigma E - \gamma I\]\[\frac{dR}{dt} = \gamma I\]where \( \sigma \) is the rate at which exposed individuals become infectious.
Agent-Based Models
Agent-Based Models (ABMs) simulate interactions of autonomous agents to study complex phenomena. In epidemiology, each agent represents an individual with specific attributes and behaviors that affect disease spread. These models offer granular insights by capturing individual-level variation.ABMs use rules-based simulation rather than equations, allowing for complex, non-linear interactions among the agents. This includes:
- Simulating social networks to reflect real-contact patterns.
- Incorporating stochastic events in disease transmission.
- Adjusting individual compliance with interventions.
Agent-Based Models in epidemiology are powerful due to their ability to simulate heterogeneity within the population. For example, varying infection probabilities based on age, social exposure, and pre-existing conditions provide a more nuanced understanding of epidemic evolution.A study of flu transmission using an agent-based model can simulate the effect of school closures or public mask-wearing. Each decision alters individual behaviors and thus changes the overall disease dynamics in a community.
Network Models
Network models analyze disease spread through networks representing connections between individuals. These models are particularly useful for sexually transmitted infections or respiratory illnesses, where physical or social networks influence disease transmission.In network models, nodes represent individuals, and edges represent interactions or potential transmission pathways. Key features include:
- Degree distribution reflecting the number of connections per individual.
- Clustering coefficient indicating the propensity of nodes to cluster together.
- Path lengths measuring shortest paths between nodes, useful for understanding speed of spread.
In network models, understanding the structure of a network (such as its density or centrality measures) can help identify key individuals or groups for targeted interventions.
Applications of Epidemiological Models
Epidemiological models are vital in understanding and controlling the spread of diseases. They serve numerous applications, from predicting outbreak patterns to evaluating interventions and informing public health policies. These models provide insights into the effectiveness of different strategies to mitigate the impact of infectious diseases.
Epidemiological Modeling Examples
Epidemiological models can be used to simulate various scenarios and outcomes. Here are some examples:
Epidemiological Modeling involves the use of mathematical equations and computational tools to simulate the transmission dynamics of infectious diseases in populations.
A well-known example is the application of the SIR model during the COVID-19 pandemic. This model helped predict the number of active infections and evaluate the potential effects of interventions like social distancing and vaccination campaigns:\[\frac{dS}{dt} = -\beta SI\]\[\frac{dI}{dt} = \beta SI - \gamma I\]\[\frac{dR}{dt} = \gamma I\]In this context, \( \beta \) and \( \gamma \) were adjusted based on real-world data to reflect restrictions and population immunity.
Another remarkable application is the use of network models to analyze the spread of sexually transmitted infections. These models create networks where individuals, represented as nodes, are connected based on their relationships. This allows researchers to:
- Identify key individuals or groups, also known as 'super-spreaders', who significantly contribute to transmission.
- Understand how different network structures, such as densely connected groups, can affect outbreak dynamics.
- Explore targeted intervention strategies, like partner notification and treatment, to reduce transmission.
Epidemiological models can also incorporate spatial components to explore how diseases spread across different geographical regions, enhancing the precision of intervention planning.
epidemiological modeling - Key takeaways
- Epidemiological Modeling: A tool in public health using mathematical and statistical techniques to predict disease spread and propose containment strategies.
- Types of Epidemiological Models: Includes deterministic models (fixed inputs), stochastic models (random variations), and compartmental models (different states like SIR).
- Techniques in Epidemiological Modeling: Utilizes compartmental models, agent-based models, and network models to study disease progression.
- Deterministic vs. Stochastic Models: Deterministic models provide specific outcomes with fixed parameters, while stochastic models incorporate randomness to account for real-world variability.
- Epidemiological Modeling Examples: Examples include the SIR model and network models used to study diseases like COVID-19 and sexually transmitted infections, respectively.
- Applications of Epidemiological Models: Used for predicting outbreaks, evaluating interventions (e.g., social distancing, vaccinations), and informing public health policies.
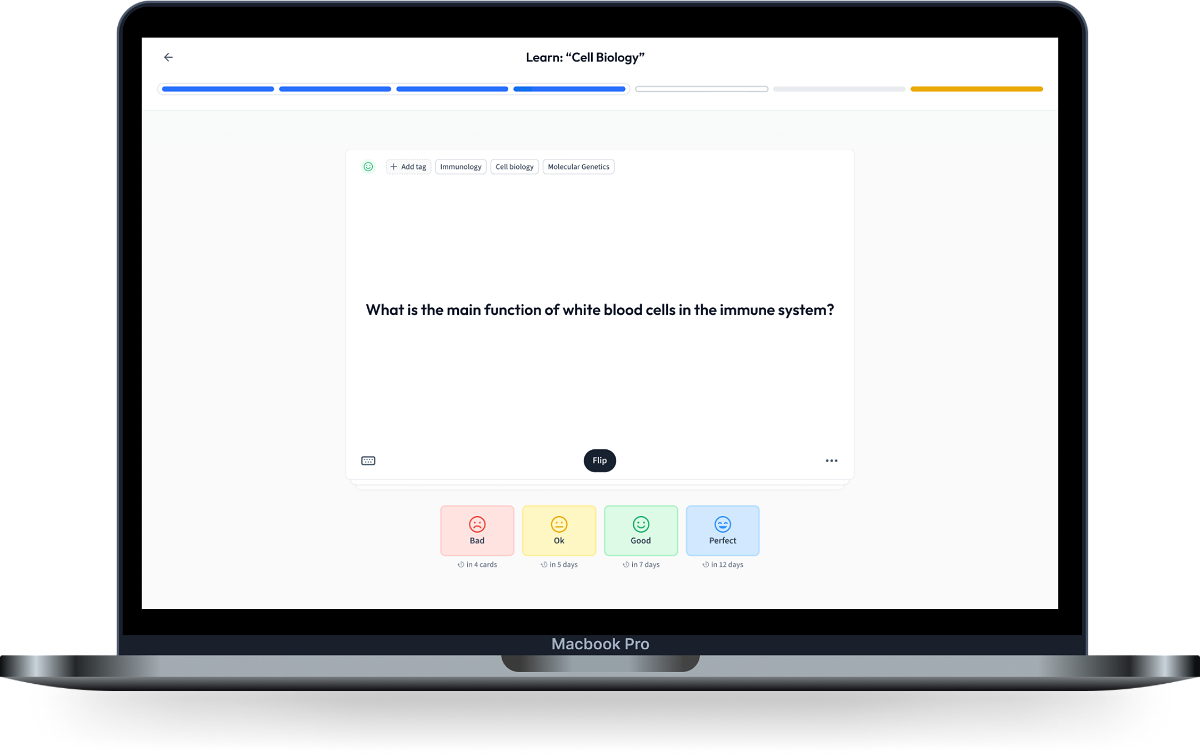
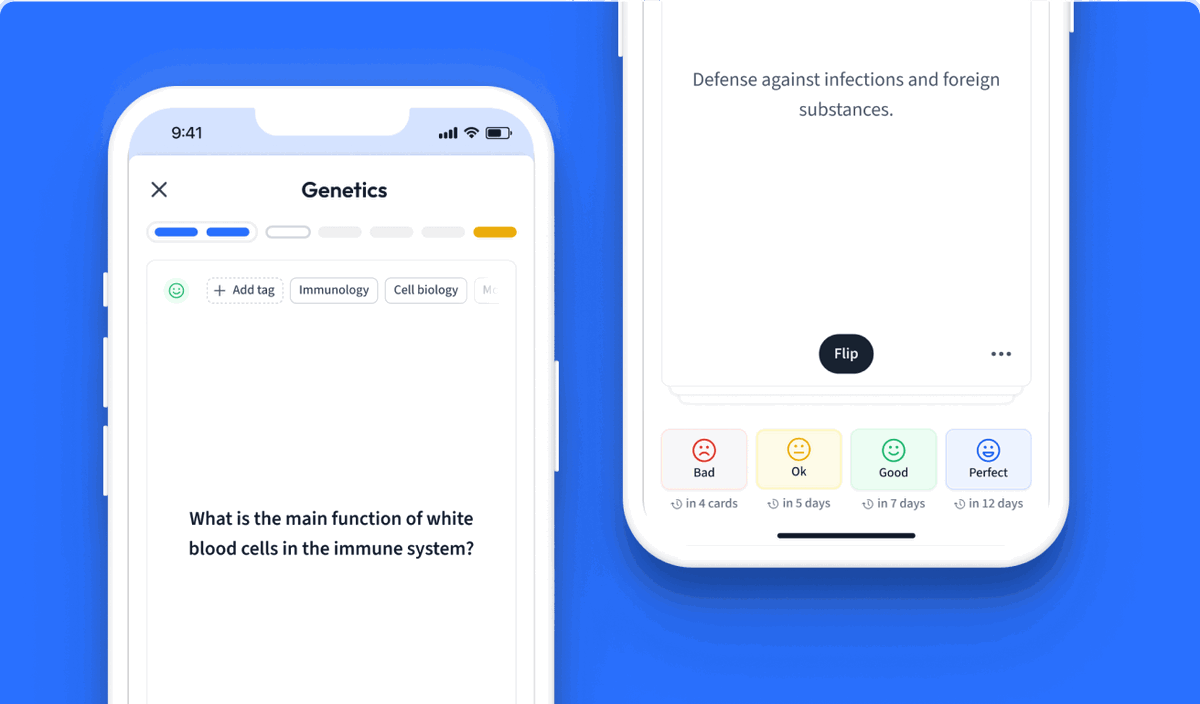
Learn with 12 epidemiological modeling flashcards in the free StudySmarter app
We have 14,000 flashcards about Dynamic Landscapes.
Already have an account? Log in
Frequently Asked Questions about epidemiological modeling
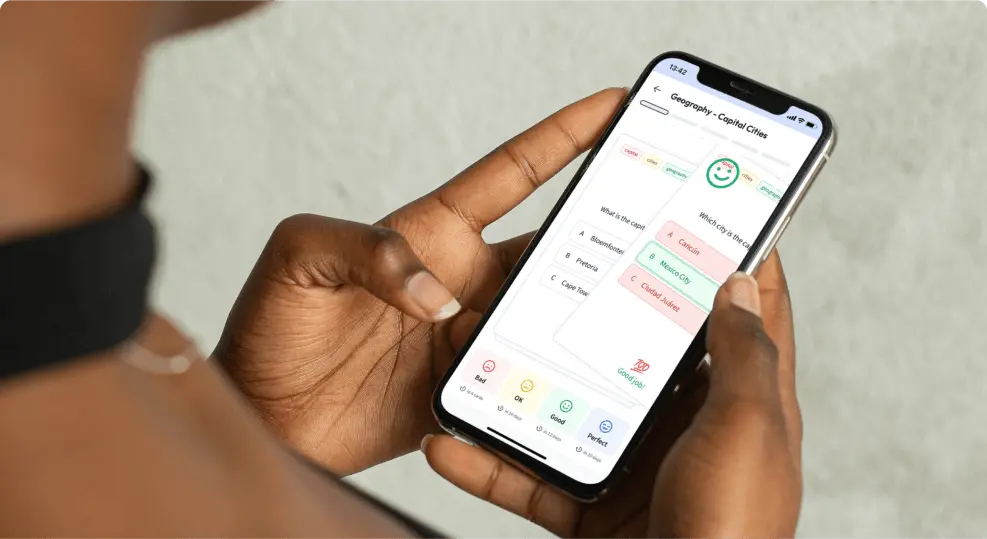
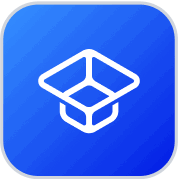
About StudySmarter
StudySmarter is a globally recognized educational technology company, offering a holistic learning platform designed for students of all ages and educational levels. Our platform provides learning support for a wide range of subjects, including STEM, Social Sciences, and Languages and also helps students to successfully master various tests and exams worldwide, such as GCSE, A Level, SAT, ACT, Abitur, and more. We offer an extensive library of learning materials, including interactive flashcards, comprehensive textbook solutions, and detailed explanations. The cutting-edge technology and tools we provide help students create their own learning materials. StudySmarter’s content is not only expert-verified but also regularly updated to ensure accuracy and relevance.
Learn more