Jump to a key chapter
Infectious Diseases Epidemiology Explained
Infectious diseases epidemiology is a critical field that focuses on understanding diseases that can spread from one person to another. It plays a vital role in public health by helping experts plan and evaluate strategies to stem the tide of infections.
Definition of Infectious Disease Epidemiology
Infectious Disease Epidemiology is the study of the spread, control, and prevention of diseases caused by infectious agents.
Infectious diseases are caused by pathogens such as bacteria, viruses, fungi, or parasites. These diseases may spread directly or indirectly from person to person or through environmental sources.The field encompasses:
- Identifying the source and mode of transmission
- Tracking disease outbreaks
- Implementing control measures
- Assessing vaccine efficacy
The study involves complex mathematical models to predict disease spread. For instance, susceptible-infectious-recovered (SIR) models can be described using differential equations such as:\[\frac{dS}{dt} = -\beta SI\]\[\frac{dI}{dt} = \beta SI - \gamma I\]\[\frac{dR}{dt} = \gamma I\]where
- S is the number of susceptible individuals
- I is the number of infectious individuals
- R is the number of recovered individuals
- \beta is the transmission rate
- \gamma is the recovery rate
Basic Infectious Disease Concepts in Epidemiology
The core concepts in infectious diseases epidemiology help us understand how diseases spread and can be controlled using mathematical and statistical tools.
The basic reproductive number, denoted as \(R_0\), is a crucial metric for gauging how contagious an infectious disease is. It represents the average number of secondary cases produced by a single infection in a fully susceptible population.
Transmission Dynamics is a fundamental concept in infectious disease epidemiology. It includes the following points:
- R_0 (Basic Reproductive Number): Indicates how many people, on average, an infected person will infect in a fully susceptible population. An \(R_0\) greater than 1 suggests that an outbreak can occur.
- Herd Immunity: The resistance of a group to infection due to immunity of a large proportion of the members.
- Attack Rate: The proportion of people who become ill in a specific population over a certain time period.
Consider a simple example:Suppose in a community of 100 people, one individual with a highly contagious virus (with an \(R_0\) of 4) enters. Without intervention, an average of 4 others will be infected by that one person. This number could represent:
- Individual behavioral changes
- Vaccine development
- Environmental alterations to slow the spread
Understanding Epidemiology of Infectious Diseases
Epidemiology of infectious diseases involves the study of factors that determine the spread and control of diseases in populations. It is an essential branch of public health that aids in developing strategies to minimize infections and improve health outcomes globally.
Techniques in Infectious Disease Epidemiology
Epidemiological techniques are employed to assess and curb the spread of infectious diseases. Various methodologies delve into various aspects, from baseline investigations to advanced predictive models.
Epidemiologic Methods: These are systematic approaches used to investigate health events, understand disease distribution, and implement preventive interventions.
Key techniques in infectious disease epidemiology include:
- Surveillance: Ongoing collection, analysis, and interpretation of health data. Early detection through surveillance enables quick action against outbreaks.
- Field Investigations: Involves studying outbreaks, often by determining causes, sources, and opportunities to intervene.
- Analytical Studies: These determine risk factors and evaluate associations between exposures and disease. Often use case-control and cohort study designs.
- Mathematical Modeling: Utilizes systems of equations to predict future outbreaks and assess the potential effectiveness of interventions. One such model employs the differential equations:\[\frac{dS}{dt} = -\beta SI\]\[\frac{dI}{dt} = \beta SI - \gamma I\]\[\frac{dR}{dt} = \gamma I\]
Consider an outbreak of an influenza-like illness in a community. Surveillance identifies a sharp increase in cases.The epidemiology team then conducts field investigations, including testing respiratory samples and interviewing affected individuals to identify common exposures. These techniques help establish the illness's contagiousness and guide public health responses.
Advanced mathematical models may simulate various scenarios to forecast infectious disease spread, accounting for different intervention strategies. For example, using scenario analysis, these models can evaluate:
- The impact of travel restrictions.
- Potential success of vaccination efforts with different efficacies.
- Probable results of social distancing and masking protocols.
Applied Infectious Disease Epidemiology
Applied infectious disease epidemiology translates research outcomes into practical strategies to handle disease on the ground. It integrates expertise gained through core epidemiological methods with policy development and application.
Applied epidemiology often involves collaboration between diverse healthcare professionals, public health planners, and governmental bodies to ensure comprehensive health response actions.
When applying epidemiological findings, consider the following:
- Policy Development: Creating guidelines based on the latest evidence to prevent and control disease spread.
- Health Communication: Proper messaging to educate and inform the public and promote health behaviors.
- Cross-sector Collaboration: Involves working with different sectors such as education, law enforcement, and private enterprises to enhance public health response.
During the COVID-19 pandemic, applied infectious disease epidemiology saw practical manifestation in:
- Developing and distributing vaccines based on epidemiological data.
- Designing communication campaigns about social distancing and mask usage.
- Utilizing mobile applications to track contacts and alert users of potential exposure.
Techniques in Infectious Disease Epidemiology
Techniques in infectious disease epidemiology are essential for understanding and controlling disease spread. These techniques include data collection, statistical analysis, and predictive modeling to target interventions effectively.
Modern Techniques in Infectious Disease Epidemiology
Modern epidemiological techniques have evolved to incorporate advanced technologies and innovative approaches to combat infectious diseases. They enable real-time monitoring and provide precise data to track the progress of interventions.
Real-time Surveillance: A continuous data collection method that provides immediate information about disease spread, allowing for quick response strategies.
Key modern techniques include:
- Gene Sequencing: Identifies pathogens at the molecular level, facilitating precise tracking of strains and mutations.
- Modeling and Simulation: Predicts potential disease outbreaks using computational models. This involves equations like the basic Susceptible-Infectious-Recovered (SIR) model:\[\frac{dS}{dt} = -\beta SI\]\[\frac{dI}{dt} = \beta SI - \gamma I\]\[\frac{dR}{dt} = \gamma I\]
- Data Mining: Analyzes large data sets to identify patterns and trends in disease spread.
Genomic data from gene sequencing can now be shared globally at unprecedented speeds, allowing scientists worldwide to collaborate in the fight against infectious diseases.
In a recent viral outbreak, real-time surveillance systems used mobile technology to quickly identify and isolate new cases. The integration of GPS data helped track the movements of potentially infected individuals, curbing the disease spread.
Simulations generated from infectious disease modeling can use sophisticated methods such as agent-based modeling. These models consider individual behavior and interactions within populations to simulate disease dynamics. For example, they may:
- Account for human behavior patterns and cultural differences across regions.
- Incorporate vaccination and treatment options to study outcomes under various scenarios.
- Analyze economic impacts and costs of interventions.
Data Analysis in Infection Studies
Data analysis in infection studies involves scrutinizing numerous data points to understand disease patterns and inform public health strategies. It encompasses statistical methods and the use of computational tools to interpret complex data sets efficiently.
Statistical Analysis: Utilizes mathematical techniques to examine data and draw meaningful conclusions about the relationship between variables and disease spread.
Key components of data analysis include:
- Descriptive Statistics: Provides basic summaries of data, such as mean, median, and standard deviation, to understand disease characteristics.
- Inferential Statistics: Allows predictions and generalizations about a population based on a sample. Techniques like regression analysis are commonly used to identify key risk factors.For example, logistic regression can model the probability of infection occurring based on exposure variables:\[\log\left(\frac{p}{1-p}\right) = \beta_0 + \beta_1X_1 + \beta_2X_2 + \ldots + \beta_nX_n\]where \(p\) is the probability of infection, \(X_1, X_2, \ldots, X_n\) are predictors, and \(\beta_i\) are coefficients.
- Spatial Analysis: Examines geographical patterns of disease spread and identifies hotspots.
Spatial analysis can be enhanced using Geographic Information Systems (GIS) that integrate data from various sources to provide comprehensive spatial insights.
In controlling Dengue fever outbreaks, data analysis revealed that certain climatic factors, such as temperature and humidity, significantly influence mosquito breeding and virus transmission. By using regression models, researchers predicted outbreaks based on these environmental indicators. This analysis allowed targeted control measures and public alerts in high-risk areas.
Applied Infectious Disease Epidemiology in Public Health
Infectious disease epidemiology is pivotal in public health by providing tools and knowledge necessary to tackle disease outbreaks. Its application spans from local to global scales, influencing policy-making and enhancing population health management.
Real-World Applications of Infectious Disease Epidemiology
Infectious disease epidemiology is instrumental in shaping responses to health threats. By applying theories and models, you can anticipate and mitigate disease spread efficiently.Applications include:
- Outbreak Investigation: Identifies the source and control measures of infectious diseases.
- Vaccine Development: Epidemiological studies assess vaccine effectiveness and establish vaccination strategies.
- Policy Formulation: Epidemiological data guides health policies, including quarantine measures and travel restrictions.
In the H1N1 influenza pandemic (2009), rapid epidemiological assessment identified spread patterns, significantly aiding in the distribution of antiviral medications and initiation of vaccination efforts. Mathematical models predicted hospital surge capacities, ensuring preparedness in healthcare facilities.
Predictive models in epidemiology aren't only theoretical—they often incorporate real-time data, enhancing accuracy and intervention outcomes.
Case Studies in Applied Infectious Disease Epidemiology
Real-life case studies exemplify the impact of applied infectious disease epidemiology. These scenarios highlight the collaboration between epidemiologists, public health professionals, and policy-makers.Case Study Examples:
- Ebola Virus Outbreak (West Africa, 2014-2016):Epidemiologists played a key role in understanding Ebola's transmission. By mapping cases, they identified hot zones and implemented targeted interventions. Mathematical modeling projected infection trends, informing resource allocation and quarantine policies.
- Zika Virus (2015-2016):Epidemiological research discovered the link between the virus and birth defects. Quick data sharing and international collaboration produced guidelines to reduce transmission—particularly among pregnant women—by advising travel and promoting mosquito control.
Analyzing infectious disease situations involves a deep dive into data and model interpretation. For example, during the COVID-19 pandemic, epidemiologists utilized complex models to comprehend the virus's impact on healthcare systems and formulate control strategies.Utilizing advanced models such as compartmental and agent-based models helped public health officials develop strategies for:
- Vaccination distribution considering population density and vulnerability.
- Estimating the effects of social and physical distancing measures.
- Anticipating healthcare resource needs through forecasting ICU bed availability.
infectious diseases epidemiology - Key takeaways
- Definition of Infectious Disease Epidemiology: Study of spread, control, and prevention of diseases caused by infectious agents.
- Basic Infectious Disease Concepts: Includes terms like R0 (basic reproductive number), herd immunity, and attack rate to understand transmission dynamics.
- Techniques in Infectious Disease Epidemiology: Surveillance, field investigations, analytical studies, and mathematical modeling to predict and control outbreaks.
- Applied Infectious Disease Epidemiology: Integrates epidemiological research with public health strategies and policy development to manage outbreaks.
- Modern Techniques: Real-time surveillance, gene sequencing, and data mining enhance understanding and control of diseases.
- Data Analysis in Infection Studies: Involves statistical analysis, spatial analysis, and computational tools to interpret epidemiological data.
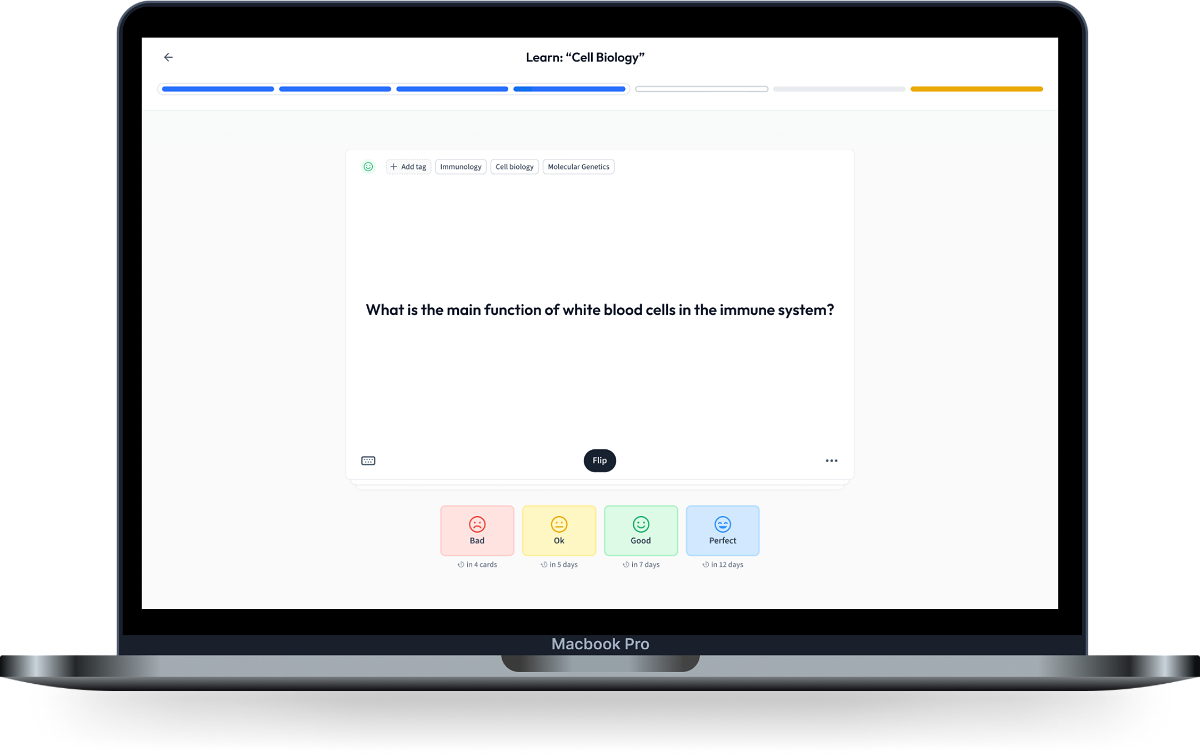
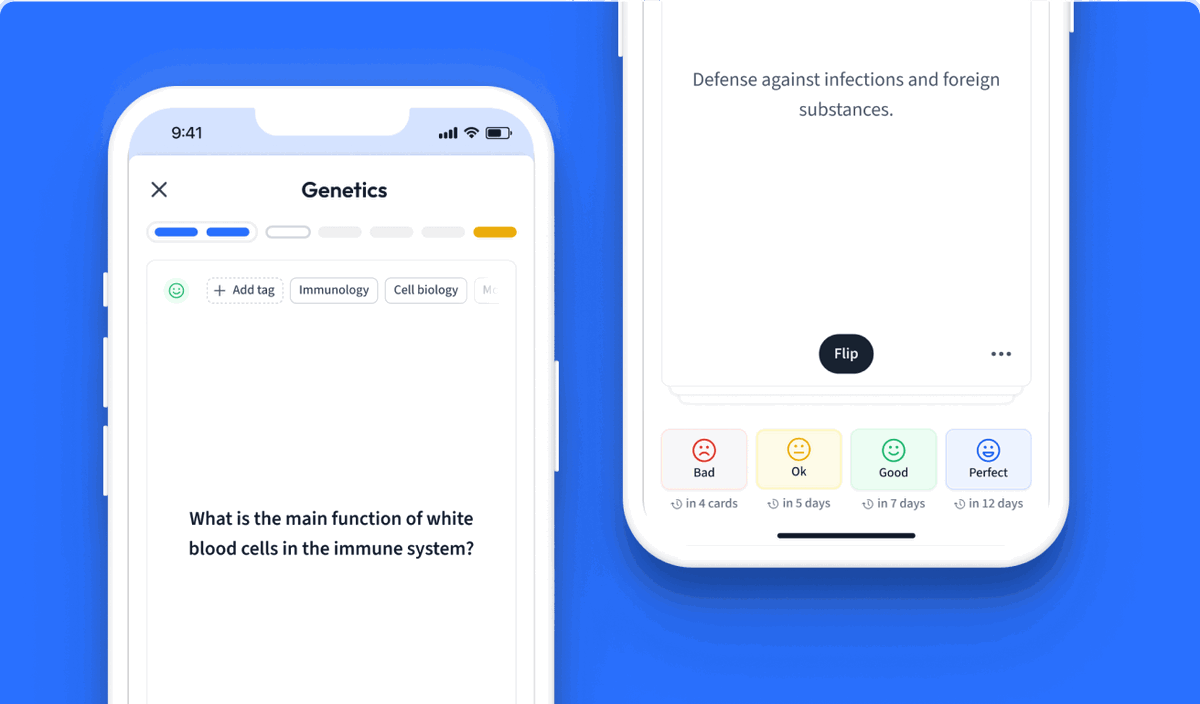
Learn with 12 infectious diseases epidemiology flashcards in the free StudySmarter app
We have 14,000 flashcards about Dynamic Landscapes.
Already have an account? Log in
Frequently Asked Questions about infectious diseases epidemiology
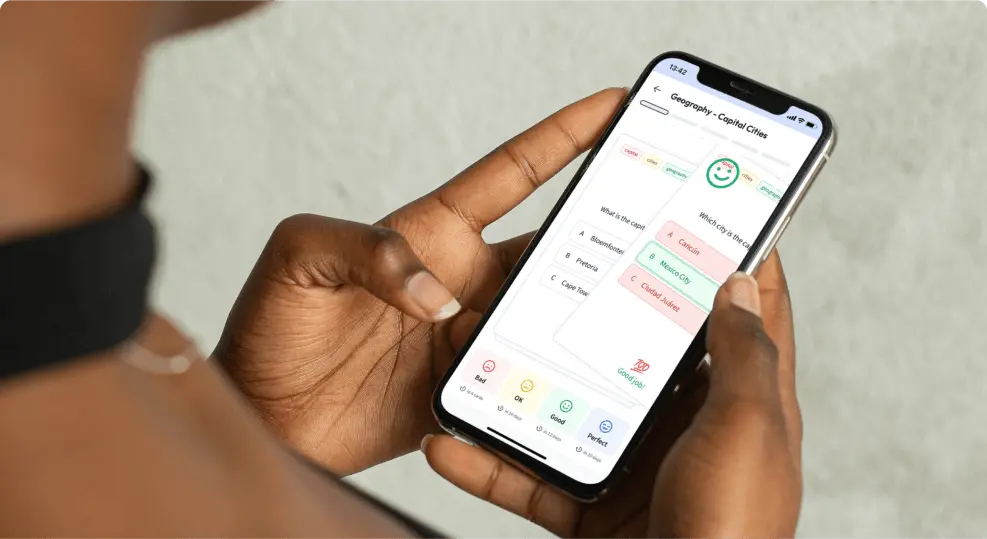
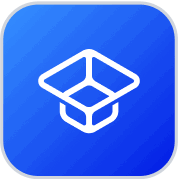
About StudySmarter
StudySmarter is a globally recognized educational technology company, offering a holistic learning platform designed for students of all ages and educational levels. Our platform provides learning support for a wide range of subjects, including STEM, Social Sciences, and Languages and also helps students to successfully master various tests and exams worldwide, such as GCSE, A Level, SAT, ACT, Abitur, and more. We offer an extensive library of learning materials, including interactive flashcards, comprehensive textbook solutions, and detailed explanations. The cutting-edge technology and tools we provide help students create their own learning materials. StudySmarter’s content is not only expert-verified but also regularly updated to ensure accuracy and relevance.
Learn more