Jump to a key chapter
Definition of P Value in Medicine
P-value is a statistical measure that helps you understand the significance of your medical study results. It’s crucial for evaluating whether your findings are due to chance or if they reveal something meaningful.
Understanding P-Value in Hypothesis Testing
In medical research, hypothesis testing is a method used to decide whether there is enough evidence to reject a null hypothesis. The p-value plays a central role in this process by quantifying the probability of obtaining results at least as extreme as those observed, assuming that the null hypothesis is true.
A p-value is a number, computed from a statistical test, that provides context on the probability of observing the data equivalently or more extreme if the null hypothesis is true. It's expressed as:
- P(X >= observed | H0)
Suppose you want to test the effect of a new drug on blood pressure. Your null hypothesis (H0) is that the drug does not affect blood pressure. After conducting a clinical trial, you compute a p-value of 0.03. This value indicates that there is only a 3% probability of seeing the effect you observed, or something more extreme, assuming the drug actually has no effect.
A smaller p-value indicates stronger evidence against the null hypothesis. Often, a p-value less than 0.05 is considered significant.
It's important to note that the p-value is not the probability that the null hypothesis is true. Instead, it measures the compatibility of the observed data with the null hypothesis. To grasp its significance, remember:
- A p-value < 0.05 often leads to rejecting the null hypothesis.
- Common thresholds for p-value significance: 0.05, 0.01, and 0.001.
P Value Explained in Medicine
When conducting medical research, understanding the significance of your findings is paramount. The p-value is a tool that helps in interpreting study results by providing a measure of statistical significance. It informs you whether the observed effects are likely genuine or simply due to random chance.
Role of P-Value in Medical Research
P-value is essential in hypothesis testing, serving as a quantifiable metric that assists you in making decisions about the null hypothesis. In simpler terms, a p-value can tell you if the findings from your study give you enough reason to believe that an effect is present.
The p-value is defined mathematically as the probability of observing a test statistic as extreme as the one observed, under the assumption that the null hypothesis is true. It is usually represented as:\( P(TS \, \text{as or more extreme than observed} \, | \, H_0) \)Where TS represents the test statistic.
Consider a scenario where you are testing a new vaccine's effectiveness. Your null hypothesis (H0) might assert that the vaccine does not reduce the incidence of a specific disease. If your test produces a p-value of 0.04, it suggests there's just a 4% chance that the observed reduction in disease incidence is due to random variation, assuming the null hypothesis is valid.
The commonly accepted threshold for p-value significance in medicine is 0.05, meaning a 5% risk of wrongly rejecting the null hypothesis.
While calculating a p-value is crucial, it's equally important to understand what it doesn't tell you. A p-value does not indicate the probability that the null hypothesis is true or false; rather, it describes the probability of the sample data under the assumption that the null hypothesis is correct. A p-value less than 0.05 does not automatically confirm the alternative hypothesis; it suggests that further investigation is warranted.For instance, when dealing with multiple comparisons, raw p-values can be misleading, potentially increasing the likelihood of Type I errors. Accordingly, adjustments such as the Bonferroni correction may be necessary to keep the error rate in check.In addition, even when a result is statistically significant, it may not always be clinically significant. It's important to consider both the magnitude of the effect and its implications in the real-world medical context. Thus, interpreting p-values should always be in conjunction with effect sizes and confidence intervals to provide a comprehensive understanding of the results.
How to Interpret P Value
Understanding how to interpret a p-value is crucial in medical studies. A p-value guides you in determining whether to accept or reject the null hypothesis in your experiment or clinical trial.
Significance of P-Value in Clinical Trials
In clinical trials, the p-value helps ascertain the statistical significance of the results. It offers insights into the likelihood that your findings are not due to random variability. You can better understand the data’s significance by reviewing the calculated p-value in comparison to an accepted threshold level, typically set at 0.05.
The p-value is the probability of obtaining results as extreme as those observed, given that the null hypothesis is true. Mathematically, this can be expressed as:
- \[ P(X \geq \text{observed value} | H_0) \]
Consider a study to test the effectiveness of a new cholesterol-lowering medication. Assume the null hypothesis states that the drug has no effect. After analysis, suppose a p-value of 0.01 is obtained. This result suggests there is only a 1% probability of observing a reduction in cholesterol levels due to chance, leading to the rejection of the null hypothesis.
Remember, a p-value does not measure the size or importance of an effect. It tells you about the strength of evidence against the null hypothesis.
When dealing with complex medical data, interpreting p-values involves understanding both type I and type II errors. A Type I error occurs when you incorrectly reject a true null hypothesis, often controlled by the significance level (commonly 0.05).Consider further factors such as:
- Statistical Power: This refers to the probability of correctly rejecting a false null hypothesis. It's influenced by the sample size and effect size. In formula terms: \[ \text{Power} = 1 - \beta \]
- Multiple Comparisons: When multiple tests are performed, the likelihood of Type I errors increases. Corrections (like Bonferroni correction) adjust p-values accordingly to reduce error rates.
Examples of P Value Interpretation in Medical Research
When you analyze the results of a medical study, the p-value helps determine the statistical significance of your findings. This is particularly valuable when validating the effectiveness of a new treatment or drug. Understanding p-value interpretation not only aids in assessing these results but also informs decisions in clinical practice.
Applying P-Value in Studies on Medication Effectiveness
Imagine you are evaluating the efficacy of a new medication in lowering blood pressure. Your null hypothesis (H0) may be that the medication has no effect on blood pressure levels. Upon testing, you calculate a p-value for the effectiveness of the drug.
A p-value quantifies the probability of observing study results as extreme as the ones obtained if the null hypothesis is true. It's typically expressed as:\[ P(X \geq \text{observed} \, | \, H_0) \]Here, \(X\) represents the test statistic, and \(H_0\) the null hypothesis.
Suppose your test yields a p-value of 0.02. This implies a 2% probability that the reduction in blood pressure observed in your sample could occur due to random chance if the null hypothesis is correct. Because this p-value is lower than the commonly accepted threshold of 0.05, you may reject the null hypothesis, suggesting a significant effect of the medication.
In similar studies, interpreting p-values correctly is crucial for determining the reliability of experimental findings. Utilizing p-values ensures that conclusions drawn are based on sound statistical reasoning.
Incorporating P-Value Interpretation in Clinical Diagnostics
P-value interpretation is equally important in clinical diagnostics when assessing the accuracy of diagnostic tests. Understanding these probabilities helps refine diagnostic criteria and improve patient outcomes.
If a new diagnostic test for a disease results in a p-value of 0.045, this indicates a 4.5% chance that the test’s observed accuracy is a product of random error, under the condition that the diagnostic test is ineffective. A p-value under 0.05 supports the validity of the test.
Though a p-value less than 0.05 is commonly cited as significant, different fields and studies may adjust this threshold based on the context and potential consequences of errors.
Given the complexity of p-value interpretation, consider how other statistical measures work in tandem for a comprehensive analysis:
- Confidence Intervals: Provide a range of values that likely contain the true effect, offering additional context alongside p-values.
- Effect Size: Complements p-values by indicating the magnitude of an observed effect, which can be particularly valuable in medicine where clinical significance is critical.
p-value interpretation - Key takeaways
- P-value interpretation in medicine: A p-value indicates the probability of obtaining results at least as extreme as observed, assuming the null hypothesis is true. It's essential in hypothesis testing.
- Definition of p value in medicine: A statistical measure used to evaluate the significance of medical study results, helping to determine if findings are due to chance.
- Examples of p value interpretation in medical research: A p-value under 0.05 often leads to rejecting the null hypothesis, suggesting a significant effect, considering context like effect size and clinical relevance.
- Interpretation of the p value: A p-value less than 0.05 is commonly deemed significant, indicating strong evidence against the null hypothesis, though clinical significance may need further assessment.
- P value explained in medicine: A tool for determining statistical significance, aiding in whether observed effects are genuine or due to random variations in medical studies.
- How to interpret p value: Compare the p-value to an accepted significance threshold (usually 0.05) to decide whether to reject the null hypothesis, mindful of potential errors and the clinical context.
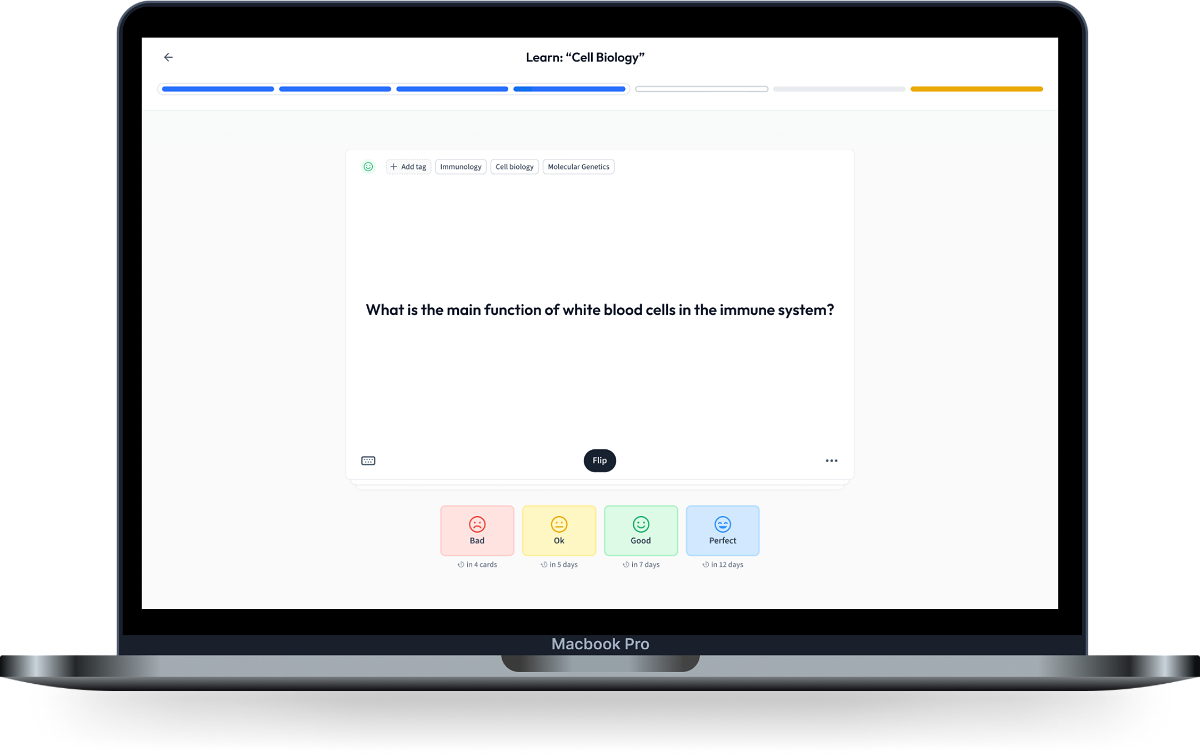
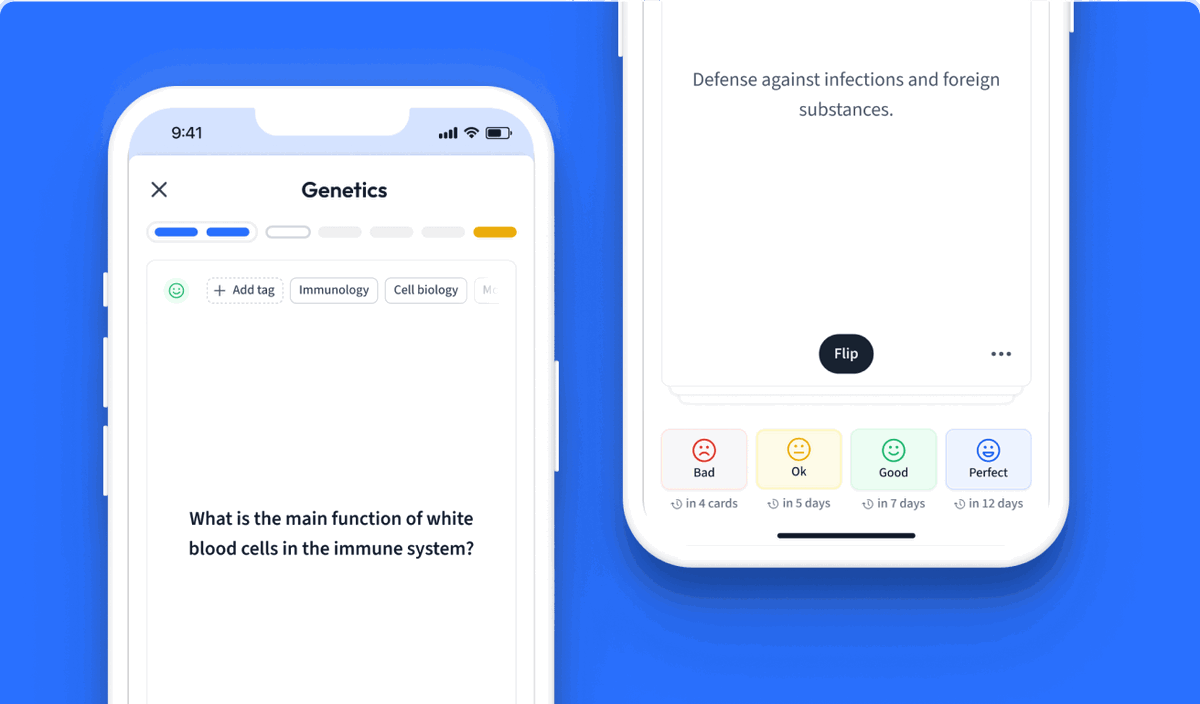
Learn with 12 p-value interpretation flashcards in the free StudySmarter app
We have 14,000 flashcards about Dynamic Landscapes.
Already have an account? Log in
Frequently Asked Questions about p-value interpretation
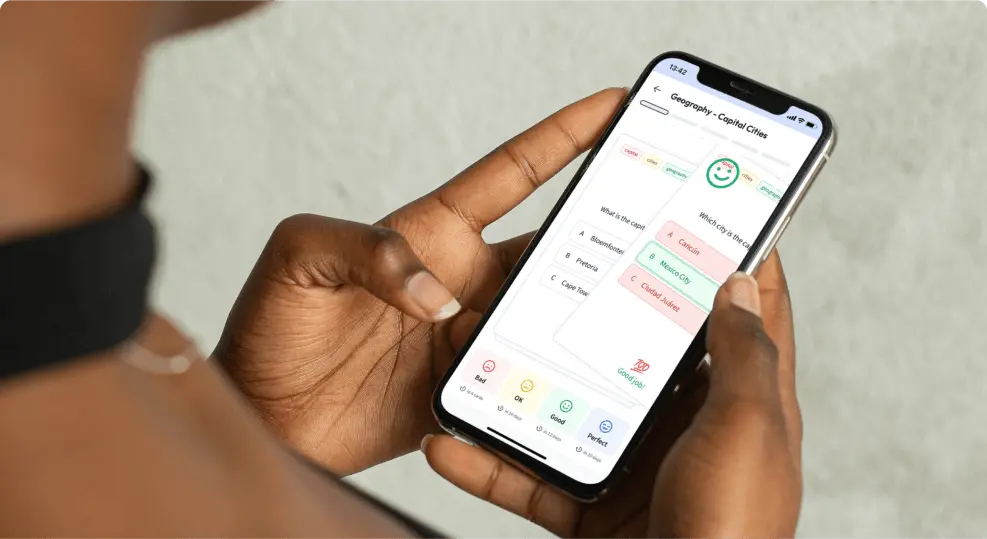
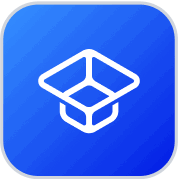
About StudySmarter
StudySmarter is a globally recognized educational technology company, offering a holistic learning platform designed for students of all ages and educational levels. Our platform provides learning support for a wide range of subjects, including STEM, Social Sciences, and Languages and also helps students to successfully master various tests and exams worldwide, such as GCSE, A Level, SAT, ACT, Abitur, and more. We offer an extensive library of learning materials, including interactive flashcards, comprehensive textbook solutions, and detailed explanations. The cutting-edge technology and tools we provide help students create their own learning materials. StudySmarter’s content is not only expert-verified but also regularly updated to ensure accuracy and relevance.
Learn more