Jump to a key chapter
Stratified Analysis Definition
Stratified Analysis is a statistical method used to control for confounding variables by analyzing subgroups within a dataset separately. This technique allows you to observe relationships within your data more accurately by ensuring that each subgroup is homogenous.
Purpose of Stratified Analysis
The purpose of stratified analysis is to enhance the clarity and accuracy of statistical interpretations by minimizing confounding biases. By breaking down your data into strata, you can examine the effects of a primary exposure, like a drug, accounting for different levels of other variables, such as age or gender. Regarding the mathematical foundation, if you think of a simple correlation coefficient formula \[r = \frac{n \sum xy - (\sum x)(\sum y)}{\sqrt{[n \sum x^{2} - (\sum x)^{2}][n \sum y^{2} - (\sum y)^{2}]}} \] controlling confounders helps ensure that the determined relationship is not falsely attributed due to undue influences.
Applications in Medicine
In medical research, stratified analysis is crucial, particularly in observational studies. It helps in understanding the impact of a treatment or intervention by controlling for variables like age, sex, and pre-existing conditions. For example, consider a clinical study assessing the effect of an asthma medication across various age groups. The analysis would be stratified by age to provide insights into differential effectiveness. This aids in making targeted medical recommendations. Additionally, stratified analysis can be used to understand incidences of certain conditions across different demographics. For instance, the prevalence of hypertension may be assessed separately for different age groups to form precise interventions, utilizing calculations like: \[\text{Odds Ratio (OR)} = \frac{\text{A/B}}{\text{C/D}} \] where 'A' and 'B' are the number of exposed cases and controls, and 'C' and 'D' are the number of unexposed cases and controls in strata.
Confounding Variable: A confounding variable is an outside influence that changes the effect of a dependent and independent variable. It can introduce bias, leading to erroneous results if not controlled.
Consider a study investigating the link between coffee consumption and heart disease. Age might act as a confounding variable. If older individuals consume more coffee and have a higher incidence of heart disease, you might incorrectly infer a direct relationship between coffee and heart disease. Stratifying the analysis by age allows for a clearer understanding of the true relationships.
Remember to ensure your data quantities are adequate in each stratum for a meaningful stratified analysis. Small sample sizes can lead to unreliable conclusions.
Stratified analysis is often paired with matching techniques in study designs to enhance reliability.
For computational analysis on large data sets, stratified random sampling can improve the precision of your estimates. This involves dividing your dataset into strata first and then taking a random sample from each group. In medicine, as previously mentioned with the coffee consumption example, pooling results from each stratum after individual analysis might involve applying multivariate statistical models. This technique is referred to as a Mantel-Haenszel procedure, allowing for adjusted estimations across various strata. The Mantel-Haenszel formula for odds ratios is: \[OR_{MH} = \frac{\sum{(ad/n)}}{\sum{(bc/n)}} \] where 'a' and 'b' refer to exposed and unexposed cases, respectively, and 'n' is the total number of participants within that stratum. Such techniques are foundational in epidemiological studies, helping validate findings by reducing bias.
Stratified Analysis in Epidemiology
Stratified Analysis in epidemiology is essential for understanding interactions in data by accounting for different categories separately. This minimization of confounding variables is crucial to ensure more accurate assessments of relationships within public health research.
Understanding Stratified Analysis
When you implement stratified analysis, you essentially separate the dataset into distinct layers or 'strata'. Each of these strata is homogenous concerning the variable of interest. By analyzing them independently, you can gain deeper insights into the data. Consider using the formula for weighted average to compute overall estimates across strata: \[ \text{Weighted Average} = \frac{\sum (W_i \times X_i)}{\sum W_i} \] where \(W_i\) is the weight of each stratum and \(X_i\) is the measurement within each stratum.
Confounding Variable: A confounding variable is an outside factor that potentially distorts the perceived relationship between an exposure and outcome. Controlling for it is vital in achieving unbiased results.
Real-World Applications
In the realm of medicine, stratified analysis is pivotal. Whether you are evaluating a new treatment or studying disease prevalence patterns, constructing well-defined strata according to demographics such as age or sex can illuminate critical findings. Let's consider an example examining the link between smoking and lung cancer across different age groups:
- Young adults (18-35)
- Middle-aged individuals (36-55)
- Older adults (56 and above)
In a study observing the efficacy of a COVID-19 vaccine, researchers might stratify participants based on age. Analyzing efficacy within these age groups helps determine if the vaccine performs differently for younger versus older demographics. Such targeted studies are depicted using the formula:\[ RR = \frac{a/(a+b)}{c/(c+d)} \]where \(a\) and \(b\) represent vaccinated individuals, and \(c\) and \(d\) represent unvaccinated, across different strata.
Ensure a sufficient sample size in each stratum; small sample sizes can lead to inconclusive results.
In stratified analysis, one advanced method is the Mantel-Haenszel test. This technique provides a summary odds ratio across various strata, accounting for potential confounders. The formula is:\[ OR_{MH} = \frac{\sum (a_i \times d_i / n_i)}{\sum (b_i \times c_i / n_i)} \]where \(a_i\), \(b_i\), \(c_i\), and \(d_i\) are table entries for cases and controls in each stratum, and \(n_i\) denotes the total number within each stratum. This method upholds accuracy by ensuring robust adjustments across stratified groups.
Stratified Analysis Technique
Stratified Analysis is a cornerstone in statistical examination, allowing you to dissect data by breaking it into multiple subgroups or strata, enabling the identification of underlying patterns by isolating potential confounding variables.
Key Principles of Stratified Analysis
By utilizing stratified analysis, you are ensuring more accurate insights, especially in datasets prone to bias. This method involves dividing data based on particular characteristics like age, gender, or any variable of interest. Once stratified, the analysis occurs within each subgroup, accounting for differences and controlling biases. You might consider utilizing the formula for calculating the weighted mean to combine results across strata: \[\bar{X}_w = \frac{\sum (w_i \times x_i)}{\sum w_i}\] where \(w_i\) represents the weight assigned to each stratum and \(x_i\) is the value within each stratum.
Confounding Variable: A confounding variable is an external influence that can skew the relationship being studied between the primary variables of interest, potentially leading to false conclusions if left uncontrolled.
Imagine a scenario where researchers are examining the effect of a new diabetes medication. To ensure the results are not biased due to differing exercise routines among participants, the study could stratify data by activity level groups:
- Low activity
- Moderate activity
- High activity
Applications in Medical Research
Stratified analysis is widely utilized in the medical field to refine research conclusions. By stratifying individuals by characteristics like smoking status or BMI, researchers gain precision in studies. For instance, in analyzing cardiovascular risk, potential participants can be divided based on their cholesterol levels. Calculating risks via odds ratios from stratified data is common, using:\[ OR = \frac{(a/b)}{(c/d)} \] where \(a\) and \(b\) are the odds of incidence with and without exposure in one stratum, and \(c\) and \(d\) in another.
It's essential to account for sample sizes within each stratum to ensure the reliability of your conclusions.
In more advanced analyses, one technique employed is the Mantel-Haenszel method, used for stratified data to obtain an average association measure across groups. Especially in case-control studies, its odds ratio calculation is: \[ OR_{MH} = \frac{\sum (a_i \cdot d_i/n_i)}{\sum (b_i \cdot c_i/n_i)} \]Here, \(a_i\), \(b_i\), \(c_i\), and \(d_i\) are counts specific to each stratum, and \(n_i\) is the total number within those strata. This method adjusts results to account for confounding influences robustly, providing nuanced insights.
Stratified Analysis and Confounding
Stratified Analysis is a powerful statistical technique that helps to control for confounding variables by examining detailed subgroups within larger datasets. By dividing data into strata (like age or gender groups), you can more accurately study the relationships between variables, minimizing bias from confounders.
Confounding Variable: An external factor that potentially distorts the true relationship between the variables being studied, leading to a misinterpretation if not adequately controlled or accounted for.
When conducting stratified analysis, always ensure that each stratum has a sufficient number of observations to maintain the integrity of statistical conclusions.
Stratified Analysis Importance
Stratified analysis is crucial in fields such as medicine and public health, where it helps to achieve accurate and unbiased interpretations of complex data. For instance, suppose you are assessing the effect of a new antihypertensive drug. By stratifying your participants by age groups, you can determine if the drug is equally effective across different age bands. Mathematically, this can be visualized through weighted averages when combining stratum-specific results: \[ \bar{X}_w = \frac{\sum (w_i \times x_i)}{\sum w_i} \] where \(w_i\) represents the weight of each stratum, and \(x_i\) is the measurement for each stratum. This helps adjust for any unequal distribution of confounding variables across your groups.
For a more technical perspective, stratified analysis can also employ the Mantel-Haenszel test, which provides a pooled measure of association across all strata, often used in epidemiological studies to adjust for confounders. The calculation of the Mantel-Haenszel odds ratio is given by: \[ OR_{MH} = \frac{\sum (a_i \times d_i / n_i)}{\sum (b_i \times c_i / n_i)} \] In this equation, \(a_i\), \(b_i\), \(c_i\), \(d_i\) are the entries of contingency tables, specifically cases and controls across different strata, while \(n_i\) is the total within each stratum. This provides a comprehensive estimation that accounts for inter-stratum variations.
Stratified Analysis Example
Consider a situation where researchers aim to explore the impact of exercise on quality of life among diabetic patients. They might stratify patients into categories based on their BMI:
- Underweight group
- Normal weight group
- Overweight group
- Obese group
In analyzing the effectiveness of a vaccine, researchers may stratify the population by medical history to differentiate how those with pre-existing conditions respond compared to healthy counterparts. By doing so, they enhance the understanding of vaccine efficacy across diverse population subsets, effectively reducing confounding influences.
stratified analysis - Key takeaways
- Stratified Analysis Definition: A statistical method to control for confounding variables by analyzing separate subgroups within a dataset, ensuring each subgroup is homogenous.
- Importance in Epidemiology: Helps in understanding data interactions by accounting for different categories, minimizing confounding variables to offer more accurate public health research assessments.
- Stratified Analysis Technique: Divides data into multiple subgroups, allowing identification of underlying patterns by isolating potential confounding variables.
- Confounding Role: Stratified analysis minimizes bias by controlling confounders, which distort the perceived relationship between exposure and outcome.
- Real-World Example: In evaluating vaccine efficacy, stratifying participants by age determines if the vaccine performs differently across demographics, highlighting its differential impact.
- Mantel-Haenszel Technique: A method in stratified analysis to calculate a pooled measure of association, adjusting for confounders, especially used in epidemiological studies.
Learn faster with the 12 flashcards about stratified analysis
Sign up for free to gain access to all our flashcards.
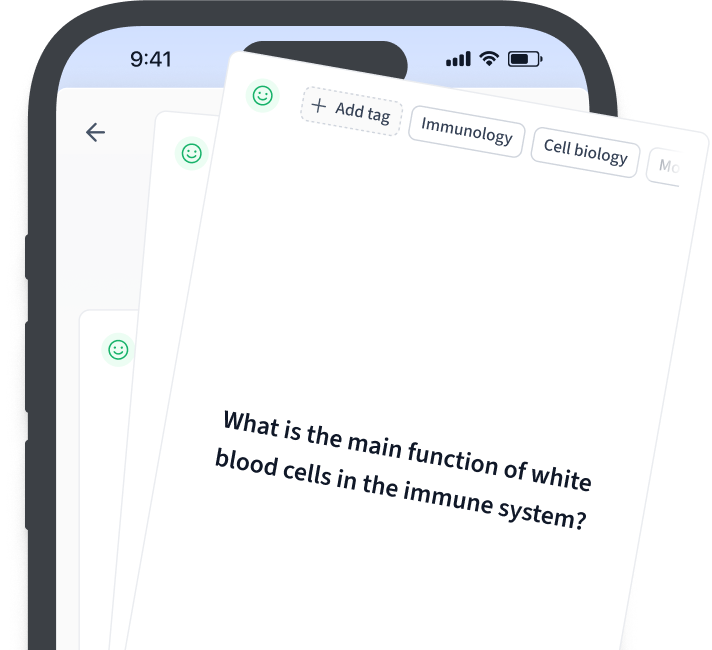
Frequently Asked Questions about stratified analysis
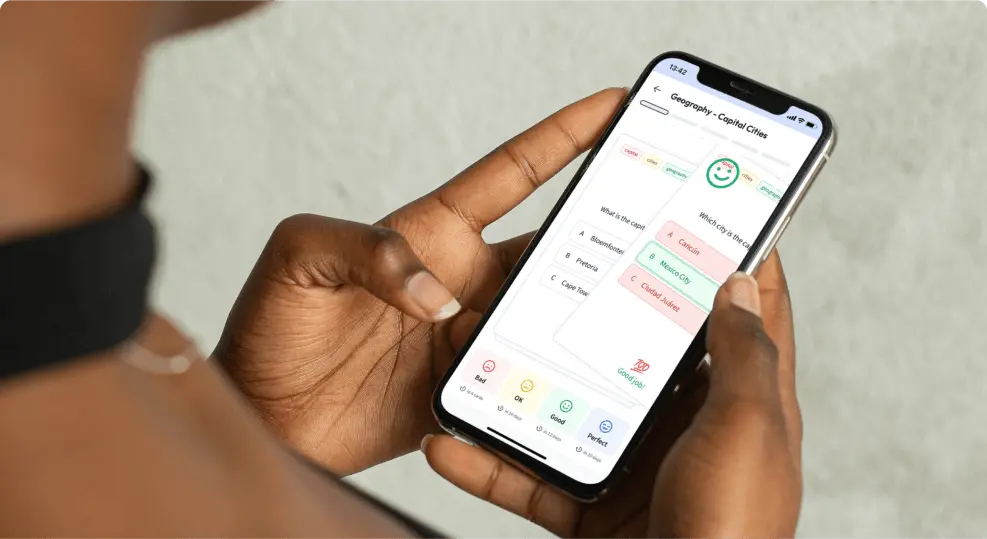
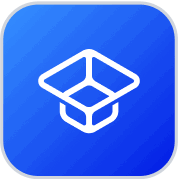
About StudySmarter
StudySmarter is a globally recognized educational technology company, offering a holistic learning platform designed for students of all ages and educational levels. Our platform provides learning support for a wide range of subjects, including STEM, Social Sciences, and Languages and also helps students to successfully master various tests and exams worldwide, such as GCSE, A Level, SAT, ACT, Abitur, and more. We offer an extensive library of learning materials, including interactive flashcards, comprehensive textbook solutions, and detailed explanations. The cutting-edge technology and tools we provide help students create their own learning materials. StudySmarter’s content is not only expert-verified but also regularly updated to ensure accuracy and relevance.
Learn more