Jump to a key chapter
Nonparametric Statistics in Psychology
In the field of psychology, nonparametric statistics offer valuable tools when it comes to analyzing data that does not necessarily meet the assumptions of parametric tests. These tests are particularly useful when dealing with ordinal data or when the sample size is too small to justify assumptions about the underlying population distribution.
Understanding Nonparametric Statistics
Nonparametric statistics do not require assumptions about the specific distribution of the data. Instead, they rely on fewer assumptions, making them applicable in situations where parametric tests, like the t-test or ANOVA, are not suitable. These tests are useful when the focus is on ranks rather than numerical values. Some common nonparametric tests include:
- The Wilcoxon Signed-Rank Test, used as an alternative to the paired t-test.
- The Mann-Whitney U Test, serving as a substitute for the independent samples t-test.
- The Kruskal-Wallis Test, employed instead of a one-way ANOVA.
Nonparametric Statistics: A branch of statistics that does not assume a fixed structure or distribution for the data, especially useful when data is ordinal or the sample size is small.
Consider a psychologist studying the effects of a new therapy on anxiety levels. The therapist collects anxiety scores from a small group of participants before and after the therapy. Due to the small sample size and ordinal nature of these scores, a nonparametric test like the Wilcoxon Signed-Rank Test would be suitable for analyzing the data.
Application of Nonparametric Statistics
In psychological research, nonparametric tests are often used to supplement findings when data does not meet the assumptions necessary for parametric approaches. For instance, when researchers are dealing with data that involves rankings, such as preference scales or Likert-type items, these tests offer an effective method for analysis. Let's explore a typical formula used in nonparametric statistics - the calculation of the mean rank for the Mann-Whitney U Test. Suppose you want to compare two independent groups using their rank sums. If each group has the following rank sums:
- Group A: 36
- Group B: 28
Nonparametric statistics provide a profound advantage in exploratory research where little is known about the population or where assumptions cannot be met. Many psychological phenomena are not normally distributed or are measured in ways that do not meet parametric test requirements. For example, psychological experiments often involve subjective measures, such as happiness or satisfaction levels, which are ordinal rather than interval or ratio in nature. Another advantage is that many nonparametric tests have a reduced Type I error rate in the presence of outliers or skewed data. This makes them robust choices when data quality is a concern.Moreover, the advent of computing power has made it easier to use resampling techniques, such as bootstrapping or permutation tests, to conduct nonparametric tests over large datasets, thus improving the reliability of psychological research using nonparametric methods.
Examples of Nonparametric Statistics in Psychology
Nonparametric statistics often come to the rescue in psychological studies, especially when assumptions of normality are violated or when dealing with small sample sizes. They provide robust analytical tools that help in making meaningful inferences.
Wilcoxon Signed-Rank Test Example
The Wilcoxon Signed-Rank Test is a powerful alternative to the paired t-test, particularly when the data does not follow a normal distribution. It's often used in psychology when examining the differences between matched pairs or repeated measures.
Imagine a psychologist evaluating the impact of a mindfulness program. Pre and post-test anxiety scores of participants can be analyzed using the Wilcoxon Signed-Rank Test to determine if the program has led to a significant decrease in anxiety levels.
Mann-Whitney U Test for Independent Samples
Another common nonparametric test is the Mann-Whitney U Test, which is used when comparing differences between two independent groups. This test ranks all the data points in both groups combined and then compares the sum of ranks.
Suppose you are comparing satisfaction scores from two different counseling methods. You can apply the Mann-Whitney U Test to evaluate if there is a significant difference in satisfaction between the two groups.
Mann-Whitney U Test: A nonparametric test for assessing whether two independent samples come from the same distribution.
Kruskal-Wallis Test for More than Two Groups
The Kruskal-Wallis Test extends the Mann-Whitney U Test to more than two groups. It is an ideal choice for comparing medians across multiple groups in a psychological study where data assumptions are not met.
Consider a study evaluating the effectiveness of three different therapy techniques on reducing stress. The Kruskal-Wallis Test can be employed to check if there are statistically significant differences in the stress levels across the three groups.
An advantage of nonparametric tests is their versatility in dealing with various data types that are common in psychology, such as ordinal data from Likert scales or rankings. They also provide a safeguard against the misleading results that could arise from outlier data. One intriguing aspect of nonparametric methods includes their use in robust statistical modeling and analysis. For example, in robust regression, nonparametric techniques can be utilized to generate confidence intervals without assuming the underlying data distribution. These capabilities make them indispensable in handling diverse data challenges in psychological research.
Nonparametric tests generally have less statistical power than parametric ones, particularly with small sample sizes. However, this trade-off is often worthwhile in real-world psychological studies where assumptions of parametric tests are not met.
Nonparametric Techniques in Psychology
Nonparametric statistical techniques are pivotal in psychological research, particularly when data do not conform to parametric test assumptions. These methods provide greater flexibility, especially for analyzing ordinal data or data from smaller sample sizes.As you explore nonparametric statistics, consider how they allow you to focus on the ranks and medians of your data, rather than strict numerical values. This approach can be greatly beneficial in psychology, providing insights that might be obscured by traditional methods.
Examples of Nonparametric Tests
Here are some common nonparametric tests used in psychological analysis:
- Wilcoxon Signed-Rank Test: Ideal for matched pairs or repeated measures.
- Mann-Whitney U Test: Used to compare two independent groups.
- Kruskal-Wallis Test: Suitable for comparing more than two groups.
To better understand these tests, consider you are a psychologist examining the effect of three different learning methods on student performance. Suppose you have ordinal data from a small, non-normally distributed sample. The Kruskal-Wallis Test would be appropriate here to determine if there are significant differences in performance across the methods.
Understanding Nonparametric Tests
When employing nonparametric tests, it is crucial to comprehend their workings. These tests often employ ranks rather than actual data values, which circumvents the need for normal distribution. For example, in a Mann-Whitney U Test, data from two groups are combined and ranked. The U statistic can be calculated using the formula:\[ U = n_1 \cdot n_2 + \frac{{n_1(n_1 + 1)}}{2} - R_1 \]where:
- \( n_1 \) is the size of the first sample
- \( n_2 \) is the size of the second sample
- \( R_1 \) is the sum of ranks for the first sample
Parametric Statistics vs Nonparametric Statistics
Parametric statistics and nonparametric statistics represent two broad categories of statistical methods used in data analysis. The key difference lies in the assumptions made about the data's distribution. Parametric statistics require that the data meet certain assumptions, such as normal distribution, while nonparametric statistics do not. This makes nonparametric tests more flexible and suited for a wider range of data types, especially when data are ordinal or do not meet parametric assumptions.
Parametric Statistics: Statistical methods that assume the data follows a known distribution, typically a normal distribution.
Parametric and Nonparametric Statistical Tests
In statistical analysis, choosing between parametric and nonparametric tests often depends on the data characteristics and research questions. Here’s a comparison:
Parametric Tests | Nonparametric Tests |
T-tests, ANOVA, regression | Wilcoxon Signed-Rank Test, Mann-Whitney U Test, Kruskal-Wallis Test |
Assumes normal distribution | No specific distribution assumptions |
Higher statistical power when assumptions are met | More robust to violations of assumptions |
- Use parametric tests when data are interval or ratio and assumptions can be met.
- Opt for nonparametric tests with ordinal data or when normality cannot be assumed.
Always verify if your data meets the necessary assumptions for parametric tests to avoid unreliable results.
Types of Statistics Nonparametric Tests
Nonparametric tests are diverse and cater to various data and research requirements. Some common ones include:
- Wilcoxon Signed-Rank Test: Used for matched pairs or repeated measures.
- Mann-Whitney U Test: Tests differences between two independent groups.
- Kruskal-Wallis Test: Compares more than two independent groups.
If you're evaluating the efficacy of two different teaching methods using student scores, where scores are not normally distributed, the Mann-Whitney U Test may be ideal. The formula for calculating the U statistic is: \( U = n_1 \cdot n_2 + \frac{{n_1(n_1 + 1)}}{2} - R_1 \)where \(n_1\) and \(n_2\) are the sample sizes, and \(R_1\) is the sum of ranks for the first group.
Nonparametric tests shine in flexibility and applicability. In psychological studies, where data might be skewed or ordinal, nonparametric tests offer reliable alternatives. Consider the greater robustness these tests provide against non-normal data distributions.In a nonparametric context, resampling methods like bootstrapping further enhance analysis by generating sampling distributions through repeated sampling with replacement. This approach allows for more accurate estimation of population parameters and is particularly useful with smaller samples or in exploratory studies. With the advancement of computing, computationally intensive techniques like bootstrapping and permutation tests have become accessible, providing psychologists with robust tools to handle complex data challenges effectively.
Nonparametric Statistics Tests Guide
Navigating nonparametric tests requires understanding when and how to apply them. Here’s a quick guide:
- Identify Your Data Level: Check if data are nominal, ordinal, interval, or ratio.
- Check Assumptions: Ensure normality checks or data characteristics to determine suitability.
- Choose the Right Test: Match data characteristics with the appropriate test (e.g., choose Kruskal-Wallis for multiple group comparisons).
nonparametric statistics - Key takeaways
- Nonparametric Statistics: A branch of statistics that does not assume data distribution, ideal for ordinal data or small sample sizes. It uses fewer assumptions than parametric statistics.
- Nonparametric techniques in psychology: Include tests like the Wilcoxon Signed-Rank Test, Mann-Whitney U Test, and Kruskal-Wallis Test; useful for ordinal data or non-normal distributions in psychological studies.
- Examples of nonparametric statistics in psychology: Wilcoxon Signed-Rank Test for therapy outcomes on anxiety, Mann-Whitney U Test for different counseling methods, Kruskal-Wallis Test for multiple therapy techniques.
- Parametric Statistics vs Nonparametric Statistics: Parametric statistics assume normal distribution, while nonparametric do not, making the latter more flexible for non-normal data.
- Parametric and nonparametric statistical tests: Parametric tests include t-tests and ANOVA; nonparametric alternatives are Wilcoxon Signed-Rank, Mann-Whitney U, and Kruskal-Wallis tests.
- Statistics nonparametric tests: Used when data does not meet parametric assumptions, offering robust analysis for diverse data types common in psychology.
Learn faster with the 12 flashcards about nonparametric statistics
Sign up for free to gain access to all our flashcards.
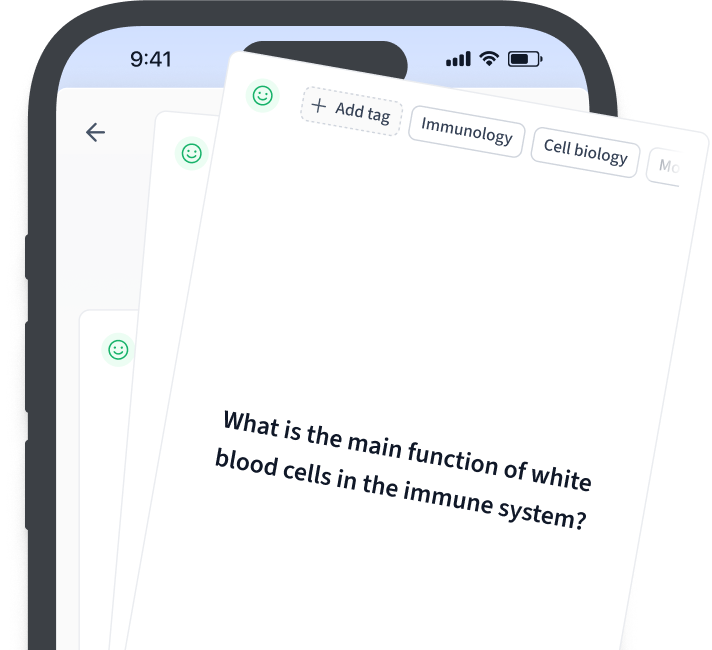
Frequently Asked Questions about nonparametric statistics
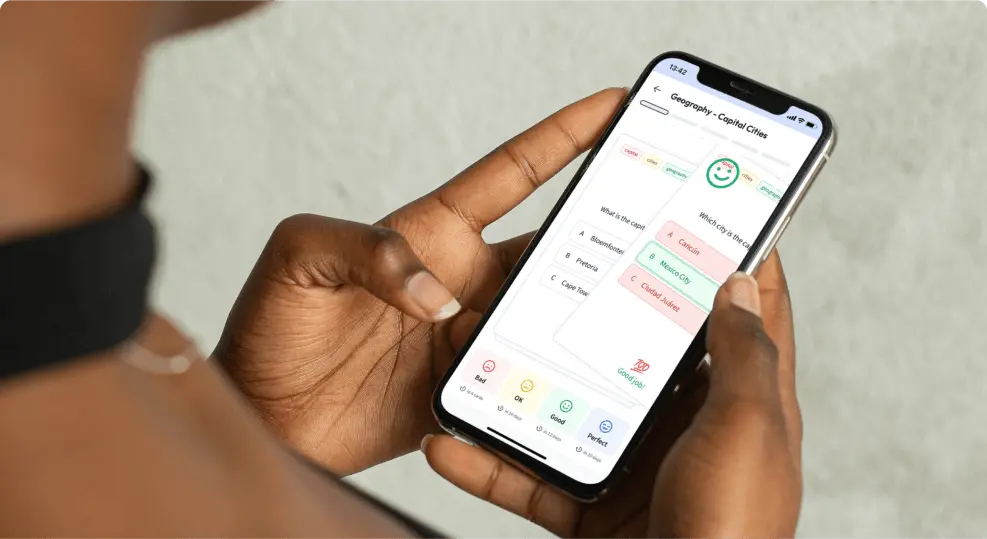
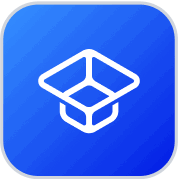
About StudySmarter
StudySmarter is a globally recognized educational technology company, offering a holistic learning platform designed for students of all ages and educational levels. Our platform provides learning support for a wide range of subjects, including STEM, Social Sciences, and Languages and also helps students to successfully master various tests and exams worldwide, such as GCSE, A Level, SAT, ACT, Abitur, and more. We offer an extensive library of learning materials, including interactive flashcards, comprehensive textbook solutions, and detailed explanations. The cutting-edge technology and tools we provide help students create their own learning materials. StudySmarter’s content is not only expert-verified but also regularly updated to ensure accuracy and relevance.
Learn more