Jump to a key chapter
Definition of Training Load Monitoring in Medicine
Training Load Monitoring is a crucial concept in sports science and medicine that involves the systematic tracking and analysis of the amount, frequency, and intensity of exercise an individual undergoes. It serves to ensure the safety, effectiveness, and optimization of athletic performance and recovery.
Purpose of Training Load Monitoring
The primary aim of training load monitoring is to balance workload and recovery, reducing the risk of injuries and enhancing performance. By understanding your current training levels, you can make informed decisions to:
- Optimize performance
- Prevent overtraining injuries
- Plan recovery effectively
- Assess adaptation to training
Methods of Monitoring Training Load
Training load can be monitored using different methodologies, which may include both internal and external load measures.Internal Load: This refers to the physiological or psychological responses during training, offering insights into how the body reacts to exercise. Important metrics are:
- Heart rate
- Perceived exertion rate
- Blood lactate concentration
- Distance covered
- Power output
- Speed
Example: An athlete uses a GPS device and a heart rate monitor during training to track distance and heart rate, integrating both external and internal load data for comprehensive monitoring.
Advanced applications exist beyond basic monitoring, where systems use sophisticated algorithms to analyze data trends over weeks and months. This is crucial in settings like professional sports, where data-driven decisions can lead to substantial improvements in athletic performance while minimizing injury risk. These systems can automatically alert you about potential overtraining or provide insights into the necessary adjustments required for optimal performance. Employing machine learning, some systems can even predict future performance outcomes based on current training regimens.
Challenges in Training Load Monitoring
Despite the benefits, there are challenges in training load monitoring. Key issues include:
- Data overload: The sheer amount of data can be overwhelming and misinterpreted.
- Technological limitations: Not every tool may accurately capture all aspects of load.
- Individual differences: Personalized responses to training may require tailored monitoring protocols.
- User compliance: Ensuring consistent and correct data input by athletes.
Training Load Monitoring Techniques in Sports Medicine
In sports medicine, monitoring training load is essential to enhance performance and prevent injuries. It integrates various techniques to measure and analyze the exertion and recovery of athletes.
Different Methods for Training Load Monitoring
Internal Load: Refers to the physiological and psychological responses of the body to external loads, measurable through indicators like heart rate, perceived exertion, and hormonal changes.
External Load involves objective measurements of the work done during training, such as:
- Distance covered
- Speed
- Power output
Example: An athlete wearing a heart rate monitor and a GPS tracker during a 5 km run might observe:
- Average heart rate: 150 bpm
- Distance: 5 km at 5 min per km pace
- Calories burned: Based on heart rate data
Consistency in using the same monitoring tools enhances the reliability of the collected data.
Technological Advances in Monitoring
Advancements in technology have significantly improved training load monitoring. For instance, wearable devices now offer:
- Real-time feedback
- Long battery life
- Wireless data transfer
- Enhanced metric accuracy
Considering advanced technology applications in sports science, data analytics can identify potential risks of overtraining by detecting anomalies in performance patterns. For example, if an athlete's heart rate recovery post-training session falls outside expected limits using the formula: \[ HRR = HR_{end} - HR_{after 2 min} \] where HRR is heart rate recovery, it might indicate insufficient recovery or underlying health issues. Data integration across numerous sessions allows for a holistic view, enhancing decision-making in training adjustments and health monitoring. Predictive models integrate multiple variables to identify potential injurious patterns before symptoms arise, optimizing overall load management.
Understanding Training Load Monitoring for Injury Prevention
Training Load Monitoring plays a pivotal role in preventing injuries, ensuring athletes maintain an optimal balance between training intensity and recovery. By regulating training loads, you can reduce the risk of overuse injuries.
Key Components of Training Load Monitoring
Training load monitoring entails various components important for maintaining athlete health.Here are key components:
- Internal Load: Physiological metrics like heart rate and perceived exertion
- External Load: Quantitative measures such as distance and speed
- Recovery Assessment: Monitoring rest and recuperation times
Internal Load measures how the body responds to training stimuli, providing insights into physical conditioning.
Example: Monitoring heart rate during a workout for an athlete might show different levels of exertion when covering the same distance on different days, allowing adjustments in training loads.
Consider using a combination of subjective methods like perceived exertion and objective methods like wearable technology for comprehensive monitoring.
Formulas and Calculations in Training Load Monitoring
Various formulas can help in calculating and quantifying training load. Two common formula types include calculation of work done and energy expenditure.An example formula for work done is:\[ Work = Force \times Distance \]Energy expenditure during training can be calculated using:\[ Energy \, Expenditure = METs \times Weight \times Duration \]Where METs signifies the Metabolic Equivalent Task, a unit that estimates energy cost.
Expanding on quantitative analyses, algorithms in software are designed to calculate more complex metrics like the Training Stress Score (TSS), which estimates the stress of a workout based on intensity and duration. The formula is shown as:\[ TSS = \frac{Training \, Intensity \, Factor^2 \, \times \, Duration}{Maximum \, Performance} \]This formula integrates factors such as VO2 max and lactate threshold parameters, offering deeper insights into the stress levels imposed on the body. It encompasses elements beyond basic calculations, helping in long-term injury prevention by optimizing training plans to individual athlete profiles.
Monitoring Training Load to Understand Fatigue in Athletes
In sports science, monitoring training load is instrumental in understanding and managing athlete fatigue. Accurately tracking and analyzing training load can help in optimal performance and risk management.
Training Load Monitoring Explained
Training load monitoring involves measuring the intensity and volume of training to understand its impact on an athlete's body. It helps track:
Various methods, both subjective and objective, such as heart rate monitors, wearables, and self-assessment scales are used to gather comprehensive data.Training Load: A measure combining both intensity and duration of exercise, reflecting the total stress placed on an athlete.
Example: For calculating training load, you might log a running session with 30 minutes at a moderate pace and 30 minutes at a high intensity. The combined effect gives a comprehensive measure of the session's load.
Monitoring fatigue can enhance recovery strategies and inform return-to-training plans after an injury.
Taking a deeper dive into technology, advanced algorithms are using machine learning to predict potential performance outcomes based on training loads. These systems analyze trends over time, providing personalized recommendations for athletes, such as suggesting adjustments in intensity or duration before signs of fatigue appear. This predictive approach is becoming increasingly reliable in professional sports settings, where optimizing performance and minimizing risk are priorities.
Applications of Training Load Monitoring in Physical Therapy
In physical therapy, training load monitoring can be pivotal for rehabilitation. It ensures safe progress through:
- Customized rehabilitation plans
- Monitoring responses to therapy
- Adjusting exercise loads
- Preventing re-injury
Example: In a case of ligament injury recovery, tracking the load during slow-paced treadmill walking sessions helps therapists gauge when the patient can progress to more challenging activities, like light jogging.
A deep exploration into the integration of training load monitoring and physical therapy reveals that data from sophisticated tools helps refine injury recovery protocols. For example, therapists might use differential equations to model muscle recovery dynamics, ensuring therapies are set at an appropriate workload for promoting healing without overstressing injured tissues. By overlaying physical therapy progress with real-time metrics, therapists can personalize interventions, respond dynamically to setbacks or rapid recoveries, and ensure physical demands correlate with clinical objectives.
training load monitoring - Key takeaways
- Definition of Training Load Monitoring in Medicine: Involves tracking and analyzing exercise quantities for safety, performance optimization, and injury prevention.
- Purpose: Balancing workload and recovery is essential to reduce injury risk, optimize performance, plan recovery, and assess training adaptation.
- Methods: Involve internal measures (heart rate, perceived exertion) and external measures (distance, power) using both subjective assessments and technological tools.
- Techniques in Sports Medicine: Essential for performance enhancement and injury prevention through comprehensive data analysis.
- Understanding Monitoring for Injury Prevention: Regulates training loads to maintain balance between exercise intensity and recovery.
- Applications in Physical Therapy: Used for rehabilitation planning, therapy response monitoring, load adjustments, and re-injury prevention.
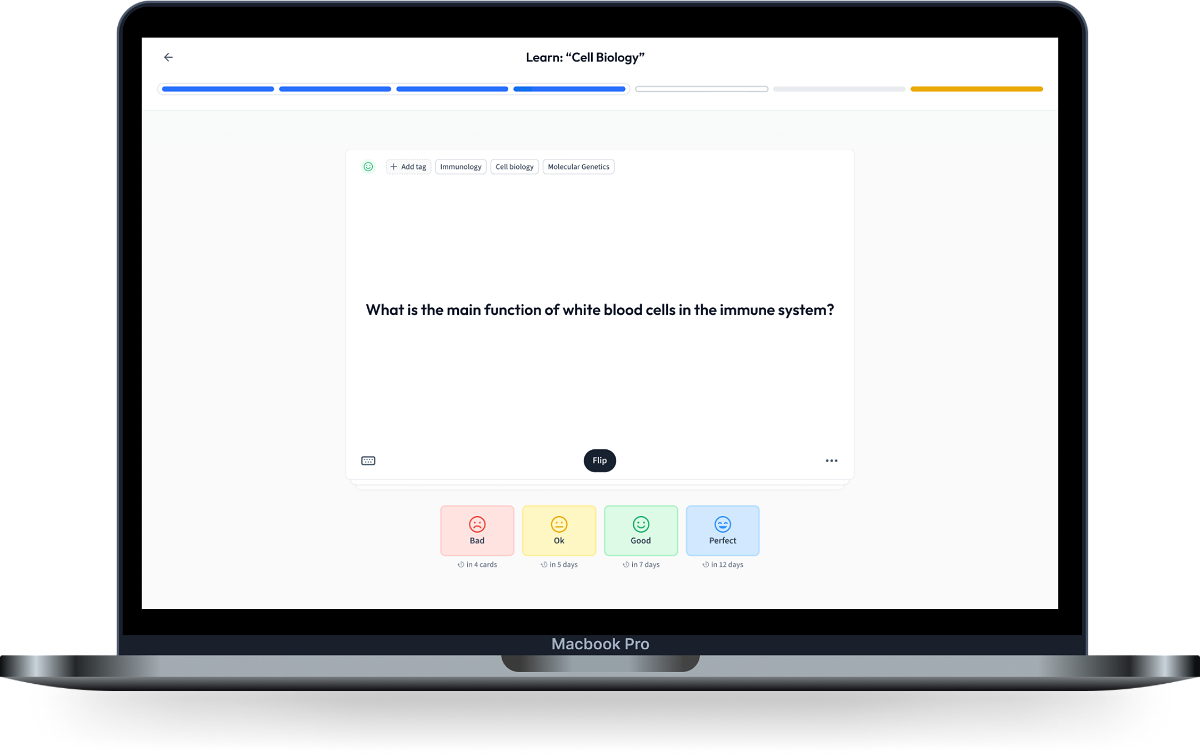
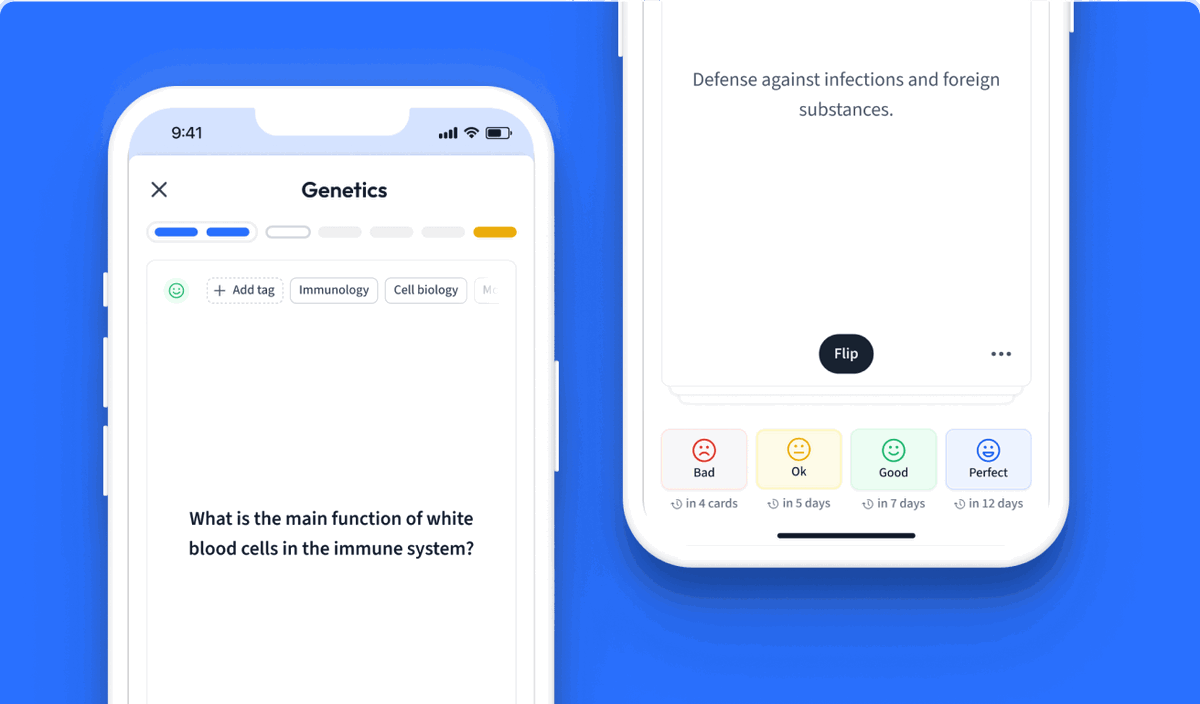
Learn with 12 training load monitoring flashcards in the free StudySmarter app
We have 14,000 flashcards about Dynamic Landscapes.
Already have an account? Log in
Frequently Asked Questions about training load monitoring
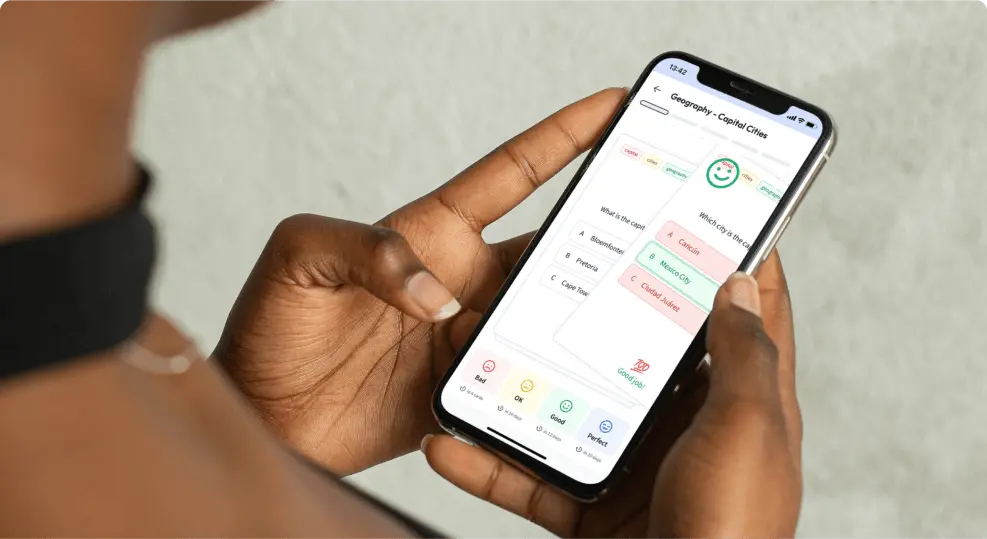
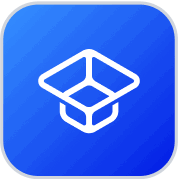
About StudySmarter
StudySmarter is a globally recognized educational technology company, offering a holistic learning platform designed for students of all ages and educational levels. Our platform provides learning support for a wide range of subjects, including STEM, Social Sciences, and Languages and also helps students to successfully master various tests and exams worldwide, such as GCSE, A Level, SAT, ACT, Abitur, and more. We offer an extensive library of learning materials, including interactive flashcards, comprehensive textbook solutions, and detailed explanations. The cutting-edge technology and tools we provide help students create their own learning materials. StudySmarter’s content is not only expert-verified but also regularly updated to ensure accuracy and relevance.
Learn more